Multi-View Forest: A New Ensemble Method Based On Dempster-Shafer Evidence Theory
INTERNATIONAL JOURNAL OF APPLIED MATHEMATICS & STATISTICS(2011)
摘要
This paper proposes a new ensemble method that constructs an ensemble of tree-structured classifiers using multi-view learning. We are motivated by the fact that an ensemble can outperform its members providing that these classifiers are diverse and accurate. In order to construct diverse individual classifiers, we assume that the object to be classified is described by multiple feature sets (views). We aim to construct different tree classifiers using different views to improve the accuracy of the multi-class recognition task. For the decision fusion of the binary classifiers within each tree classifier, Dempster's unnormalized rule of combination is applied and an evidence-theoretic decision profile is proposed to combine the decisions of different trees. Numerical experiments have been performed on two real-world data sets: a data set of handwritten digits, and another data set of 3D visual objects. The results indicate that the proposed forest efficiently integrates multi-view data and outperforms the individual tree classifiers.
更多查看译文
关键词
Dempster-Shafer evidence theory, pattern recognition, multi-view learning, radial basis function neural networks, multi-class decomposition
AI 理解论文
溯源树
样例
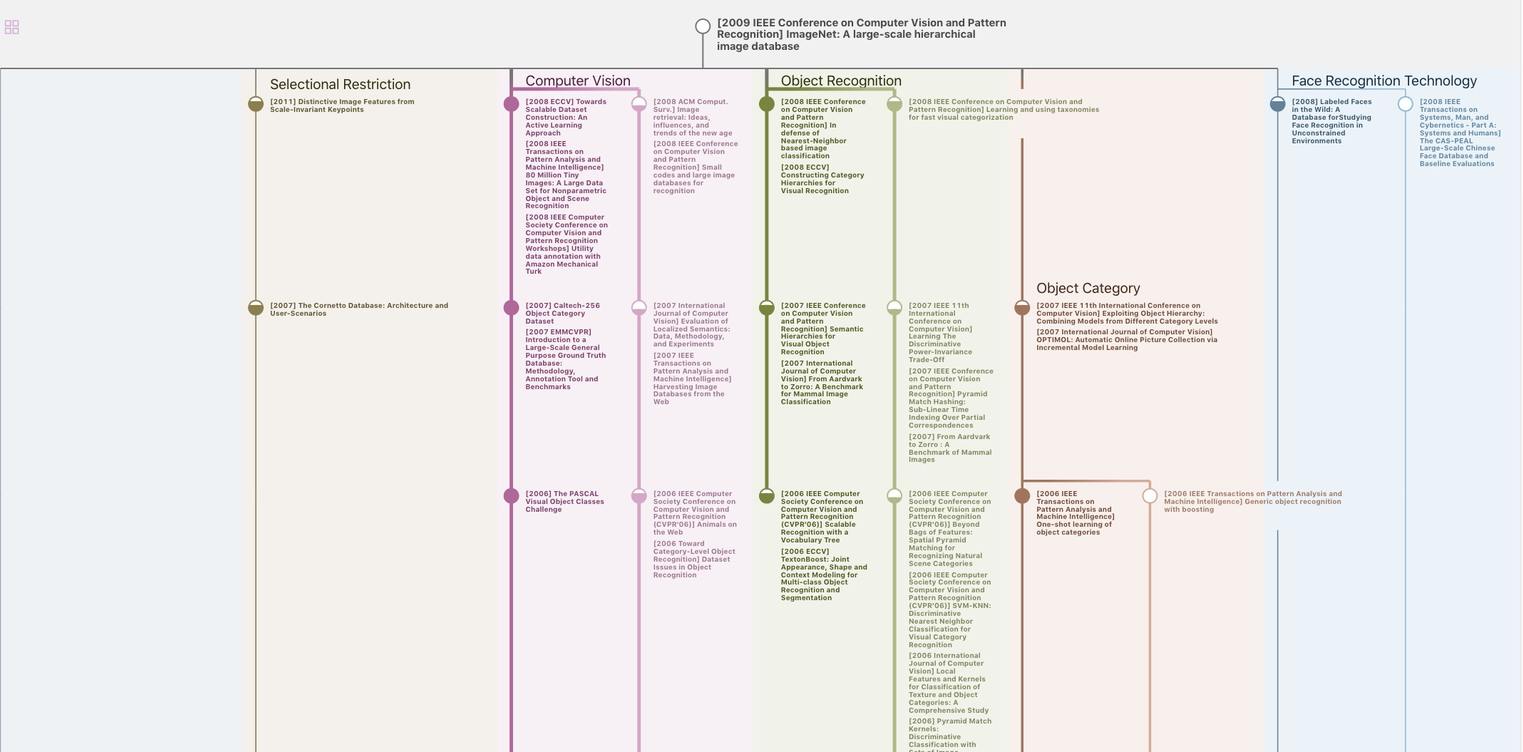
生成溯源树,研究论文发展脉络
Chat Paper
正在生成论文摘要