Pedestrian Recognition By Using A Dynamic Modality Selection Approach
2015 IEEE 18TH INTERNATIONAL CONFERENCE ON INTELLIGENT TRANSPORTATION SYSTEMS(2015)
摘要
Despite many years of research, pedestrian recognition is still a difficult, but very important task. It was proved that concatenating information from multi-modality images improves the recognition accuracy, but with a high computational cost. We present a modality selection approach, which is able to dynamically select the most discriminative modality for a given image and furthermore use it in the classification process. Firstly, we extract kernel descriptor features from a given image in three modalities: intensity, depth and flow. Secondly, we dynamically determine the most suitable modality for that image using both: a modality pertinence classifier and a decision confidence indicator. Thirdly, we classify the image in the selected modality using a linear SVM approach. Numerical experiments are performed on the Daimler benchmark dataset consisting of pedestrian and non-pedestrian bounding boxes captured in outdoor urban environments and indicate that our model outperforms all the individual-modality classifiers and the model based on a posterior fusion of multi-modality decisions. Moreover, the proposed selection model is a promising and less computational expensive alternative to the concatenation of multi-modality features prior to classification.
更多查看译文
关键词
multimodality decision fusion,individual-modality classifier,Daimler benchmark dataset,support vector machines,linear SVM approach,decision confidence indicator,modality pertinence classifier,flow modality,depth modality,intensity modality,kernel descriptor feature extraction,image classification,multimodality image concatenation,dynamic modality selection approach,pedestrian recognition
AI 理解论文
溯源树
样例
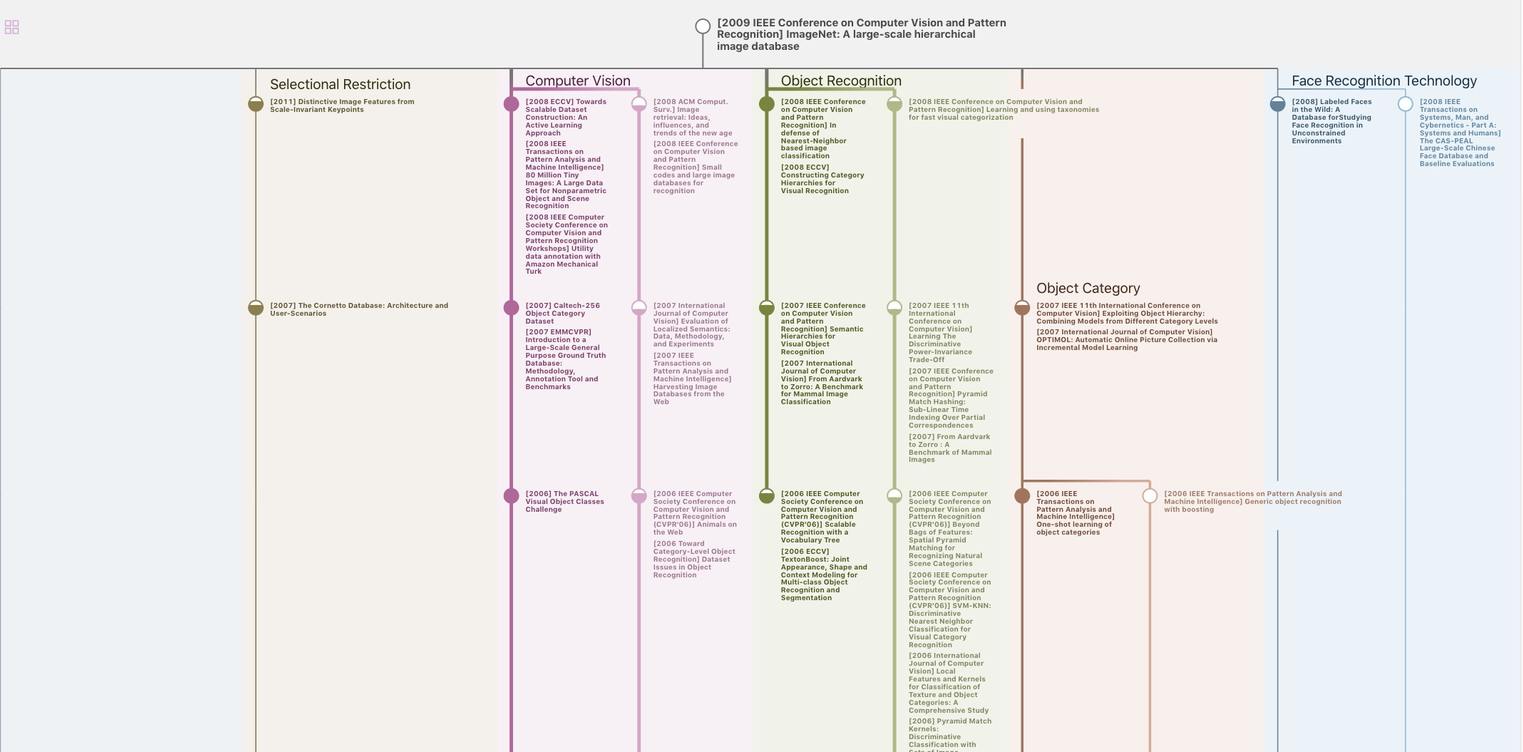
生成溯源树,研究论文发展脉络
Chat Paper
正在生成论文摘要