Knowledge-based approach for functional MRI analysis by SOM neural network using prior labels from Talairach stereotaxic space
Proceedings of SPIE(2002)
摘要
Among the methods proposed for the analysis of functional MR we have previously introduced a model-independent analysis based on the self-organizing map (SOM) neural network technique. The SOM neural network can be trained to identify the temporal patterns in voxel time-series of individual functional MRI (fMRI) experiments. The separated classes consist of activation, deactivation and baseline patterns corresponding to the task-paradigm. While the classification capability of the SOM is not only based on the distinctness of the patterns themselves but also on their frequency of occurrence in the training set, a weighting or selection of voxels of interest should be considered prior to the, training of the neural network to improve pattern learning. Weighting of interesting voxels by means of autocorrelation or F-test significance levels has been used successfully, but still a large number of baseline voxels is included in the training. The purpose of this approach is to avoid the inclusion of these voxels by using three different levels of segmentation and mapping from Talairach space: (1) voxel partitions at the lobe level, (2) voxel partitions at the gyrus level and (3) voxel partitions at the cell level (Brodmann areas). The results of the SOM classification based on these mapping levels in comparison to training with all brain voxels are presented in this paper.
更多查看译文
关键词
fMRI,non-linear regression,self-organizing map (SOM),time series analysis,un-supervised classification,Talairach stereotaxic space
AI 理解论文
溯源树
样例
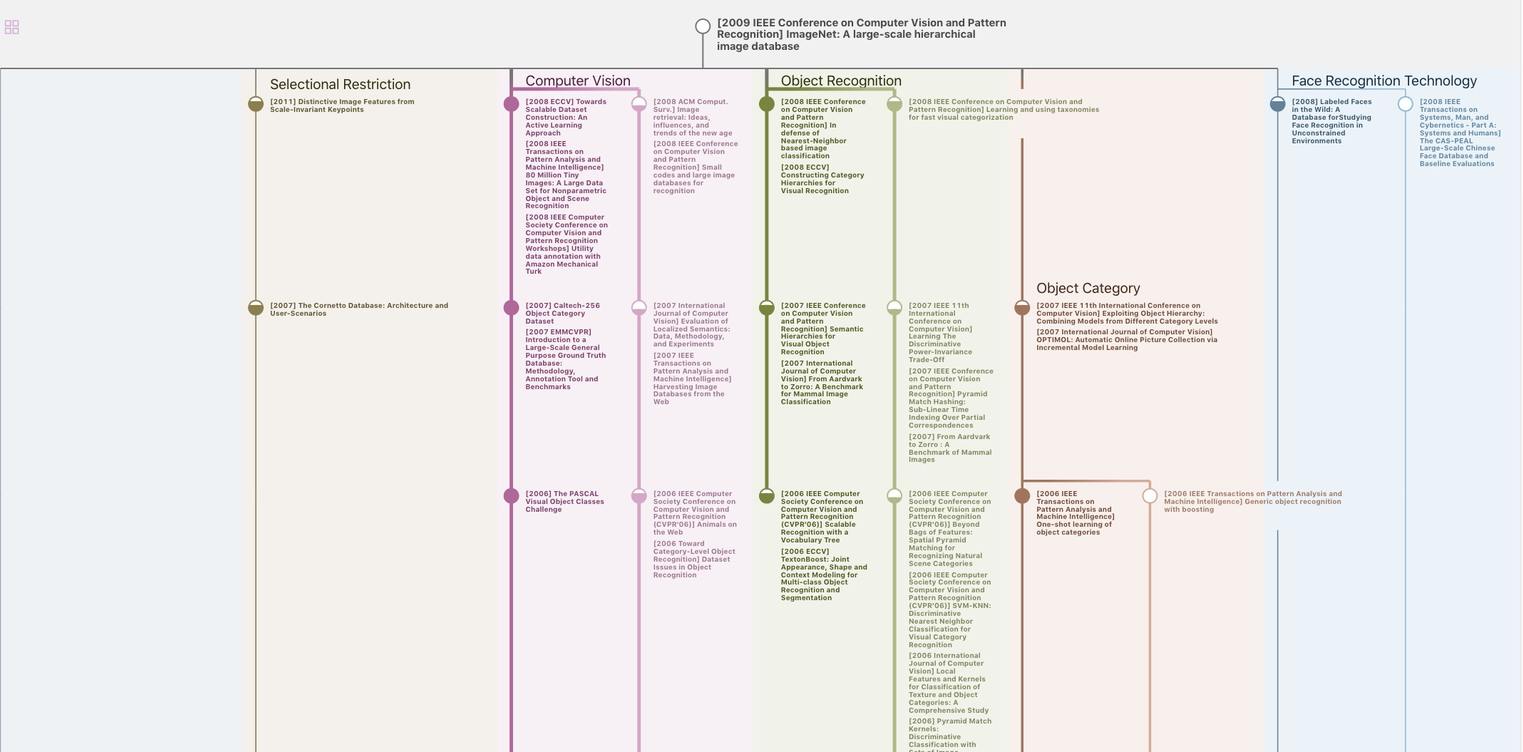
生成溯源树,研究论文发展脉络
Chat Paper
正在生成论文摘要