Utjecaj relevantnosti konteksta na predviđanje ocjena u sustavu za preporuke filmova
AUTOMATIKA(2013)
摘要
Recommender systems are a popular and a highly researched way of helping users get to their desired content in the huge amount of available data, and services online. Understanding the situation in which users consume the items was shown to improve the recommendation process. For that reason, context-aware recommender system (CARS) employs contextual information in order to enhance the user's model and to improve the recommendations. An issue that is still open is how to decide which pieces of contextual information to acquire and how to incorporate them into CARS, since using irrelevant piece of contextual information could have a negative impact on the recommendations. We propose a methodology for detecting which pieces of contextual information contribute to explaining the variance in the ratings, based on statistical testing. We also inspect the impact of the detected relevant pieces of contextual information on the ratings prediction based on the matrix-factorization algorithm. The experiment was conducted on the MovieAT database. The results showed a significant difference in the ratings prediction using the relevant and the irrelevant pieces of contextual information. We also confirmed the positive impact of the relevant, and negative impact of the irrelevant pieces of contextual information with respect to the uncontextualized model.
更多查看译文
关键词
Personalization,Recommender systems,Context-awareness
AI 理解论文
溯源树
样例
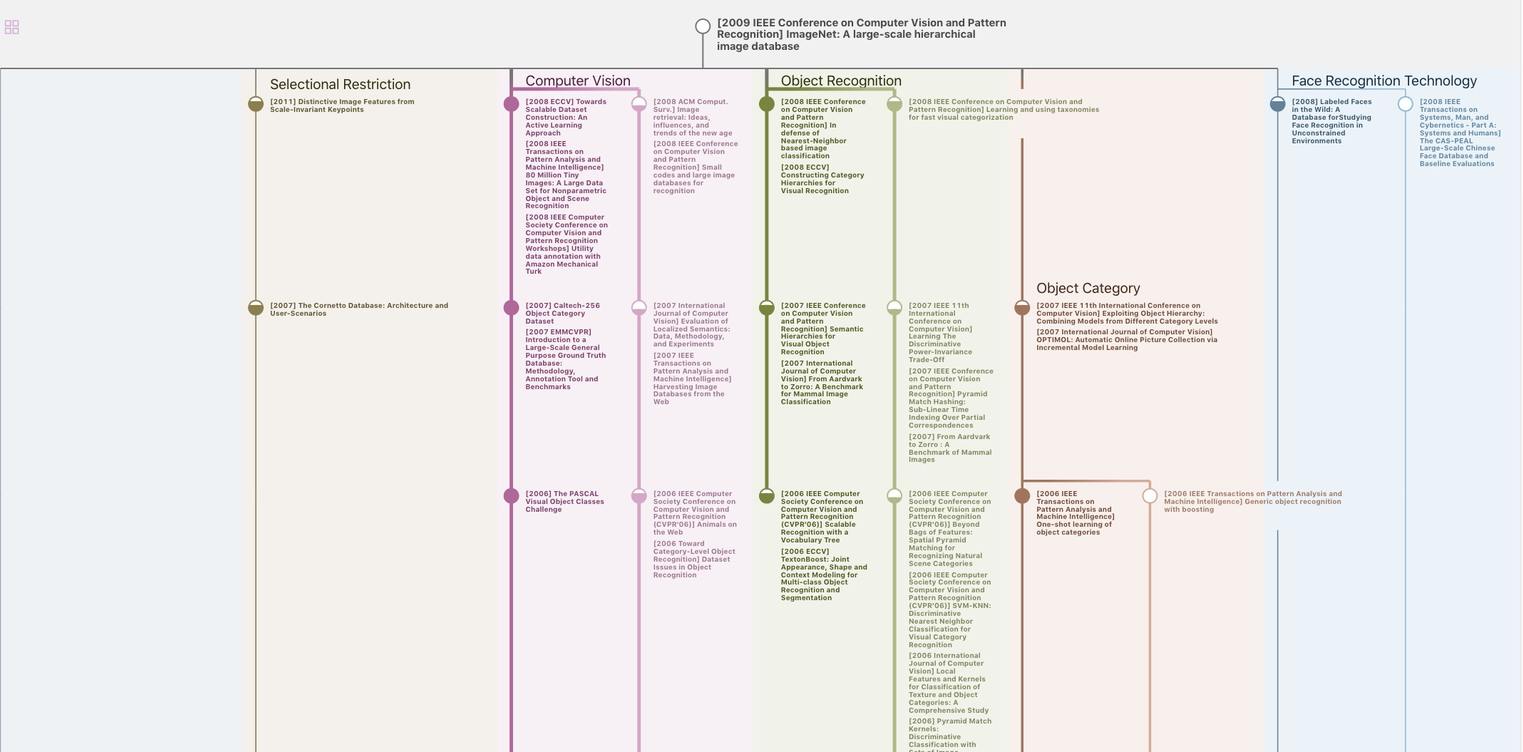
生成溯源树,研究论文发展脉络
Chat Paper
正在生成论文摘要