Consistent Nonparametric Estimation For Heavy-Tailed Sparse Graphs
ANNALS OF STATISTICS(2021)
摘要
We study graphons as a nonparametric generalization of stochastic block models, and show how to obtain compactly represented estimators for sparse networks in this framework. In contrast to previous work, we relax the usual boundedness assumption for the generating graphon and instead assume only integrability, so that we can handle networks that have long tails in their degree distributions. We also relax the usual assumption that the graphon is defined on the unit interval, to allow latent position graphs based on more general spaces.We analyze three algorithms. The first is a least squares algorithm, which gives a consistent estimator for all square-integrable graphons, with errors expressed in terms of the best possible stochastic block model approximation. Next, we analyze an algorithm based on the cut norm, which works for all integrable graphons. Finally, we show that clustering based on degrees works whenever the underlying degree distribution is atomless.
更多查看译文
关键词
Sparse networks, estimation, graphons
AI 理解论文
溯源树
样例
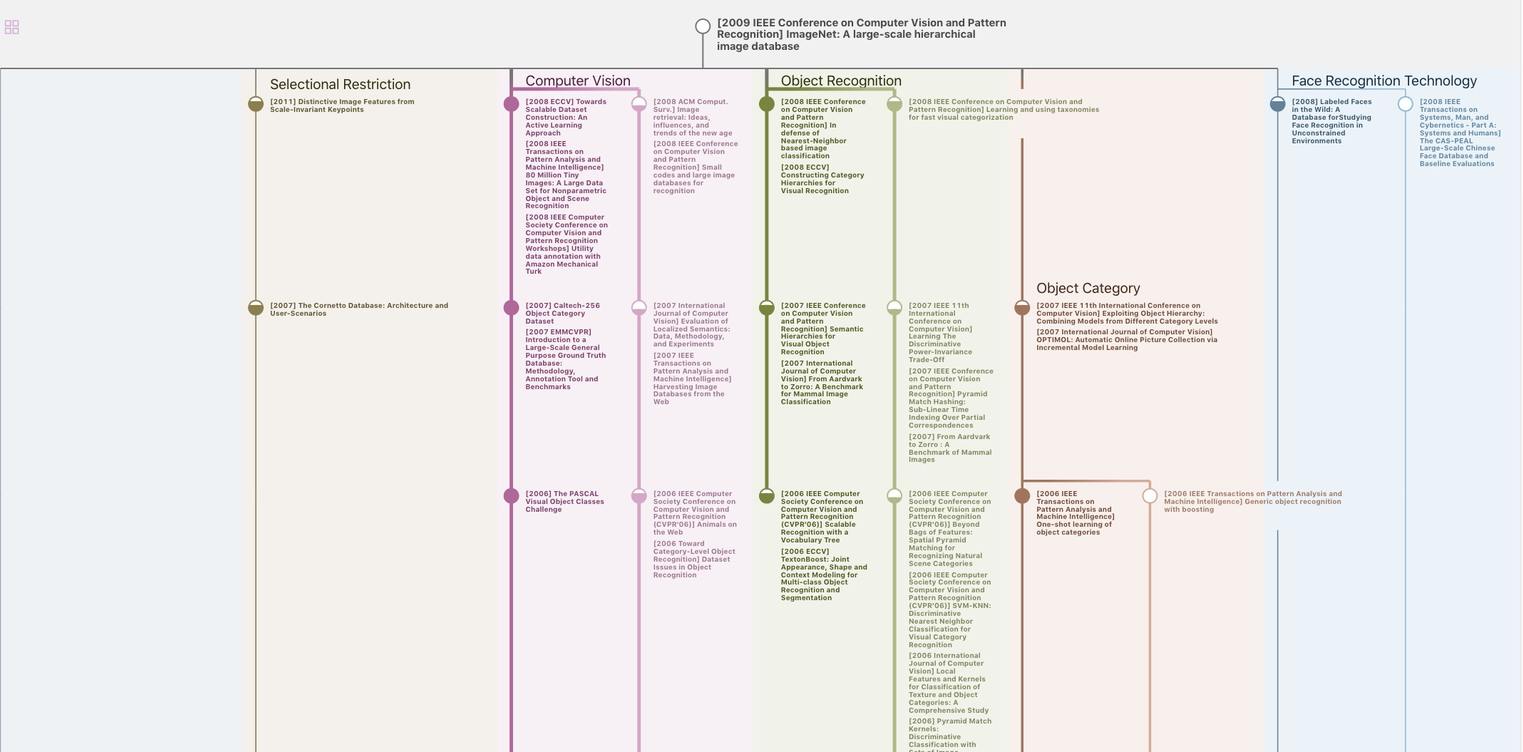
生成溯源树,研究论文发展脉络
Chat Paper
正在生成论文摘要