Learning the Intrinsic Dimensions of the Timit Speech Database with Maximum Variance Unfolding
2009 IEEE 13th Digital Signal Processing Workshop and 5th IEEE Signal Processing Education Workshop(2009)
摘要
Modern methods for nonlinear dimensionality reduction have been used extensively in the machine learning community for discovering the intrinsic dimension of several datasets. In this paper we apply one of the most successful ones maximum variance unfolding on a big sample of the well known speech benchmark TIMIT. Although MVU is not generally scalable, we managed to apply to 1 million 39-dimensional points and successfully reduced the dimension down to 15. In this paper we apply some of the state-of-the-art techniques for handling big datasets. The biggest bottleneck is the local neighborhood computation. For 300 K points it took 9 hours while for 1 M points it took 3.5 days. We also demonstrate the weakness of MFCC representation under the k-nearest neighborhood classification since the error rate is more than 50%.
更多查看译文
关键词
Maximum Variance Unfolding,Manifold Learning,Dimensionality Reduction,Speech,Mel Cepstrum
AI 理解论文
溯源树
样例
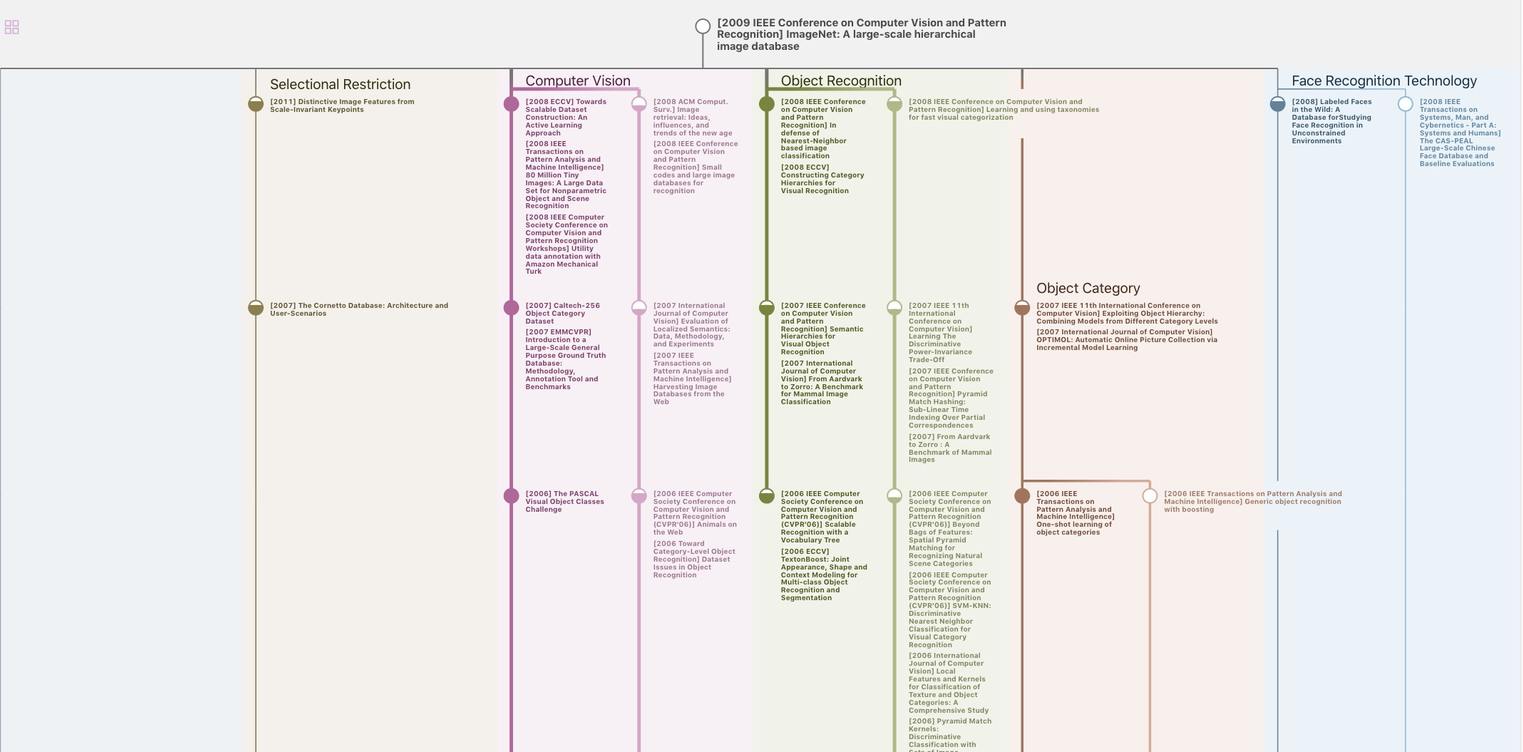
生成溯源树,研究论文发展脉络
Chat Paper
正在生成论文摘要