Inference for Monotone Trends Under Dependence
arXiv: Statistics Theory(2014)
摘要
We focus on the problem estimating a monotone trend function under additive and dependent noise. New point-wise confidence interval estimators under both short- and long-range dependent errors are introduced and studied. These intervals are obtained via the method of inversion of certain discrepancy statistics arising in hypothesis testing problems. The advantage of this approach is that it avoids the estimation of nuisance parameters such as the derivative of the unknown function, which existing methods are forced to deal with. While the methodology is motivated by earlier work in the independent context, the dependence of the errors, especially longrange dependence leads to new challenges, such as the study of convex minorants of drifted fractional Brownian motion that may be of independent interest. We also unravel a new family of universal limit distributions (and tabulate selected quantiles) that can henceforth be used for inference in monotone function problems involving dependence.
更多查看译文
AI 理解论文
溯源树
样例
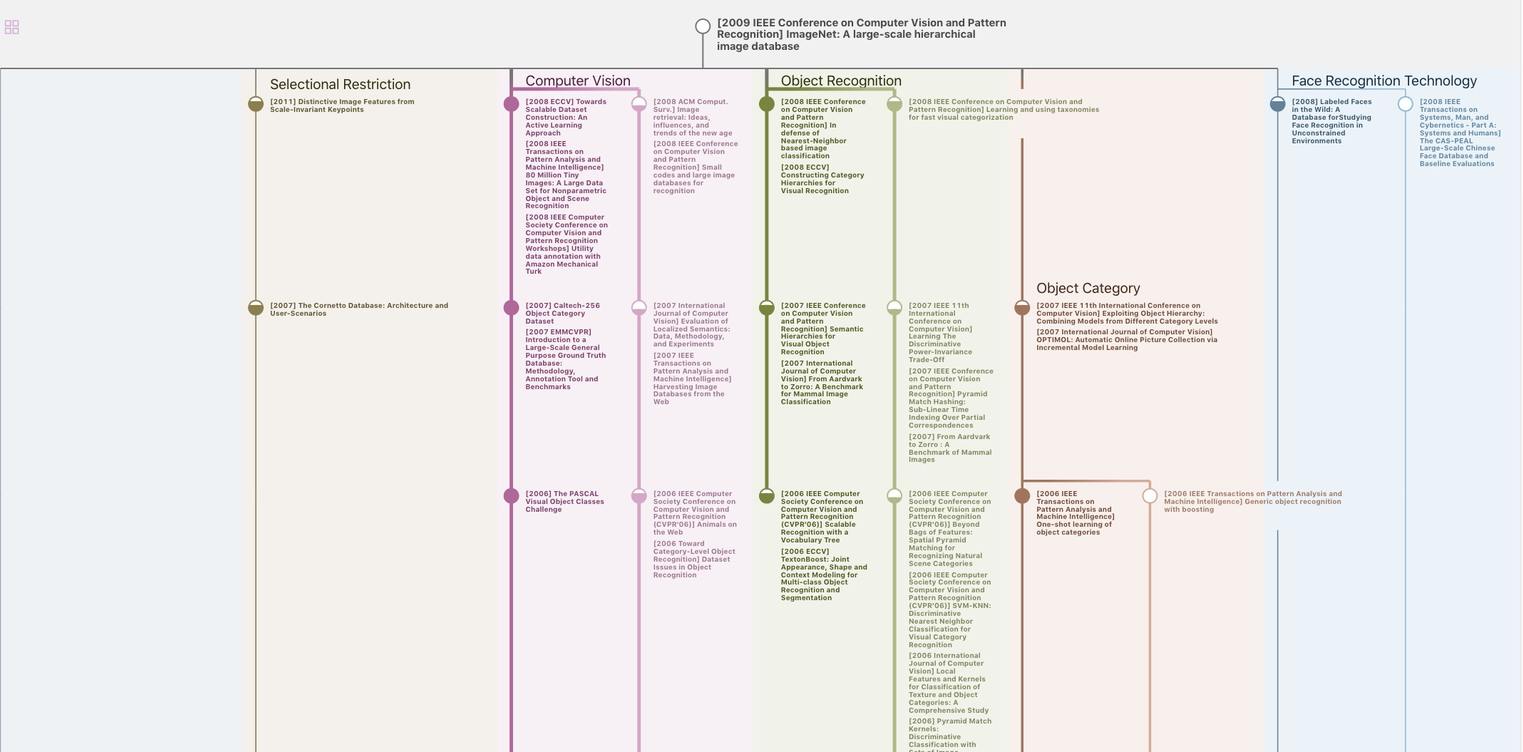
生成溯源树,研究论文发展脉络
Chat Paper
正在生成论文摘要