Nonlocal means-based regularizations for statistical CT reconstruction
Proceedings of SPIE(2014)
摘要
Statistical iterative reconstruction (SIR) methods have shown remarkable gains over the conventional filtered backprojection (FBP) method in improving image quality for low-dose computed tomography (CT). They reconstruct the CT images by maximizing/minimizing a cost function in a statistical sense, where the cost function usually consists of two terms: the data-fidelity term modeling the statistics of measured data, and the regularization term reflecting a prior information. The regularization term in SIR plays a critical role for successful image reconstruction, and an established family of regularizations is based on the Markov random field (MRF) model. Inspired by the success of non-local means (NLM) algorithm in image processing applications, we proposed, in this work, a family of generic and edge-preserving NLM-based regularizations for SIR. We evaluated one of them where the potential function takes the quadratic-form. Experimental results with both digital and physical phantoms clearly demonstrated that SIR with the proposed regularization can achieve more significant gains than SIR with the widely-used Gaussian MRF regularization and the conventional FBP method, in terms of image noise reduction and resolution preservation.
更多查看译文
关键词
CT reconstruction,shifted Poisson approximation,non-local means,regularizations
AI 理解论文
溯源树
样例
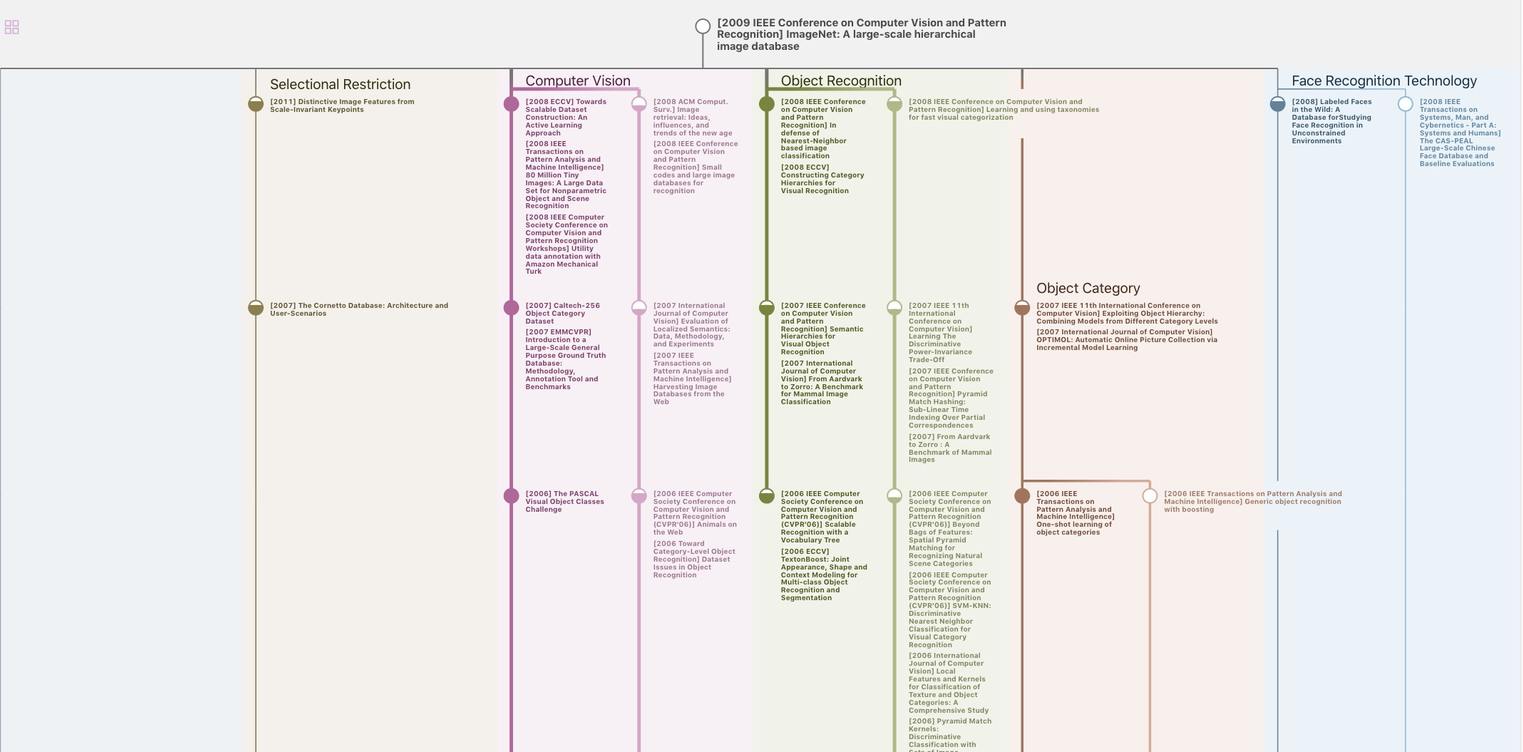
生成溯源树,研究论文发展脉络
Chat Paper
正在生成论文摘要