TPRM: Tensor partition regression models with applications in imaging biomarker detection
ANNALS OF APPLIED STATISTICS(2018)
摘要
Medical imaging studies have collected high-dimensional imaging data to identify imaging biomarkers for diagnosis, screening, and prognosis, among many others. These imaging data are often represented in the form of a multi-dimensional array, called a tensor. The aim of this paper is to develop a tensor partition regression modeling (TPRM) framework to establish a relationship between low-dimensional clinical outcomes (e.g., diagnosis) and high-dimensional tensor covariates. Our TPRM is a hierarchical model and efficiently integrates four components: (i) a partition model, (ii) a canonical polyadic decomposition model, (iii) a principal components model, and (iv) a generalized linear model with a sparse inducing normal mixture prior. This framework not only reduces ultra-high dimensionality to a manageable level, resulting in efficient estimation, but also optimizes prediction accuracy in the search for informative sub-tensors. Posterior computation proceeds via an efficient Markov chain Monte Carlo algorithm. Simulation shows that TPRM outperforms several other competing methods. We apply TPRM to predict disease status (Alzheimer versus control) by using structural magnetic resonance imaging data obtained from the Alzheimer's Disease Neuroimaging Initiative (ADNI) study.
更多查看译文
关键词
Bayesian hierarchical model,big data,MCMC,tensor decomposition,tensor regression
AI 理解论文
溯源树
样例
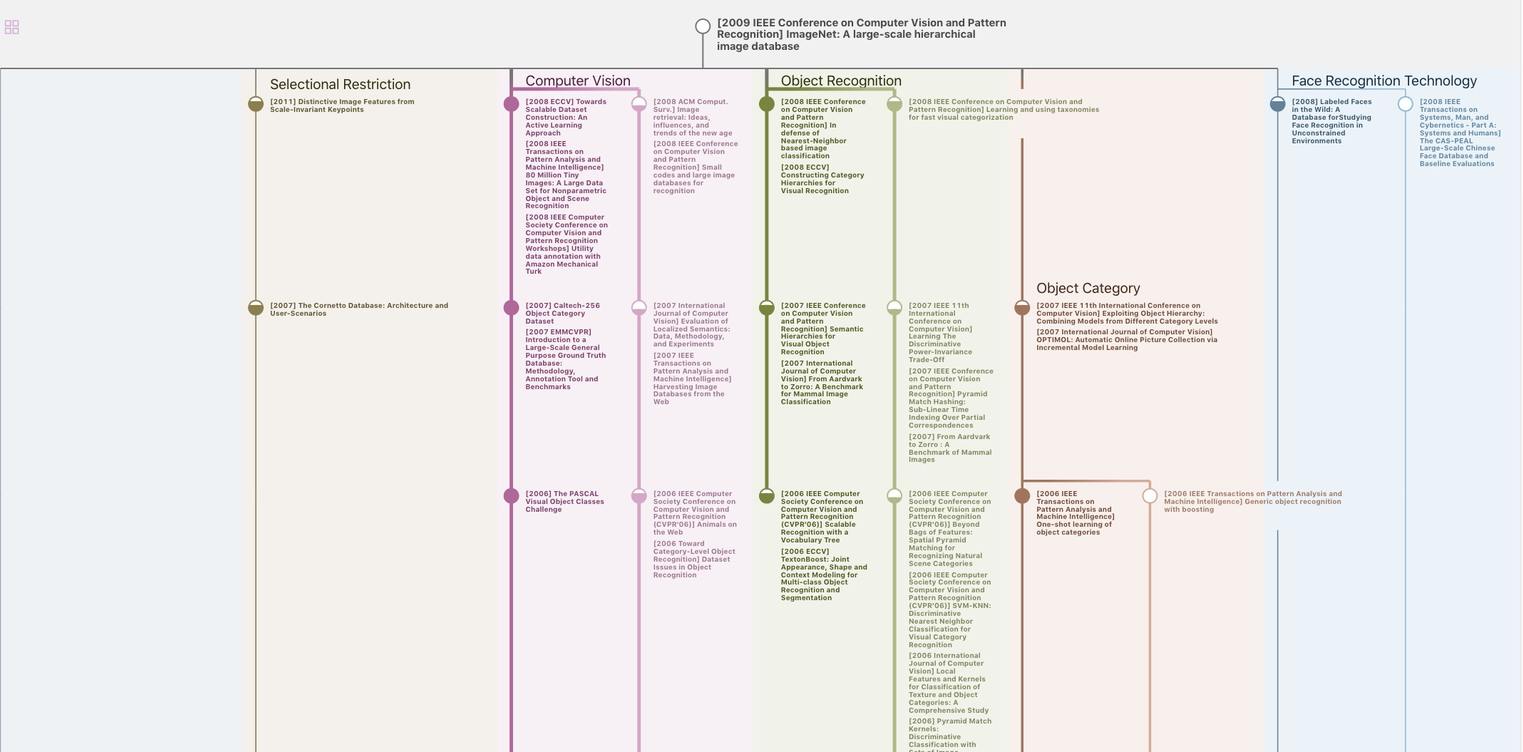
生成溯源树,研究论文发展脉络
Chat Paper
正在生成论文摘要