Feature-driven model-based segmentation
Proceedings of SPIE(2011)
摘要
The accurate delineation of anatomical structures is required in many medical image analysis applications. One example is radiation therapy planning (RTP), where traditional manual delineation is tedious, labor intensive, and can require hours of clinician's valuable time. Majority of automated segmentation methods in RTP belong to either model-based or atlas-based approaches. One substantial limitation of model-based segmentation is that its accuracy may be restricted by the uncertainties in image content, specifically when segmenting low-contrast anatomical structures, e. g. soft tissue organs in computed tomography images. In this paper, we introduce a non-parametric feature enhancement filter which replaces raw intensity image data by a high level probabilistic map which guides the deformable model to reliably segment low-contrast regions. The method is evaluated by segmenting the submandibular and parotid glands in the head and neck region and comparing the results to manual segmentations in terms of the volume overlap. Quantitative results show that we are in overall good agreement with expert segmentations, achieving volume overlap of up to 80%. Qualitatively, we demonstrate that we are able to segment low-contrast regions, which otherwise are difficult to delineate with deformable models relying on distinct object boundaries from the original image data.
更多查看译文
关键词
model-based segmentation,probability maps,voxel classification,radiation therapy,head and neck cancer
AI 理解论文
溯源树
样例
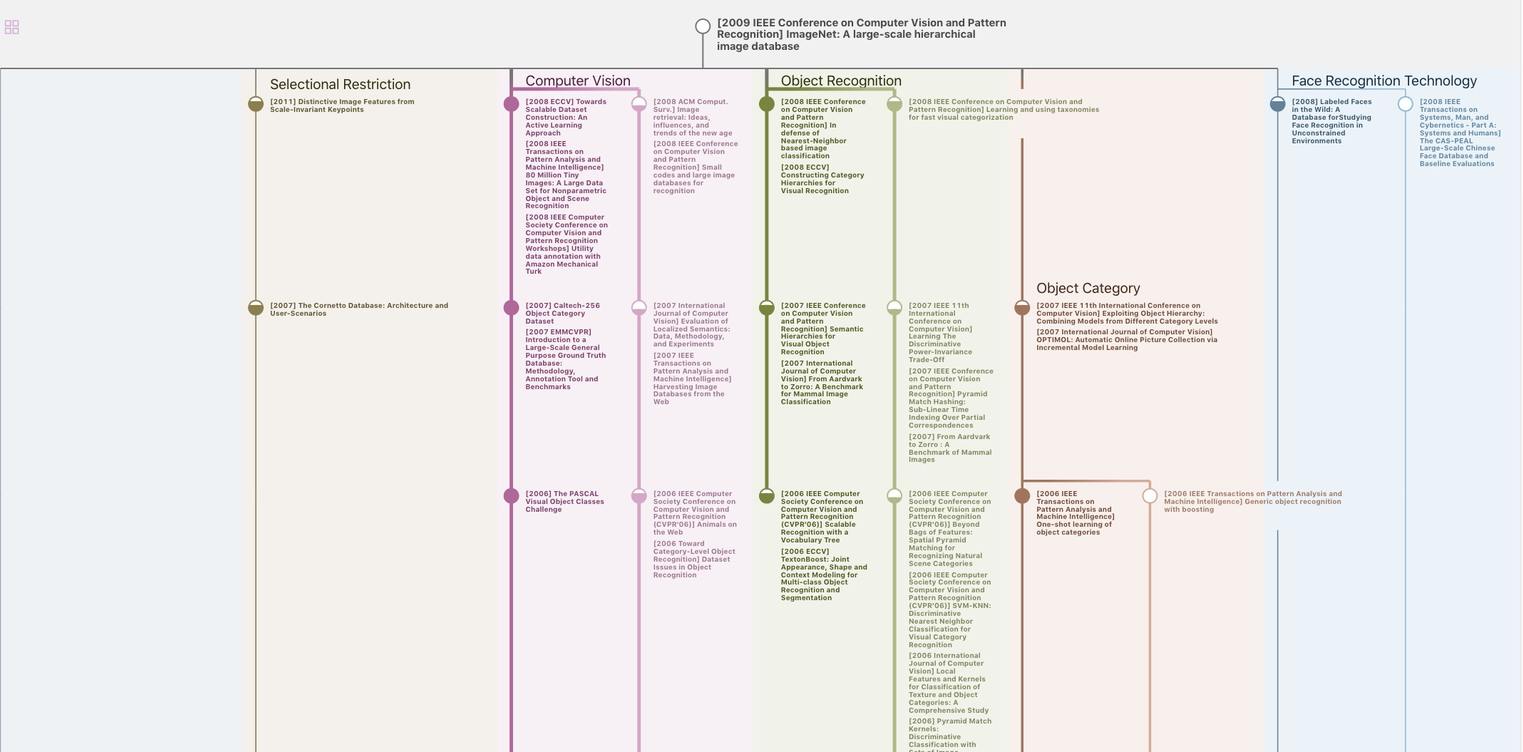
生成溯源树,研究论文发展脉络
Chat Paper
正在生成论文摘要