A COMPARISON STUDY OF DIFFERENT KERNEL FUNCTIONS FOR SVM-BASED CLASSIFICATION OF MULTI-TEMPORAL POLARIMETRY SAR DATA
International Archives of the Photogrammetry Remote Sensing and Spatial Information Sciences(2014)
摘要
In this paper, a framework is developed based on Support Vector Machines (SVM) for crop classification using polarimetric features extracted from multi-temporal Synthetic Aperture Radar (SAR) imageries. The multi-temporal integration of data not only improves the overall retrieval accuracy but also provides more reliable estimates with respect to single-date data. Several kernel functions are employed and compared in this study for mapping the input space to higher Hilbert dimension space. These kernel functions include linear, polynomials and Radial Based Function (RBF). The method is applied to several UAVSAR L-band SAR images acquired over an agricultural area near Winnipeg, Manitoba, Canada. In this research, the temporal alpha features of H/A/alpha decomposition method are used in classification. The experimental tests show an SVM classifier with RBF kernel for three dates of data increases the Overall Accuracy (OA) to up to 3% in comparison to using linear kernel function, and up to 1% in comparison to a 3rd degree polynomial kernel function.
更多查看译文
关键词
Full-polarimetric SAR data,multi-temporal data,SVM,kernel functions,cop classification
AI 理解论文
溯源树
样例
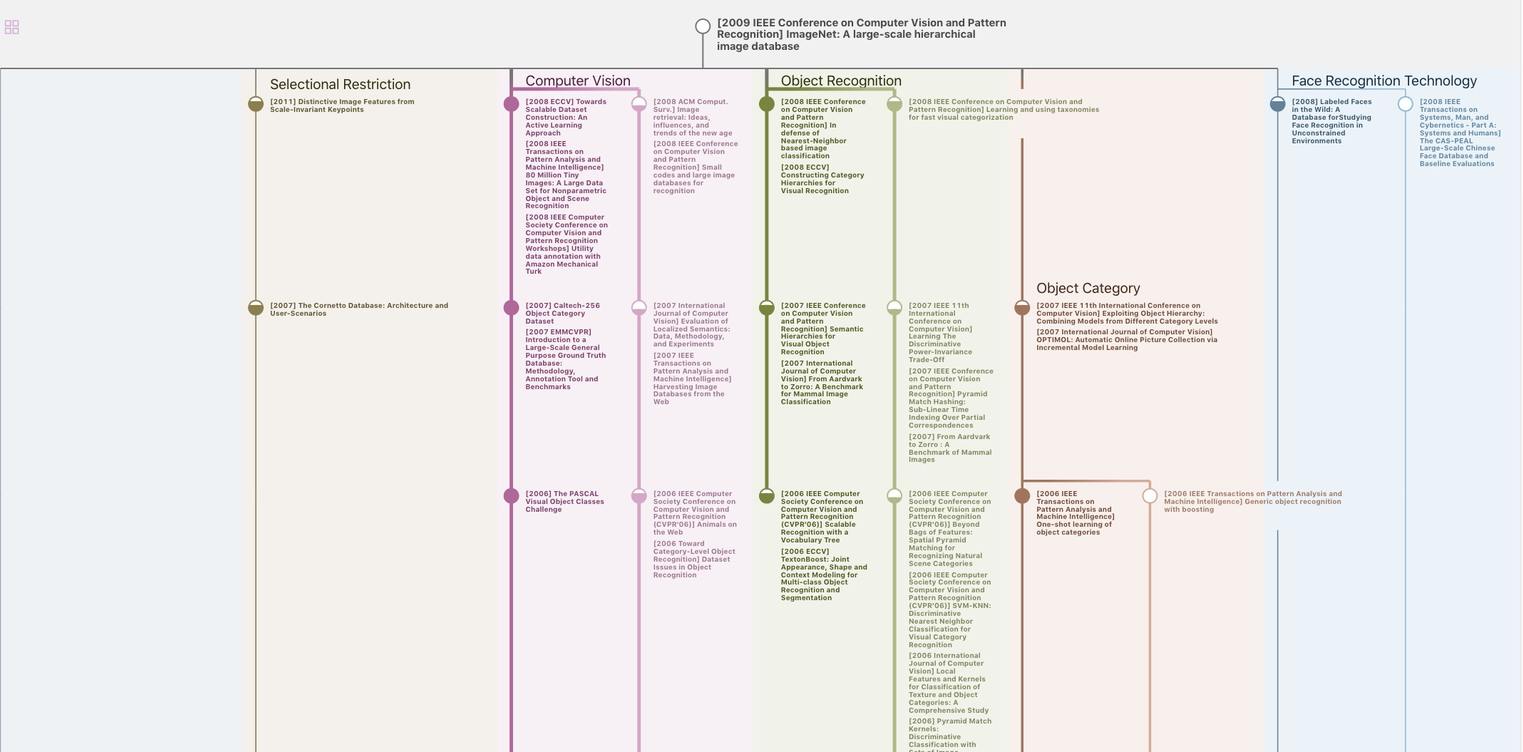
生成溯源树,研究论文发展脉络
Chat Paper
正在生成论文摘要