A Swarm-Based Approach To Learning Phase-Type Distributions For Continuous Time Bayesian Networks
2015 IEEE SYMPOSIUM SERIES ON COMPUTATIONAL INTELLIGENCE (IEEE SSCI)(2015)
摘要
The use of phase-type distributions is an established method for extending the representational power of continuous time Bayesian networks beyond exponentially-distributed state transitions. In this paper, we propose a method for learning phase-type distributions from known parametric distributions. We find that by using particle swarm optimization to minimize a modified KL-divergence value, we are able to efficiently obtain good phase-type approximations for a variety of parametric distributions. Our experiments show that particle swarm optimization outperforms genetic algorithms and hill climbing with simulated annealing. In addition, we investigate the trade-off between accuracy and complexity with respect to the number of phases in the phase-type distribution. Finally, we propose and evaluate an extension that uses informed starting locations during optimization, which we found to improve convergence rates when compared to random initialization.
更多查看译文
关键词
particle swarm optimization,exponential distribution,markov processes,weibull distribution
AI 理解论文
溯源树
样例
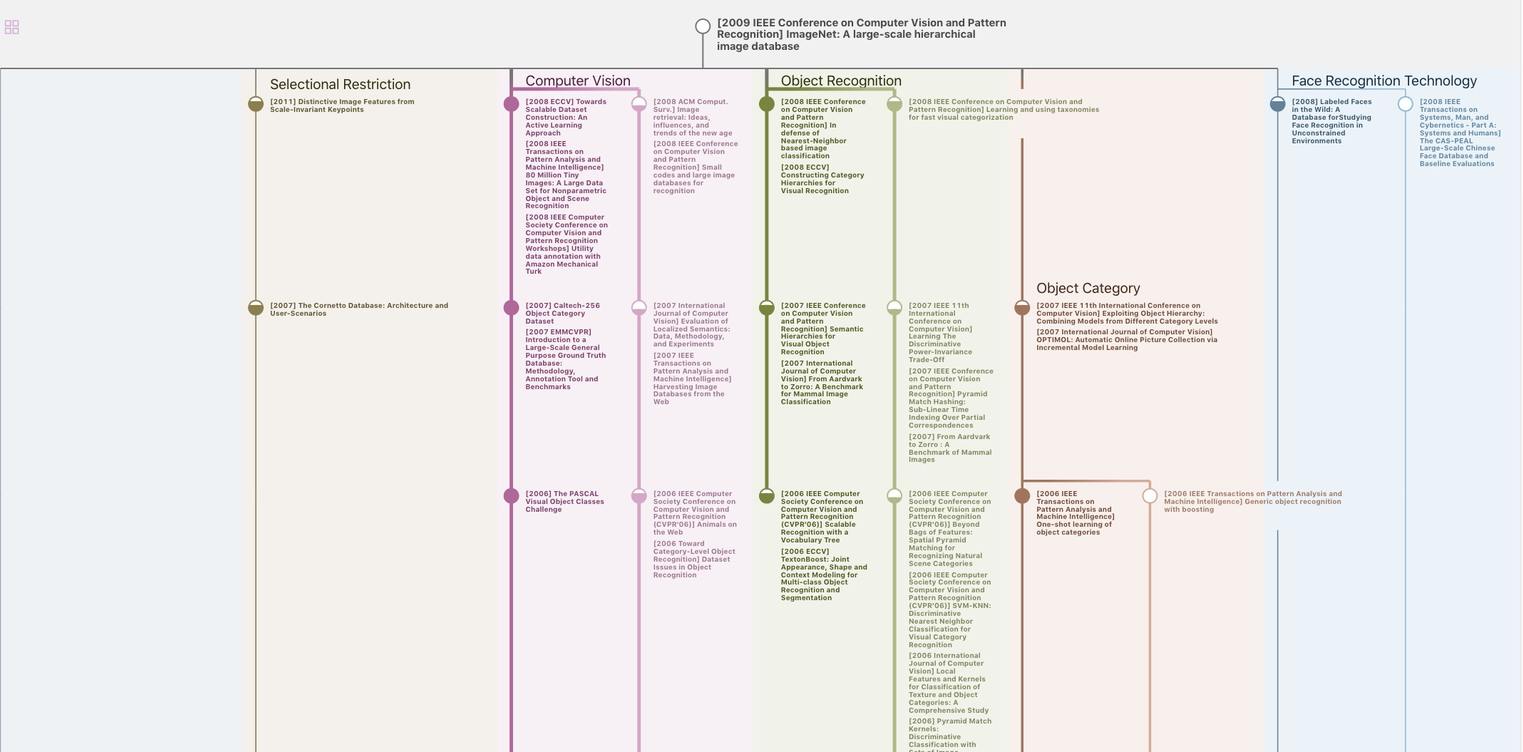
生成溯源树,研究论文发展脉络
Chat Paper
正在生成论文摘要