Bayesian Inference of Arrival Rate and Substitution Behavior from Sales Transaction Data with Stockouts
KDD '16: The 22nd ACM SIGKDD International Conference on Knowledge Discovery and Data Mining San Francisco California USA August, 2016(2016)
摘要
When an item goes out of stock, sales transaction data no longer reflect the original customer demand, since some customers leave with no purchase while others substitute alternative products for the one that was out of stock. Here we develop a Bayesian hierarchical model for inferring the underlying customer arrival rate and choice model from sales transaction data and the corresponding stock levels. The model uses a nonhomogeneous Poisson process to allow the arrival rate to vary throughout the day, and allows for a variety of choice models. Model parameters are inferred using a stochastic gradient MCMC algorithm that can scale to large transaction databases. We fit the model to data from a local bakery and show that it is able to make accurate out-of-sample predictions, and to provide actionable insight into lost cookie sales.
更多查看译文
关键词
Bayesian analysis,Langevin dynamics,Markov chain Monte Carlo,sales transaction data
AI 理解论文
溯源树
样例
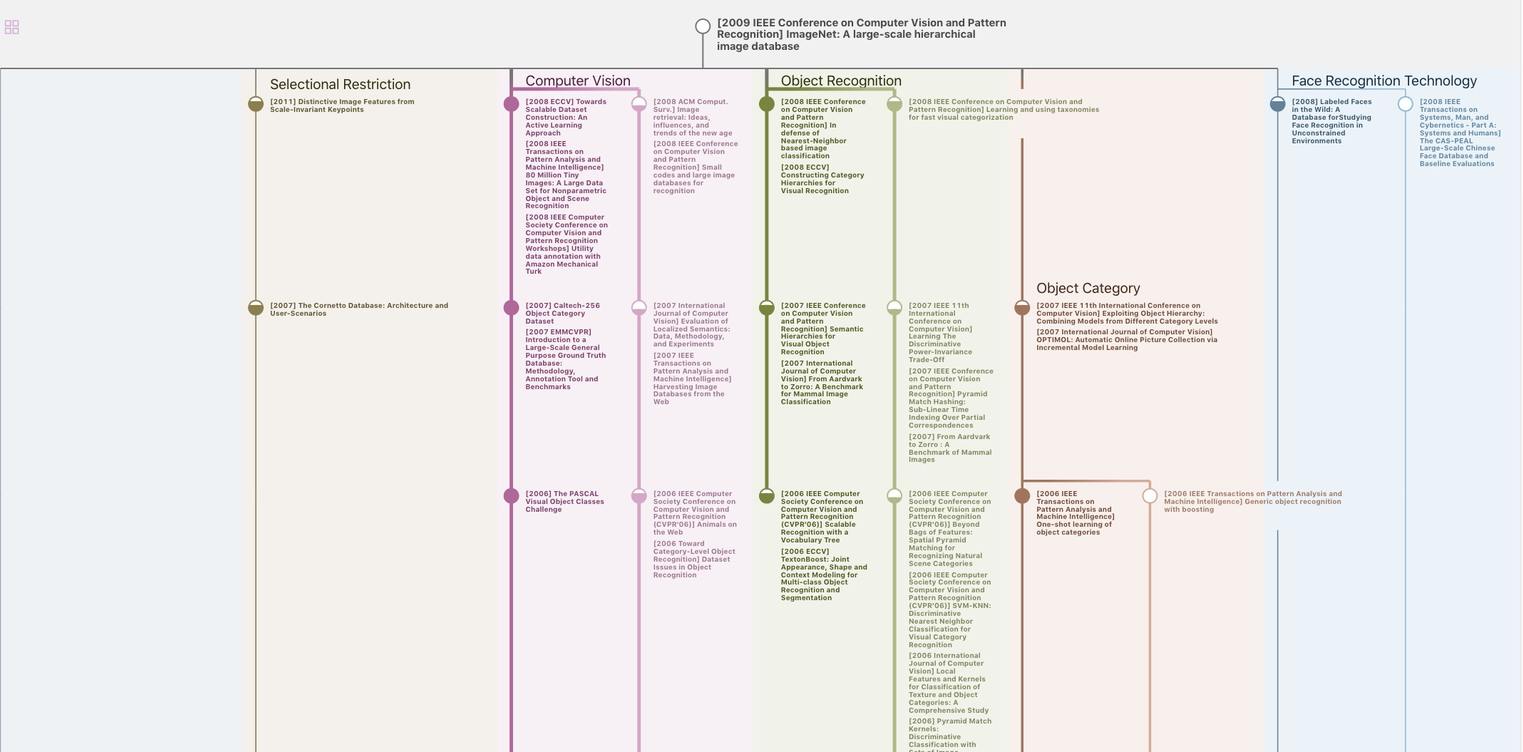
生成溯源树,研究论文发展脉络
Chat Paper
正在生成论文摘要