Information-decomposition-model-based missing value estimation for not missing at random dataset
Int. J. Machine Learning & Cybernetics(2015)
摘要
Missing data estimation is an important strategy for improving learning performance in learning from incomplete data, especially, when there are non discardable records with missing values. However, most of the existing algorithms are focused on missing at random (MAR) or missing completely at random (MCAR), and less attention has been paid to data not missing at random (NMAR). In this paper, an information decomposition imputation (IDIM) algorithm using fuzzy membership function is proposed for addressing the missing value problem under NMAR. Firstly, the proposed IDIM algorithm is presented with detailed examples. Then, the proposed approach is evaluated with extensive experiments compared with some typical algorithms. The experimental results demonstrate that the proposed algorithm has higher accuracy than the exiting imputation approaches in terms of normal root mean square error (NRMSE) and TP+TN evaluation under different missing strategies.
更多查看译文
关键词
Data mining,Machine learning,Estimation,Algorithm,Missing value
AI 理解论文
溯源树
样例
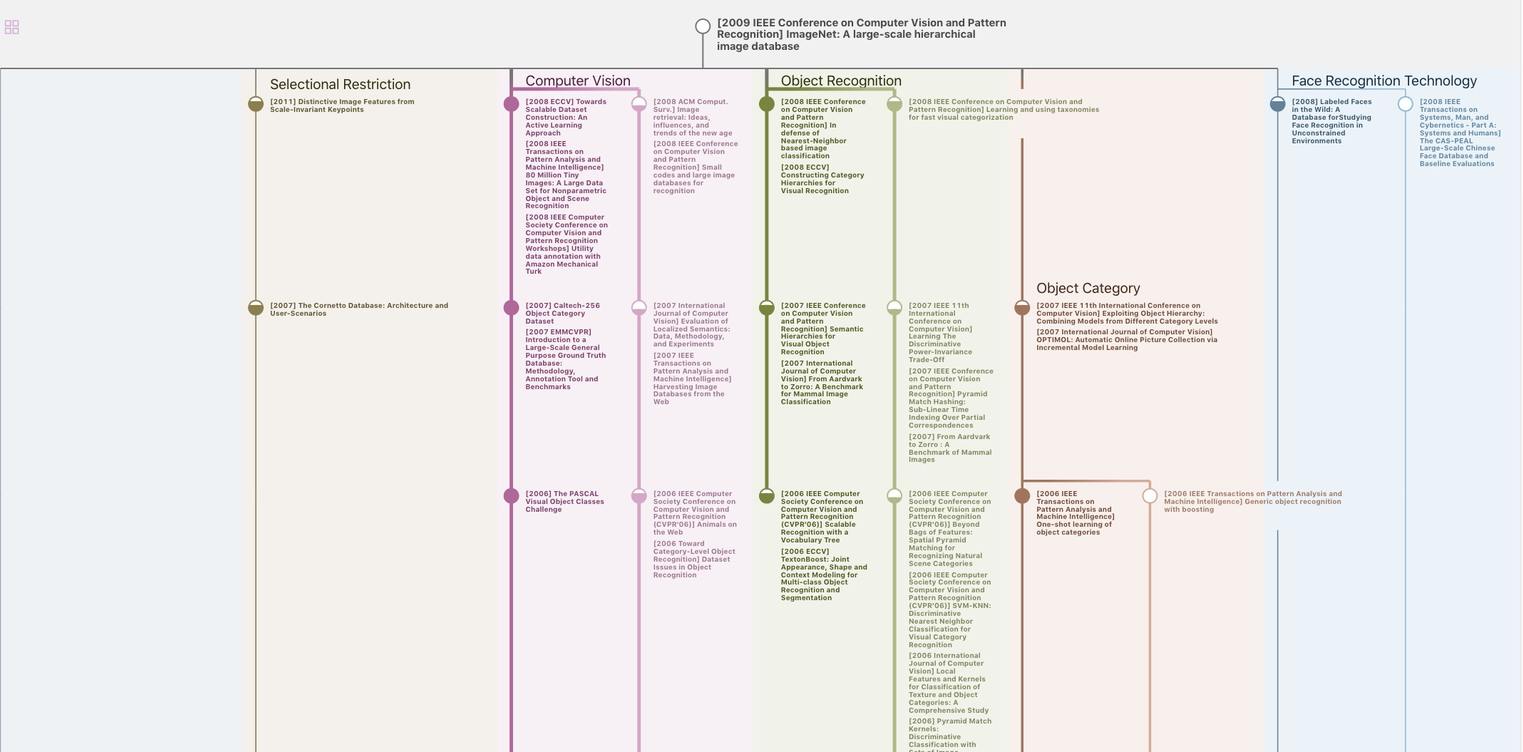
生成溯源树,研究论文发展脉络
Chat Paper
正在生成论文摘要