Receding horizon controller using particle swarm optimization for closed-loop ground target surveillance and tracking
Proceedings of SPIE(2009)
摘要
This paper investigates the problem of non-myopic multiple platform trajectory control in a multiple target search and
track setting. It presents a centralized receding discrete time horizon controller (RHC) with variable-step look-ahead for
motion planning of a heterogeneous ensemble of airborne sensor platforms. The controller operates in a closed feedback
loop with a Multiple Hypothesis Tracker (MHT) that fuses the disparate sensor data to produce target declarations and
state estimates. The RHC action space for each air vehicle is represented via maneuver automaton with simple motion
primitives. The reward function is based on expected Fisher information gain and priority scaling of target tracks and
ground regions. A customized Particle Swarm Optimizer (PSO) is developed to handle the resulting non-Markovian,
time-varying, multi-modal, and discontinuous reward function. The algorithms were evaluated by simulating ground
surveillance scenarios using representative sensors with varying fields of view and typical target densities and motion
profiles. Simulation results show improved aggregate target detection, track accuracy, and track maintenance for closed-loop
operation as compared with typical open-loop surveillance plans.
更多查看译文
关键词
look ahead,sensors,algorithms,motion planning,feedback loop,field of view,feedback loops,particle swarm optimization,particles,fisher information,discrete time
AI 理解论文
溯源树
样例
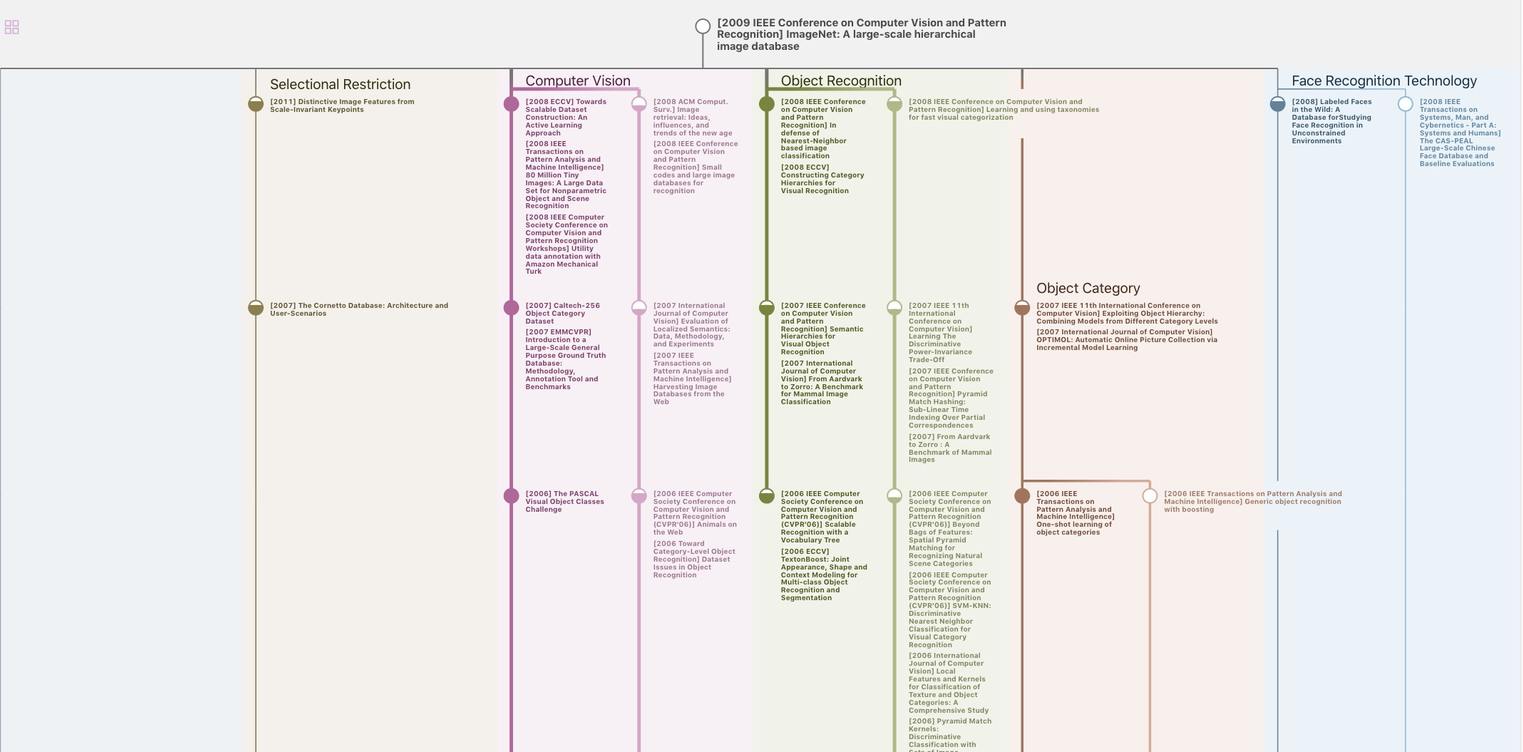
生成溯源树,研究论文发展脉络
Chat Paper
正在生成论文摘要