A bioinformatics framework for the identification of active regulatory elements through the integrative analysis of high-throughput genomic data
EMBnet.journal(2012)
摘要
Motivations. High-throughput technologies as microarray, Cap Analysis of Gene Expression (CAGE), and chromatin immunoprecipitation (ChIP), coupled with next generation sequencing (NGS) allow the identification of the molecular mechanisms regulating DNA transcription. In particular, CAGE and chromatin immunoprecipitation followed by DNA sequencing (ChIP-seq) are particularly suited to analyze transcriptional regulation and DNA methylation and histone modification patterns, while gene expression microarrays allow assessing transcriptional patterns. All these technologies produce data that are highly informative per se, but merging and integrating the various types of information would help answering many long-standing questions related to fundamental mechanisms of gene regulation and genome utilization. Data integration can be addressed using three main approaches, i.e., reduction of data complexity, unsupervised integration, and supervised integration [1]. Although these methods proved their efficiency in the analysis of single types of data, no bioinformatics tool integrates them all in a unified pipeline. In this context, we present a bioinformatics framework for the integrative analysis of CAGE, ChIP-Seq, and microarray data and the genome-wide identification of active regulatory elements. The computational workflow comprises three steps, i.e., i) stand-alone analysis of CAGE, ChIP-Seq, and microarray data; ii) construction of a unified data structure where results from CAGE, ChIP-Seq, and microarray analysis converge to generate integrated patterns of genomic signals; and iii) analysis of the integrated patterns to identify active regulatory elements. Methods. CAGE data have been analyzed using standard methods [2]. ChIP-seq peak calling was obtained using SICER [3]. Microarray data have been processed using PREDA for the detection of chromosomal patterns of gene expression [4]. Results from stand-alone analyses have been integrated in a unique matrix based on the UCSC BED file structure and on the chromosomal coordinate of genomic elements. The analysis of the integrated matrix has been performed using an ad-hoc procedure coded in R for the identification of i) enhancers, ii) non-coding RNA, and iii) promoters. The algorithm comprises three steps: in the first, CAGE and ChIP-seq peaks are merged to determine which histone modifications are present in correspondence of a CAGE peak. CAGE peaks that fall within H3K4me1 regions are classified as associated with a putative enhancer, while peaks that fall into both H3K4me1 and H3K4me3 regions are classified as associated with a putative promoter. In the second step, the algorithm verifies if there exists bi-directional transcription between two consecutive CAGE peaks located on opposite strands. Briefly, given any two peaks on opposite strands, an R function calculates the distance between them and labels as bi-directionally transcribed those peaks lying at a distance ≤ 1 kb. All other peaks are marked as mono-directionally transcribed. In the last step, the presence of putative enhancers and promoters is linked to the local pattern of gene expression. Results. The pipeline has been applied for the genome-wide characterization of mono-directionally and bi-directionally transcribed promoters and enhancers involved in self-renewal, commitment, and differentiation of human stem/progenitor cells and their progeny. Specifically, we analyzed data from cord-blood derived hematopoietic stem cells (HSCs) and lineage-restricted erythroblasts and myelomonocytes identifying specific regulatory regions differentially engaged during HSC differentiation. This work is supported by FIRB – Programma “Futuro in Ricerca” 2010 (RBFR10OS4G) and Progetto Bandiera Epigenomica. References 1. Hawkins RD, Hon GC, Ren B. Next-generation genomics: an integrative approach. Nat Rev Genet. 2010 Jul;11(7):476-86 2. Kodzius R, Kojima M, Nishiyori H, Nakamura M, Fukuda S, Tagami M, Sasaki D, Imamura K, Kai C, Harbers M, Hayashizaki Y, Carninci P. CAGE: cap analysis of gene expression. Nat Methods. 2006 Mar;3(3):211-22 3. Zang C, Schones DE, Zeng C, Cui K, Zhao K, Peng W. A clustering approach for identification of enriched domains from histone modification ChIP-Seq data. Bioinformatics. 2009 Aug 1; 25(15):1952-8 4. Ferrari F, Solari A, Battaglia C, Bicciato S. PREDA: an R-package to identify regional variations in genomic data. Bioinformatics. 2011 Sep 1;27(17):2446-7
更多查看译文
关键词
high throughput,bits
AI 理解论文
溯源树
样例
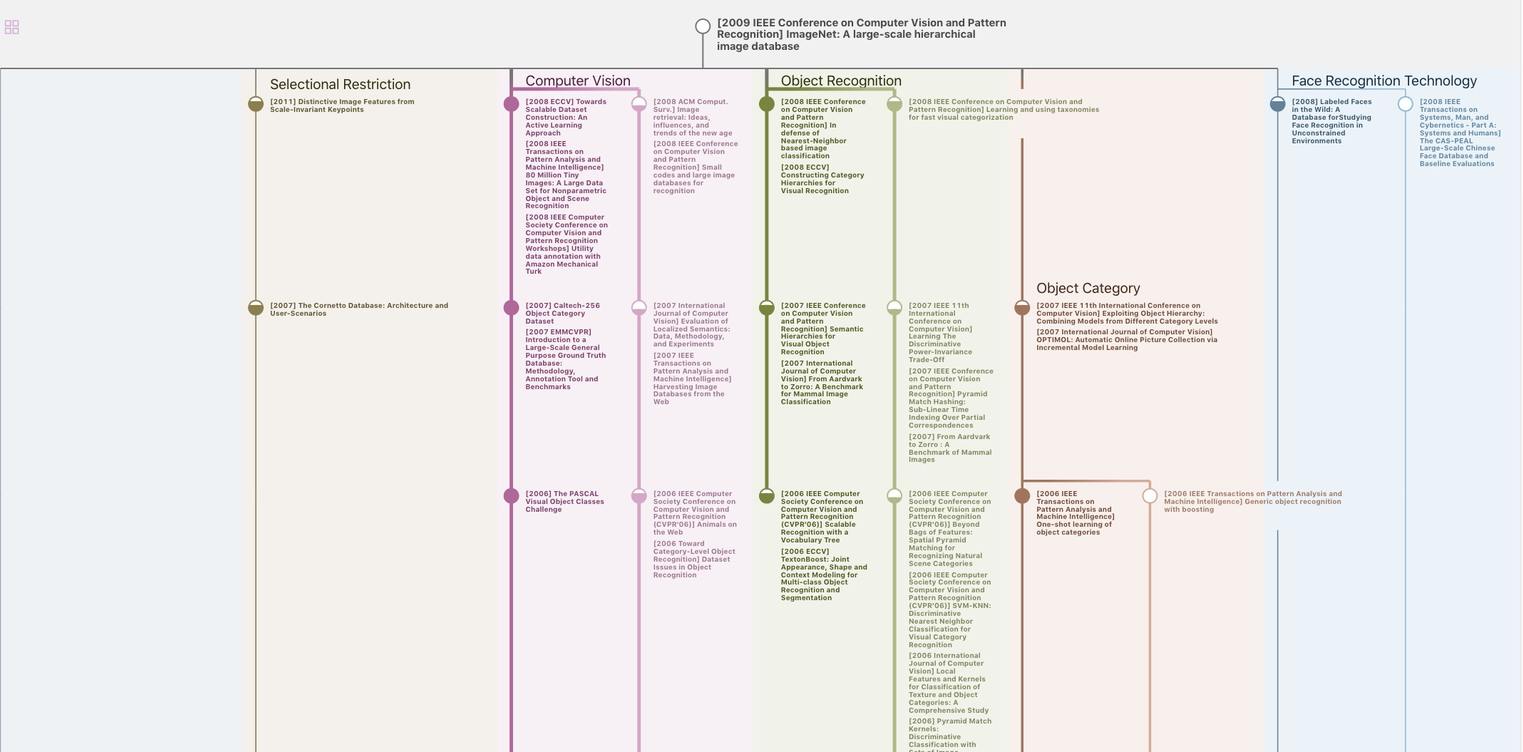
生成溯源树,研究论文发展脉络
Chat Paper
正在生成论文摘要