Computer-aided classification of lesions by means of their kinetic signatures in dynamic contrast-enhanced MR images
PROCEEDINGS OF THE SOCIETY OF PHOTO-OPTICAL INSTRUMENTATION ENGINEERS (SPIE)(2008)
摘要
The kinetic characteristics of tissue in dynamic contrast-enhanced magnetic resonance imaging data are an important source of information for the differentiation of benign and malignant lesions. Kinetic curves measured for each lesion voxel allow to infer information about the state of the local tissue. As a whole, they reflect the heterogeneity of the vascular structure within a lesion, an important criterion for the preoperative classification of lesions. Current clinical practice in analysis of tissue kinetics however is mainly based on the evaluation of the "most-suspect curve", which is only related to a small, manually or semi-automatically selected region-of-interest within a lesion and does not reflect any information about tissue heterogeneity. We propose a new method which exploits the full range of kinetic information for the automatic classification of lesions. Instead of breaking down the large amount of kinetic information to a single curve, each lesion is considered as a probability distribution in a space of kinetic features, efficiently represented by its kinetic signature obtained by adaptive vector quantization of the corresponding kinetic curves. Dissimilarity of two signatures can be objectively measured using the Mallows distance, which is a metric defined on probability distributions. The embedding of this metric in a suitable kernel function enables us to employ modern kernel-based machine learning techniques for the classification of signatures. In a study considering 81 breast lesions, the proposed method yielded an A(z) value of 0.89 +/- 0.01 for the discrimination of benign and malignant lesions in a nested leave-one-lesion-out evaluation setting.
更多查看译文
关键词
magnetic resonance imaging,tumor,breast,classification,kinetic,signatures,heterogeneity,histogram
AI 理解论文
溯源树
样例
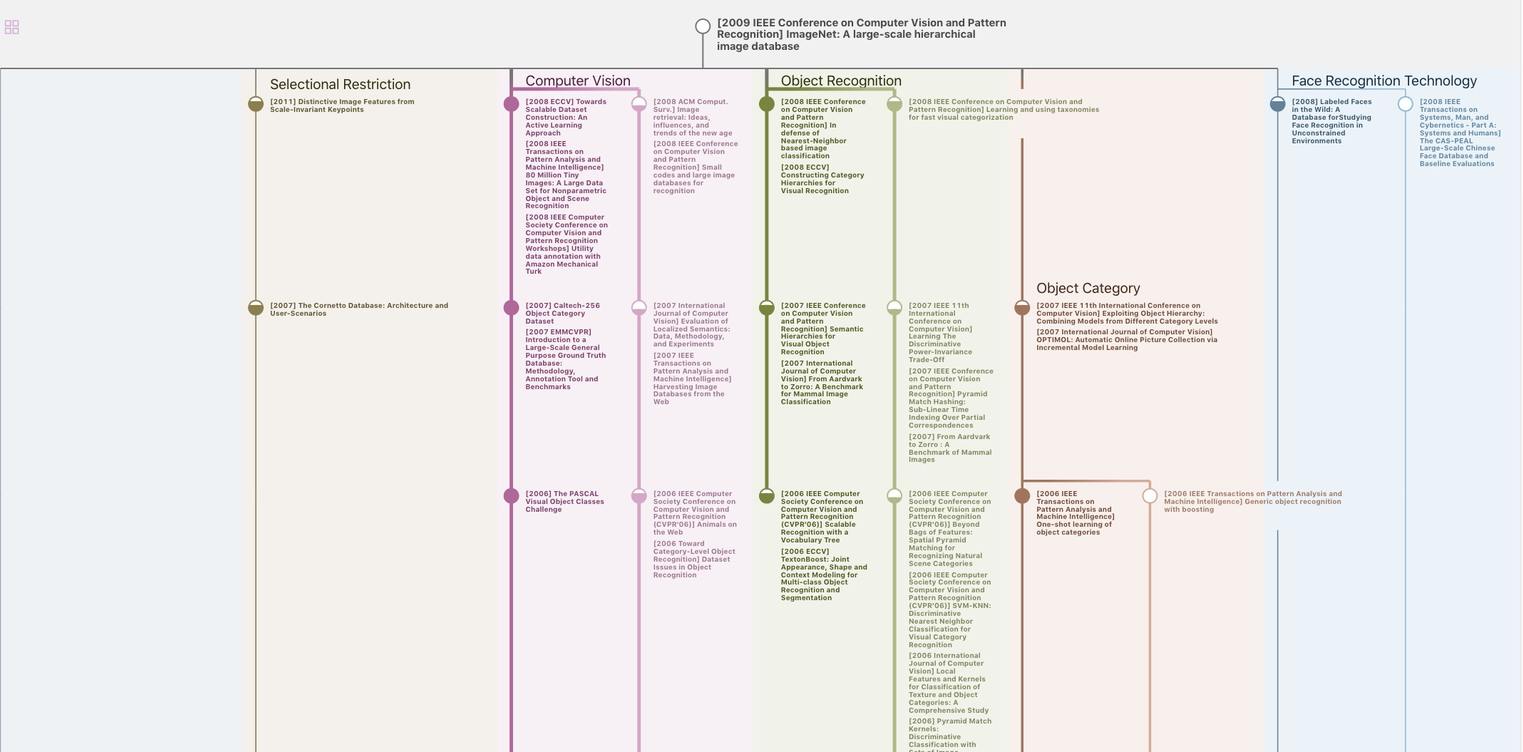
生成溯源树,研究论文发展脉络
Chat Paper
正在生成论文摘要