Differentially Private Multi-party Computation: Optimality of Non-Interactive Randomized Response
mag(2014)
摘要
We study the problem of interactive function computation by multiple parties possessing a single bit each in a differential privacy setting (i.e., there remains an uncertainty in any specific party's bit even when given the transcript of the interactions and all the other parties' bits). Each party is interested in computing a function, which could differ from party to party, and there could be a central observer interested in computing a separate function. Performance at each party and the central observer is measured via the accuracy of the function computed. We allow for an arbitrary cost function to measure the distortion between the true and the computed function value. Our main result is the exact optimality of a simple non-interactive protocol: each party randomizes (sufficiently) and publishes its own bit. In other words, non-interactive randomized response is exactly optimal. Each party and the central observer then separately compute their respective function to maximize the appropriate notion of their accuracy measure. The optimality is very general: it holds for all types of functions, heterogeneous privacy conditions on the parties, all types of cost metrics, and both average and worst-case (over the inputs) measures of accuracy. Finally, the optimality result is simultaneous, in terms of maximizing accuracy at each of the parties and the central observer.
更多查看译文
AI 理解论文
溯源树
样例
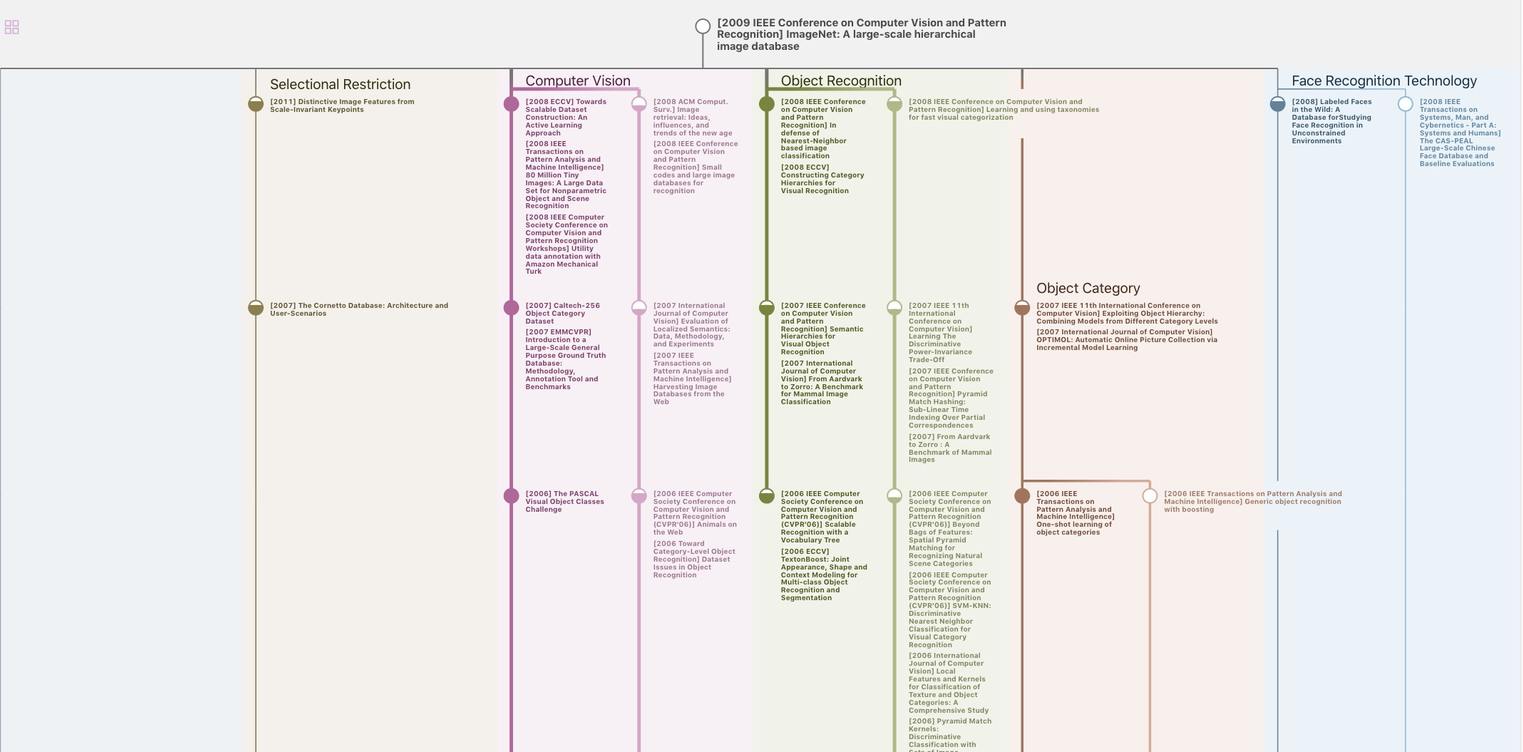
生成溯源树,研究论文发展脉络
Chat Paper
正在生成论文摘要