Distilling Informative Content From Html News Pages Using Machine Learning Classifiers
MINING FOR STRATEGIC COMPETITIVE INTELLIGENCE: FOUNDATIONS AND APPLICATIONS(2012)
摘要
Not only the Web abounds of information overload, but also its component molecules, the Web documents. In particular HTML news pages have become aggregates of cornucopian information blocks, such as advertisements, link lists, disclaimers and terms of use, or comments from readers. No more than an estimated 30%-70% of textual content appears dedicated to the actual news article itself.The amalgamation of relevant content with page clutter poses considerable concerns to applications that make use of such news information, e.g., retrieval-based applications, document clustering platforms or topic detection systems, among those our reputation monitoring solution [17].We present an approach geared towards dissecting relevant from irrelevant textual content in an automated fashion. Our system extracts linguistic and structural features from merged text segments and applies various classifiers thereafter.We have conducted empirical analyses, based on 600 labeled news documents in five different languages, in order to compare our approach's classification performance with a human gold standard as well as two benchmark systems.
更多查看译文
AI 理解论文
溯源树
样例
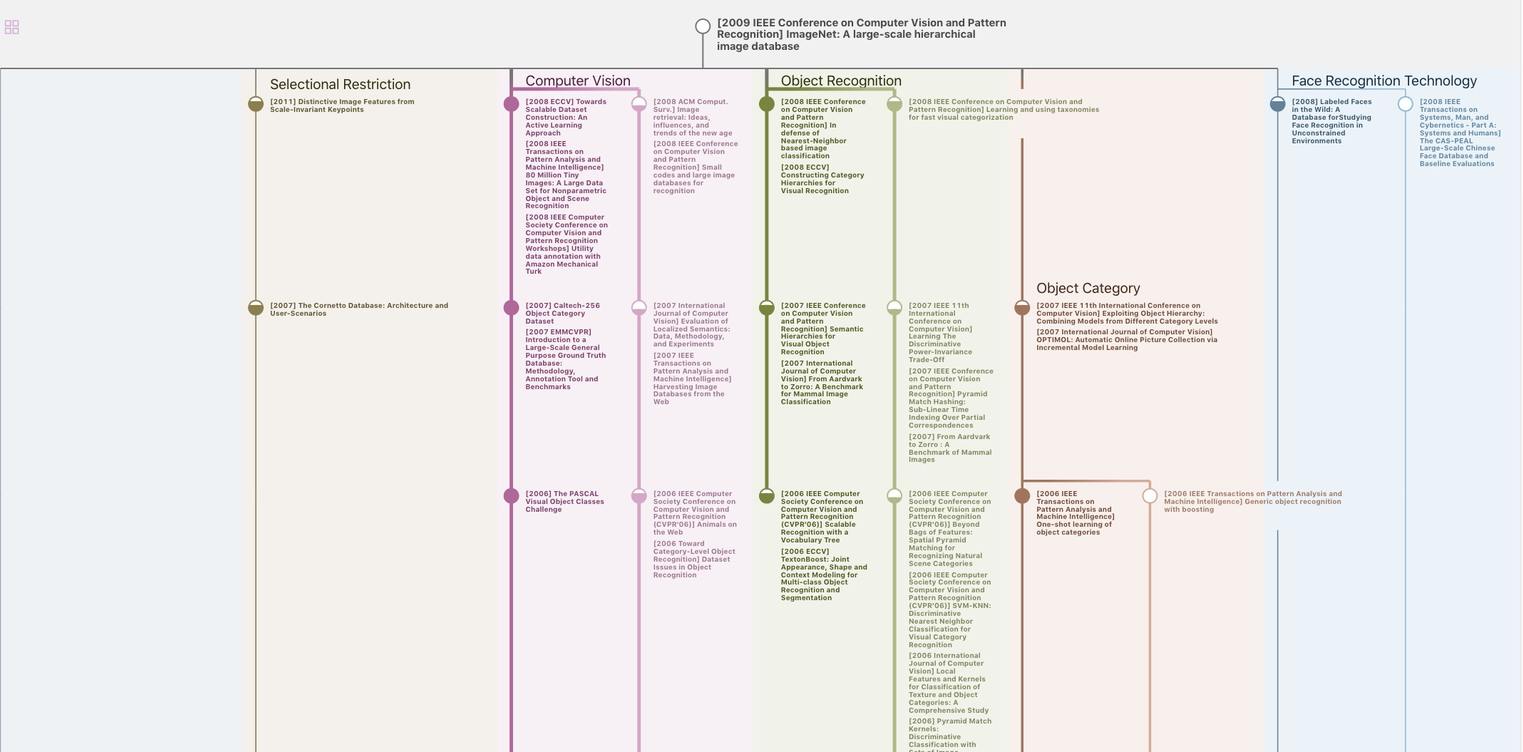
生成溯源树,研究论文发展脉络
Chat Paper
正在生成论文摘要