Expectation propagation as a way of life
mag(2014)
摘要
We revisit expectation propagation (EP) as a prototype for scalable algorithms that partition big datasets into many parts and analyze each part in parallel to perform inference of shared parameters. The algorithm should be particularly efficient for hierarchical models, for which the EP algorithm works on the shared parameters (hyperparameters) of the model. The central idea of EP is to work at each step with a "tilted distribution" that combines the likelihood for a part of the data with the "cavity distribution," which is the approximate model for the prior and all other parts of the data. EP iteratively approximates the moments of the tilted distributions and incorporates those approximations into a global posterior approximation. As such, EP can be used to divide the computation for large models into manageable sizes. The computation for each partition can be made parallel with occasional exchanging of information between processes through the global posterior approximation. Moments of multivariate tilted distributions can be approximated in various ways, including, MCMC, Laplace approximations, and importance sampling.
更多查看译文
关键词
propagation,life
AI 理解论文
溯源树
样例
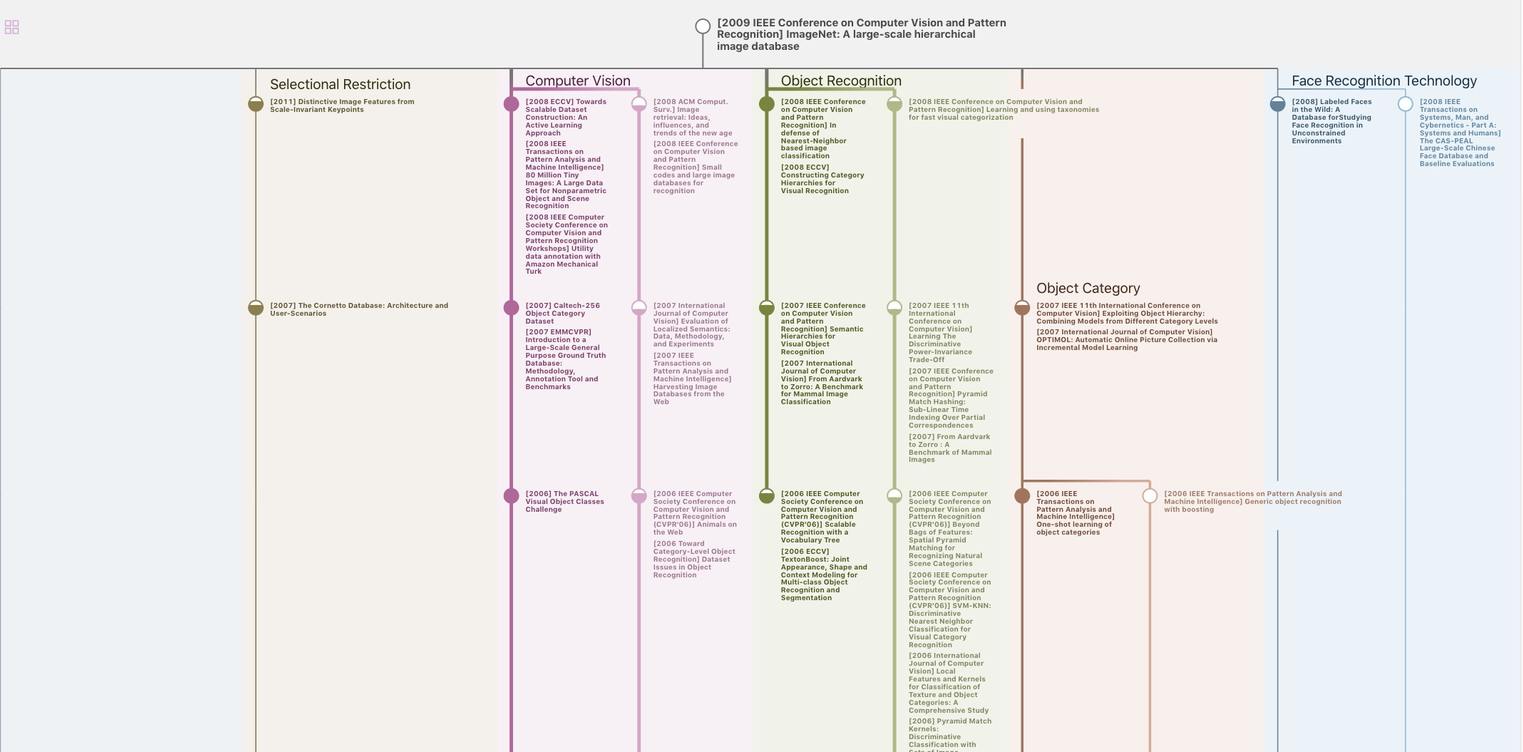
生成溯源树,研究论文发展脉络
Chat Paper
正在生成论文摘要