Large-Scale Online Expectation Maximization with Spark Streaming
mag(2013)
摘要
Many “Big Data” applications in Machine Learning (ML) need to react quickly to large streams of incoming data. The standard paradigm nowadays is to run ML algorithms on frameworks designed for batch operations, such as MapReduce or Hadoop. By design, these frameworks are not a good match for low-latency applications. This is why we explore using a new, recently proposed model for large-scale stream processing, discretized streams (D-Streams [19]), for online Machine Learning. Our application is an online Expectation-maximization algorithm, that estimates the state of car traffic in the San Francisco Bay Area. Using D-Streams, we are able to achieve near-perfect scaling of our application on a commodity cluster in a reliable, fault-tolerant way. Our algorithm can update the state of traffic from hundreds of thousand of GPS observations within a few seconds.
更多查看译文
AI 理解论文
溯源树
样例
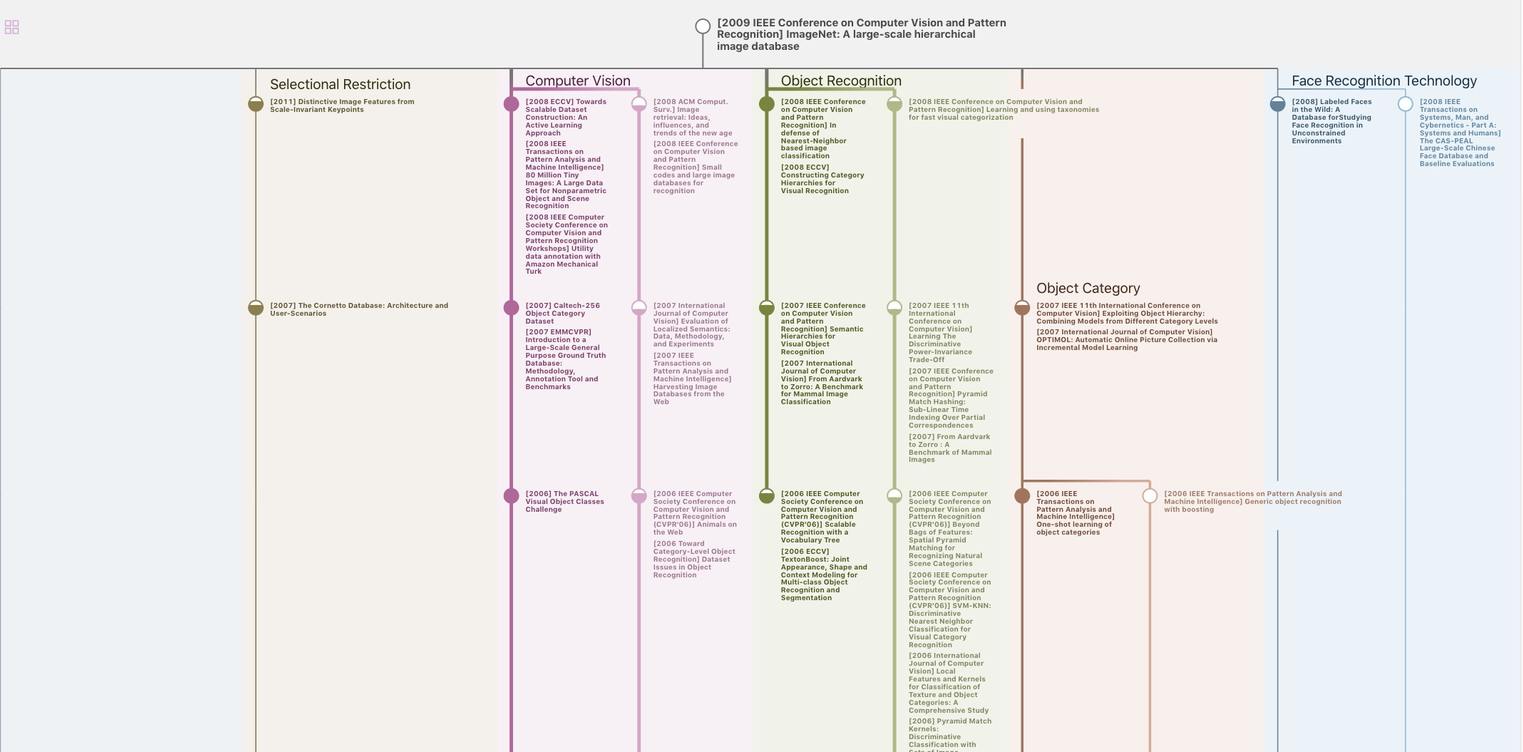
生成溯源树,研究论文发展脉络
Chat Paper
正在生成论文摘要