Gaussian Mixture Models
AUTOMATIC SPEECH RECOGNITION: A DEEP LEARNING APPROACH(2015)
摘要
In this chapter we first introduce the basic concepts of random variables and the associated distributions. These concepts are then applied to Gaussian random variables and mixture-of-Gaussian random variables. Both scalar and vector-valued cases are discussed and the probability density functions for these random variables are given with their parameters specified. This introduction leads to the Gaussian mixture model (GMM) when the distribution of mixture-of-Gaussian random variables is used to fit the real-world data such as speech features. The GMM as a statistical model for Fourier-spectrum-based speech features plays an important role in acoustic modeling of conventional speech recognition systems. We discuss some key advantages of GMMs in acoustic modeling, among which is the easy way of using them to fit the data of a wide range of speech features using the EM algorithm. We describe the principle of maximum likelihood and the related EM algorithm for parameter estimation of the GMM in some detail as it is still a widely used method in speech recognition. We finally discuss a serious weakness of using GMMs in acoustic modeling for speech recognition, motivating new models and methods that form the bulk part of this book.
更多查看译文
AI 理解论文
溯源树
样例
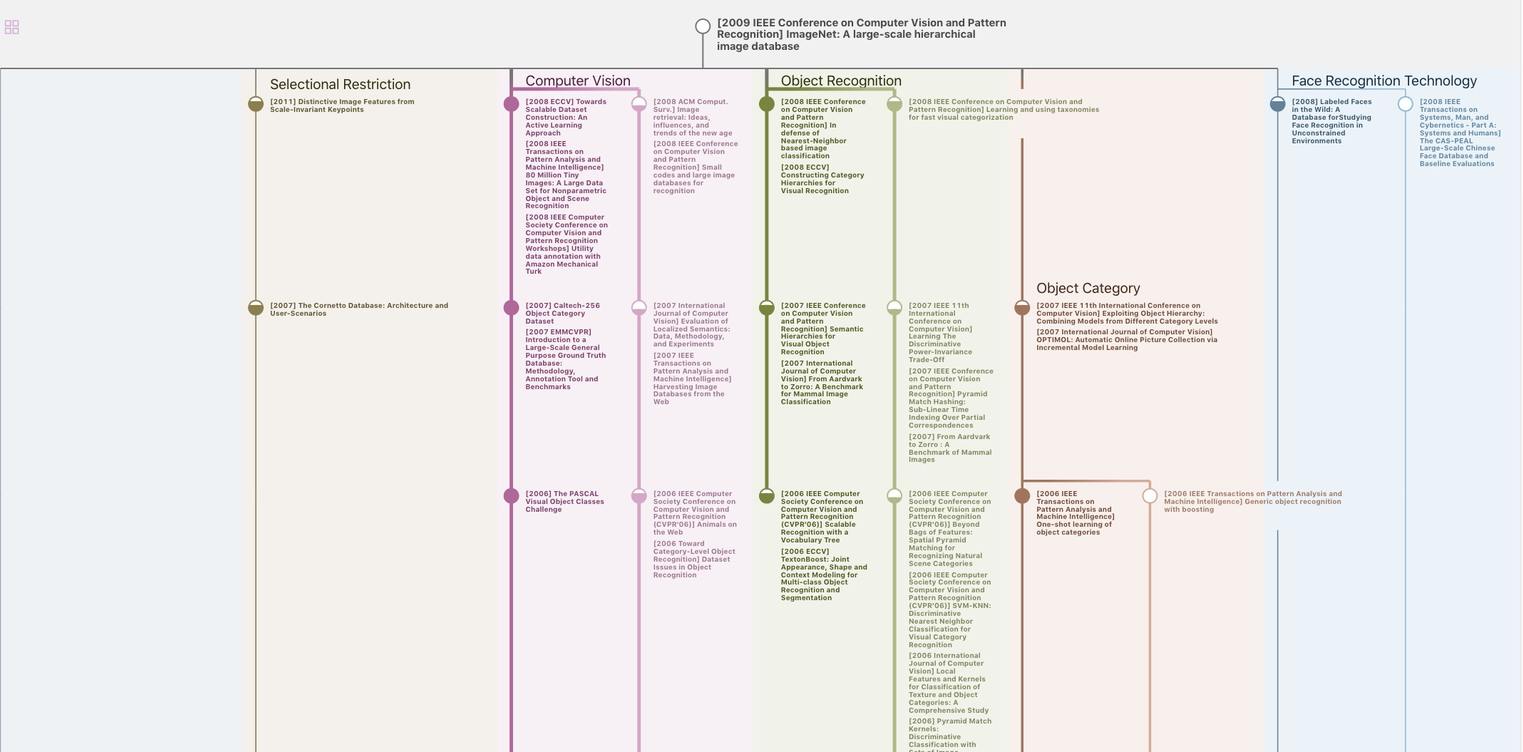
生成溯源树,研究论文发展脉络
Chat Paper
正在生成论文摘要