A Kernel Approach to Tractable Bayesian Nonparametrics
mag(2011)
摘要
Inference in popular nonparametric Bayesian models typically relies on sampling or other approximations. This paper presents a general methodology for constructing novel tractable nonparametric Bayesian methods by applying the kernel trick to inference in a parametric Bayesian model. For example, Gaussian process regression can be derived this way from Bayesian linear regression. Despite the success of the Gaussian process framework, the kernel trick is rarely explicitly considered in the Bayesian literature. In this paper, we aim to fill this gap and demonstrate the potential of applying the kernel trick to tractable Bayesian parametric models in a wider context than just regression. As an example, we present an intuitive Bayesian kernel machine for density estimation that is obtained by applying the kernel trick to a Gaussian generative model in feature space.
更多查看译文
关键词
parametric model,gaussian process,bayesian model,gaussian process regression,density estimation,linear regression,kernel machine,feature space,bayesian method
AI 理解论文
溯源树
样例
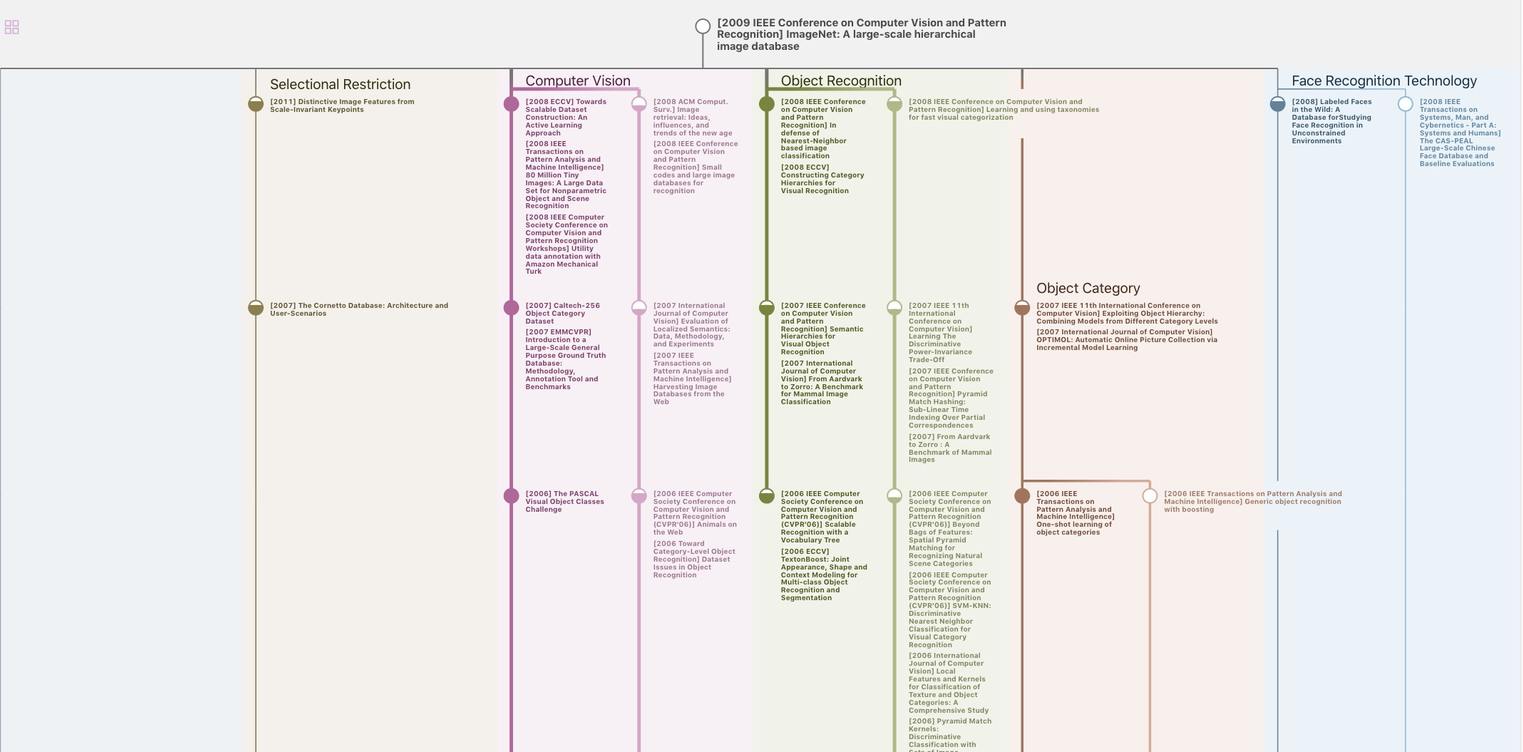
生成溯源树,研究论文发展脉络
Chat Paper
正在生成论文摘要