Multi-label Object Categorization Using Histograms of Global Relations
3DV '15 Proceedings of the 2015 International Conference on 3D Vision(2015)
摘要
In this paper, we present an object categorization system capable of assigning multiple and related categories for novel objects using multi-label learning. In this system, objects are described using global geometric relations of 3D features. We propose using the Joint SVM method for learning and we investigate the extraction of hierarchical clusters as a higher-level description of objects to assist the learning. We make comparisons with other multi-label learning approaches as well as single-label approaches (including a state-of-the-art methods using different object descriptors). The experiments are carried out on a dataset of 100 objects belonging to 13 visual and action-related categories. The results indicate that multi-label methods are able to identify the relation between the dependent categories and hence perform categorization accordingly. It is also found that extracting hierarchical clusters does not lead to gain in the system's performance. The results also show that using histograms of global relations to describe objects leads to fast learning in terms of the number of samples required for training.
更多查看译文
关键词
histograms,feature extraction,encoding,visualization,support vector machines
AI 理解论文
溯源树
样例
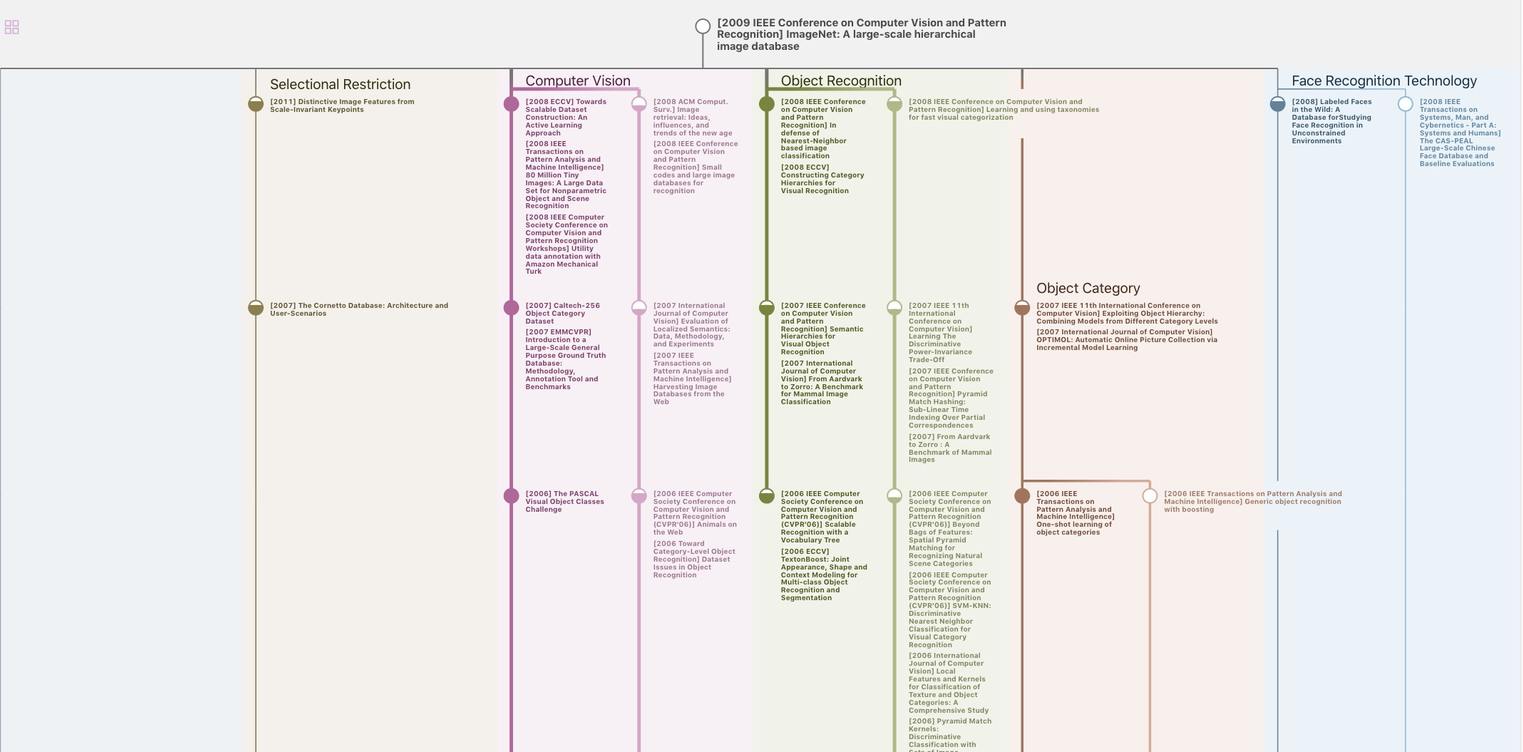
生成溯源树,研究论文发展脉络
Chat Paper
正在生成论文摘要