Adaptive importance sampling via minimization of estimators of cross-entropy, mean square, and inefficiency constant
mag(2015)
摘要
The inefficiency of using an unbiased estimator in a Monte Carlo procedure can be quantified using an inefficiency constant, equal to the product of the variance of the estimator and its mean computational cost. We develop methods for obtaining the parameters of the importance sampling (IS) change of measure via single- and multi-stage minimization of well-known estimators of cross-entropy and the mean square of the IS estimator, as well as of new estimators of such a mean square and inefficiency constant. We prove the convergence and asymptotic properties of the minimization results in our methods. We show that if a zero-variance IS parameter exists, then, under appropriate assumptions, minimization results of the new estimators converge to such a parameter at a faster rate than such results of the well-known estimators, and a positive definite asymptotic covariance matrix of the minimization results of the cross-entropy estimators is four times such a matrix for the well-known mean square estimators. We introduce criteria for comparing the asymptotic efficiency of stochastic optimization methods, applicable to the minimization methods of estimators considered in this work. In our numerical experiments for computing expectations of functionals of an Euler scheme, the minimization of the new estimators led to the lowest inefficiency constants and variances of the IS estimators, followed by the minimization of the well-known mean square estimators, and the cross-entropy ones.
更多查看译文
AI 理解论文
溯源树
样例
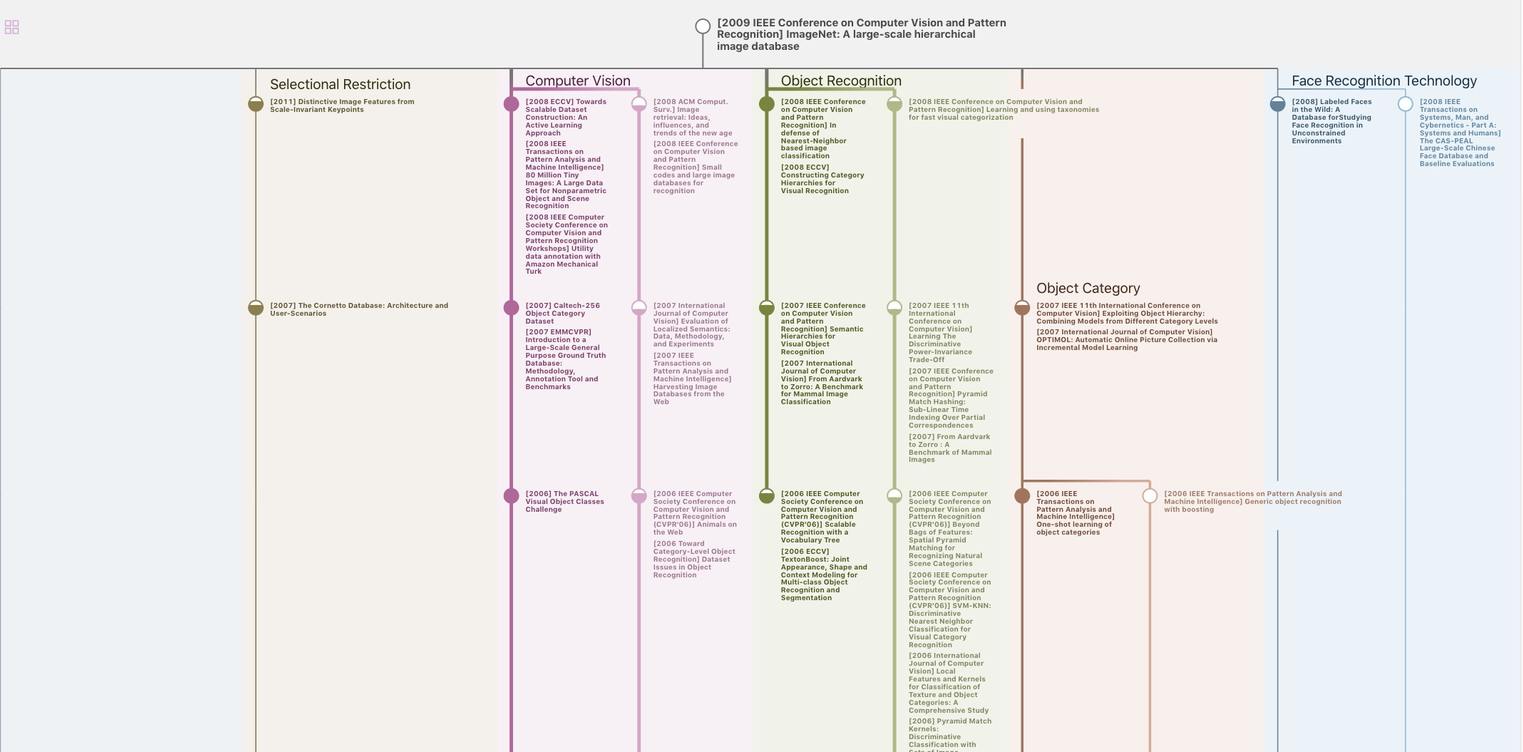
生成溯源树,研究论文发展脉络
Chat Paper
正在生成论文摘要