Detectability and image quality metrics based on robust statistics: following non-linear, noise-reduction filters
Proceedings of SPIE(2014)
摘要
Non-linear image processing and reconstruction algorithms that reduced noise while preserving edge detail are currently being evaluated in medical imaging research literature. We have implemented a robust statistics analysis of four widely utilized methods. This work demonstrates consistent trends in filter impact by which such non-linear algorithms can be evaluated. We calculate observer model test statistics and propose metrics based on measured non-Gaussian distributions that can serve as image quality measures analogous to SDNR and detectability. The filter algorithms that vary significantly in their approach to noise reduction include median (MD), bilateral (BL), anisotropic diffusion (AD) and total-variance regularization (TV). It is shown that the detectability of objects limited by Poisson noise is not significantly improved after filtration. There is no benefit to the fraction of correct responses in repeated n-alternate forced choice experiments, for n=2-25. Nonetheless, multi-pixel objects with contrast above the detectability threshold appear visually to benefit from non-linear processing algorithms. In such cases, calculations on highly repeated trials show increased separation of the object-level histogram from the background-level distribution. Increased conspicuity is objectively characterized by robust statistical measures of distribution separation.
更多查看译文
关键词
Noise-reduction,Non-Linear Filter,Dose,Observer Models
AI 理解论文
溯源树
样例
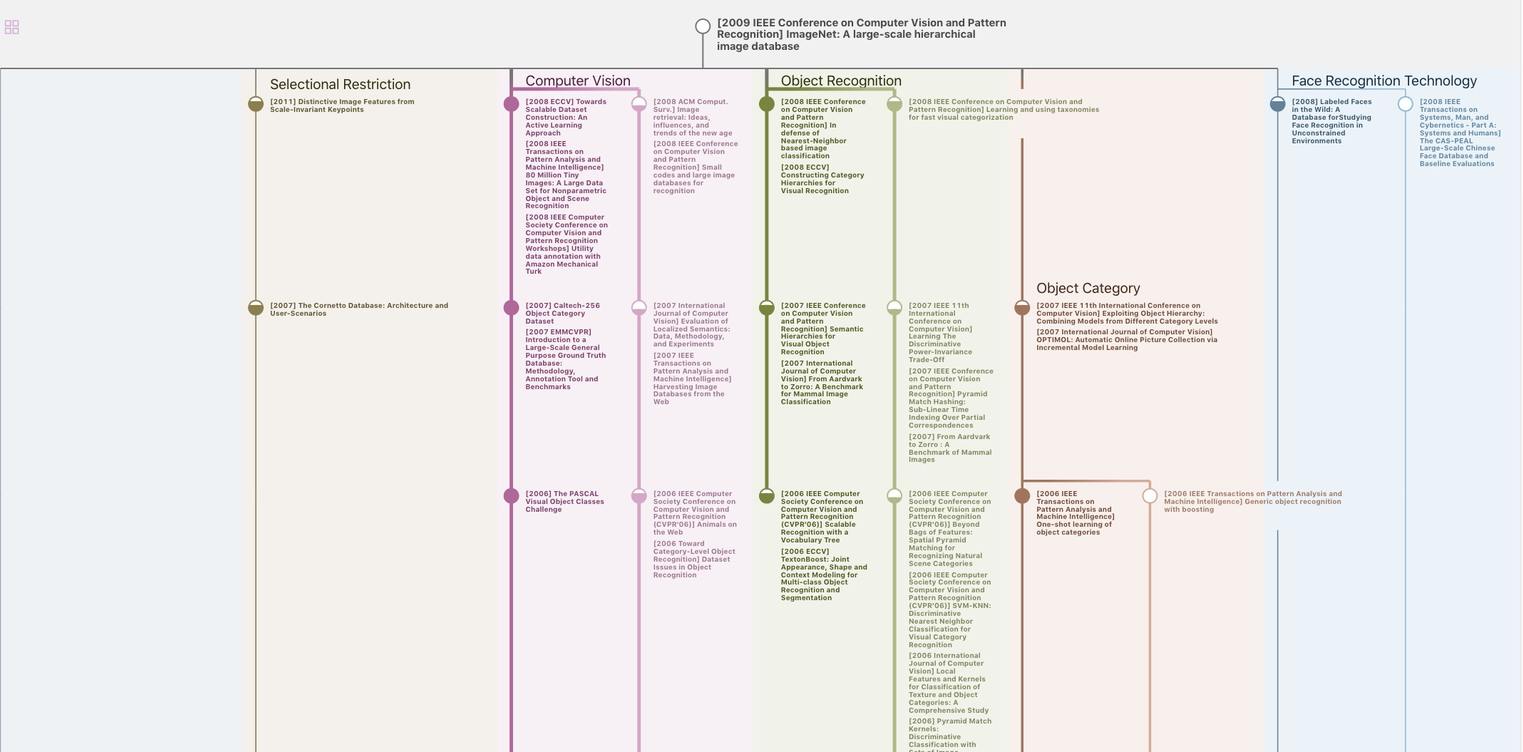
生成溯源树,研究论文发展脉络
Chat Paper
正在生成论文摘要