Feature Extraction For Bearing Fault Diagnosis Using Composite Multiscale Entropy
2013 IEEE/ASME INTERNATIONAL CONFERENCE ON ADVANCED INTELLIGENT MECHATRONICS (AIM): MECHATRONICS FOR HUMAN WELLBEING(2013)
摘要
Multiscale entropy (MSE) is a popular algorithm to measure the complexity of a time series for multiple scales. However, the conventional MSE algorithm yields imprecise estimation of entropy for a time series with large time scale factors. In this paper, a composite multiscale entropy (CMSE) method is proposed to overcome this drawback. In the CMSE algorithm, with scale factors of tau, we calculate the sample entropies (SampEns) of all coarse-grained series and then define the mean of tau SampEns as the entropy values. This proposed algorithm is then applied to two different kinds of simulated noise signals and a set of real vibration data. These results demonstrate that the proposed CMSE provides more precise entropy calculation than the convectional MSE. Furthermore, as a feature extractor for a bearing faulty signal, CMSE provides a higher distinguishability, compared with MSE.
更多查看译文
关键词
noise,time series,feature extraction,mse,time series analysis,algorithm design and analysis,entropy,vibrations
AI 理解论文
溯源树
样例
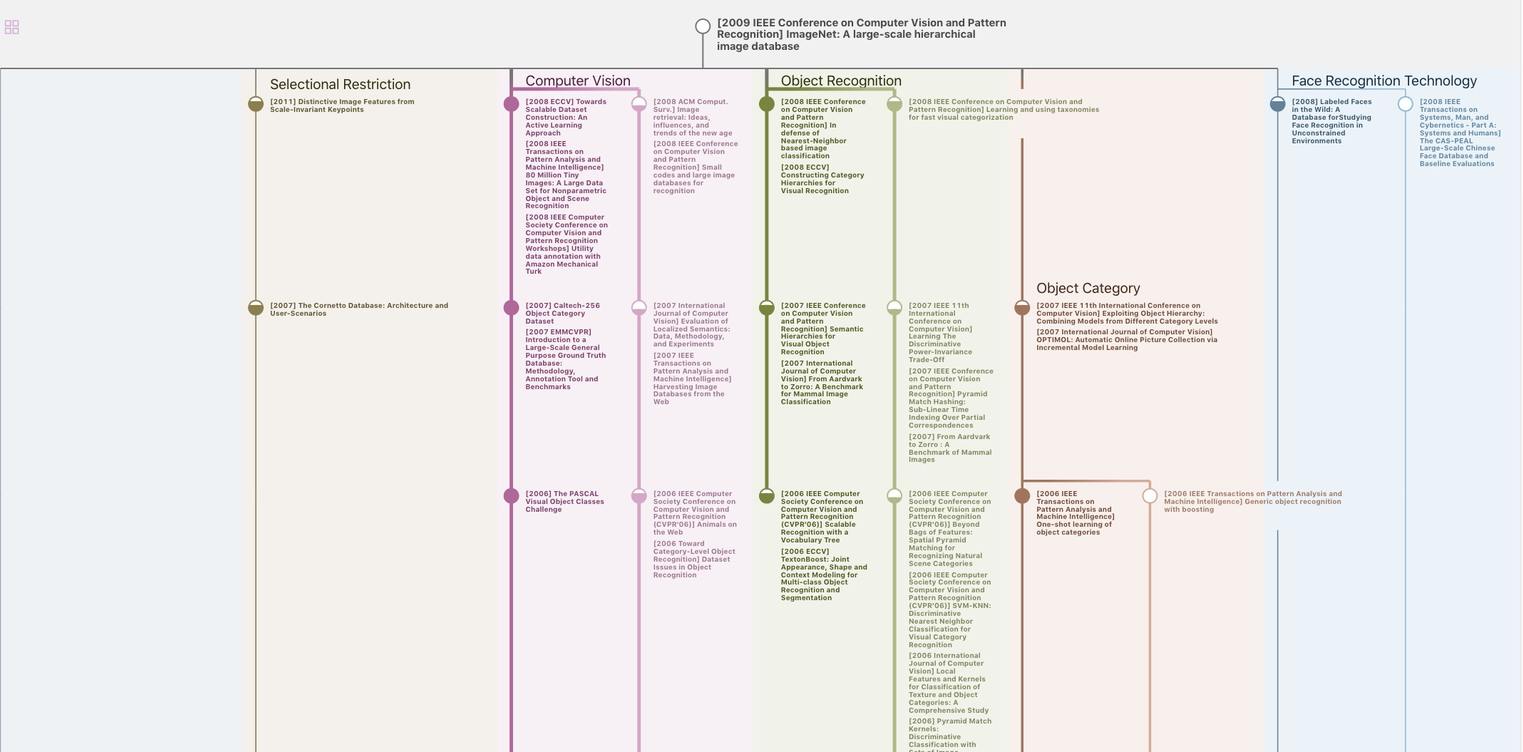
生成溯源树,研究论文发展脉络
Chat Paper
正在生成论文摘要