Locally Weighted Full Covariance Gaussian Density Estimation
msra(2004)
摘要
We describe an interesting application of the principle of local learning to density estimation. Locally weighted fitting of a Gaussian with a regularized full covariance matrix yields a density estimator which displays improved behavior in the case where much of the probability mass is concentrated along a low dimensional manifold. While the proposed estimator is not guaranteed to integrate to 1 with a finite sample size, we prove asymptotic convergence to the true density. Experimental results illustrating the advan- tages of this estimator over classic non-parametric estimators are presented.
更多查看译文
关键词
sample size,parametric model,density estimation,covariance matrix
AI 理解论文
溯源树
样例
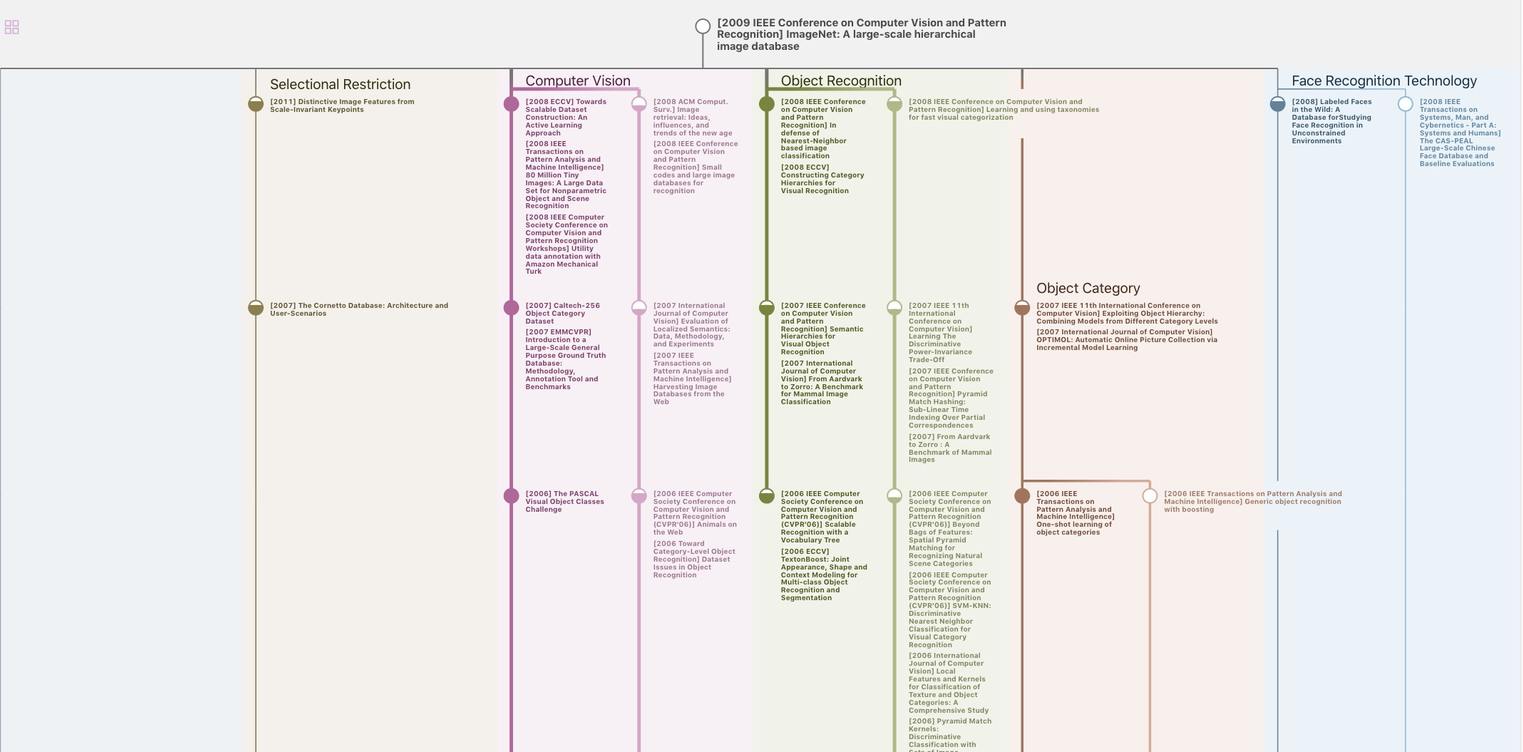
生成溯源树,研究论文发展脉络
Chat Paper
正在生成论文摘要