Speech Separation based on Deep Belief Network
mag(2015)
摘要
Thanks to its hierarchical and generative nature, Deep Belief Network (DBN) is effective to feature representation and extraction in signal processing. In this paper, DBN is investigated and implemented to monaural speech separation. Firstly, two separate DBNs are trained to extract features from mixed noisy signals and target clean speech respectively. Subsequently, the two types of extracted features are associated together by training a BP neural network to obtain a mapping from the features of mixed signals to the features of target speech. Finally, by performing DBN and the above mapping neural network, target speech can be estimated from the input mixed signals. Experiments are conducted on different kinds of mixed signals including female/male speech mixtures, human-speech/Gaussian-noise audio mixtures, and human-speech/music audio mixtures. The PESQ scores of the extracted speech are 3.32, 2.59, and 3.42 respectively, which illustrates that the model performs well on speech separation tasks, especially on the mixed signals where the inference signals have obvious spectral structures.
更多查看译文
关键词
restricted boltzmann machine,deep belief network,deep learning,autoencoder
AI 理解论文
溯源树
样例
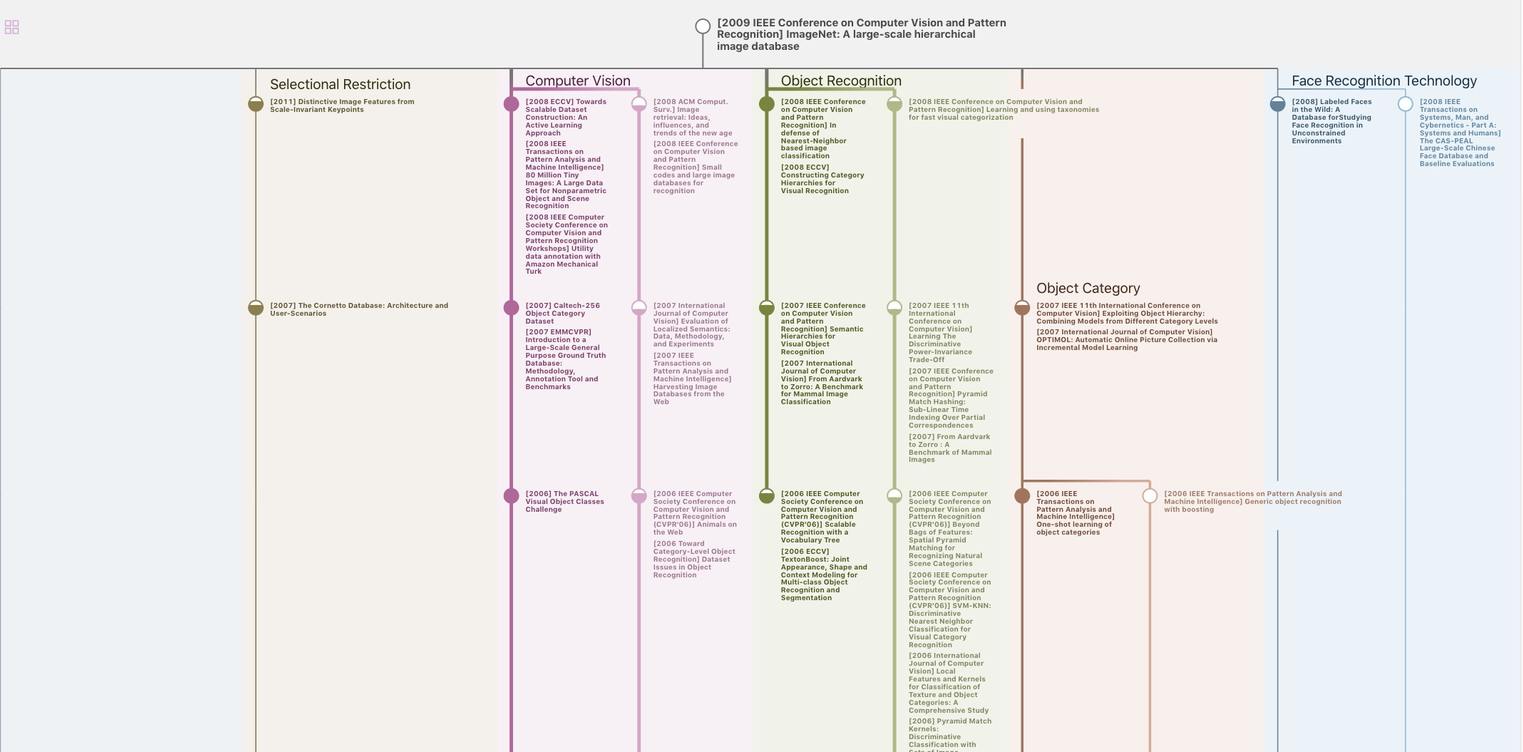
生成溯源树,研究论文发展脉络
Chat Paper
正在生成论文摘要