An Automatic And Cost-Effective Parasitemia Identification Framework For Low-End Microscopy Imaging Devices
2014 INTERNATIONAL CONFERENCE ON MECHATRONICS AND CONTROL (ICMC)(2014)
摘要
In the detection of Malarial parasites from a patient, it is usually necessary to carefully examine the corresponding blood-slide smear and distinguish the infected and healthy Red Blood Cells (RBCs). If this process is done manually, as evidenced in common traditional approaches, the following challenges may be encountered: inaccuracy of the lab results, which originates from normal human errors or lack of experience of a person conducting diagnosis, and large processing times. Consequently, doctors and specialists are likely to provide improper prescriptions to patients. With the improvement of the computational power of computers, however, the whole diagnosis process can be automated. Several methods in literature have been proposed for this purpose. Most of these methods demand the availability of high-end microscopy imaging systems to generate reliable and accurate results. Such costly advanced devices may not be afforded by developing countries with sluggish economic growth. In this paper, therefore, we have developed a cost-effective framework which can address the mentioned challenge. Our approach introduces a Super Resolution (SR) model into the existing framework to enhance the resolution of the input images before letting them subjected to the subsequent detection stages. This provides a possibility for applying the low-end microscopy devices capable of capturing Low Resolution (LR) blood smear images for identifying the degree of Malaria in a patient. In the proposed framework, the SR component uses the nonlinear Charbonnier diffusion model in the regularization part because of its good regularity characteristics. Experimental results demonstrate strong correlation of our method and the manual one.
更多查看译文
关键词
Malaria, SuperResolution, Parasitemia, Reconstruction, Regularization
AI 理解论文
溯源树
样例
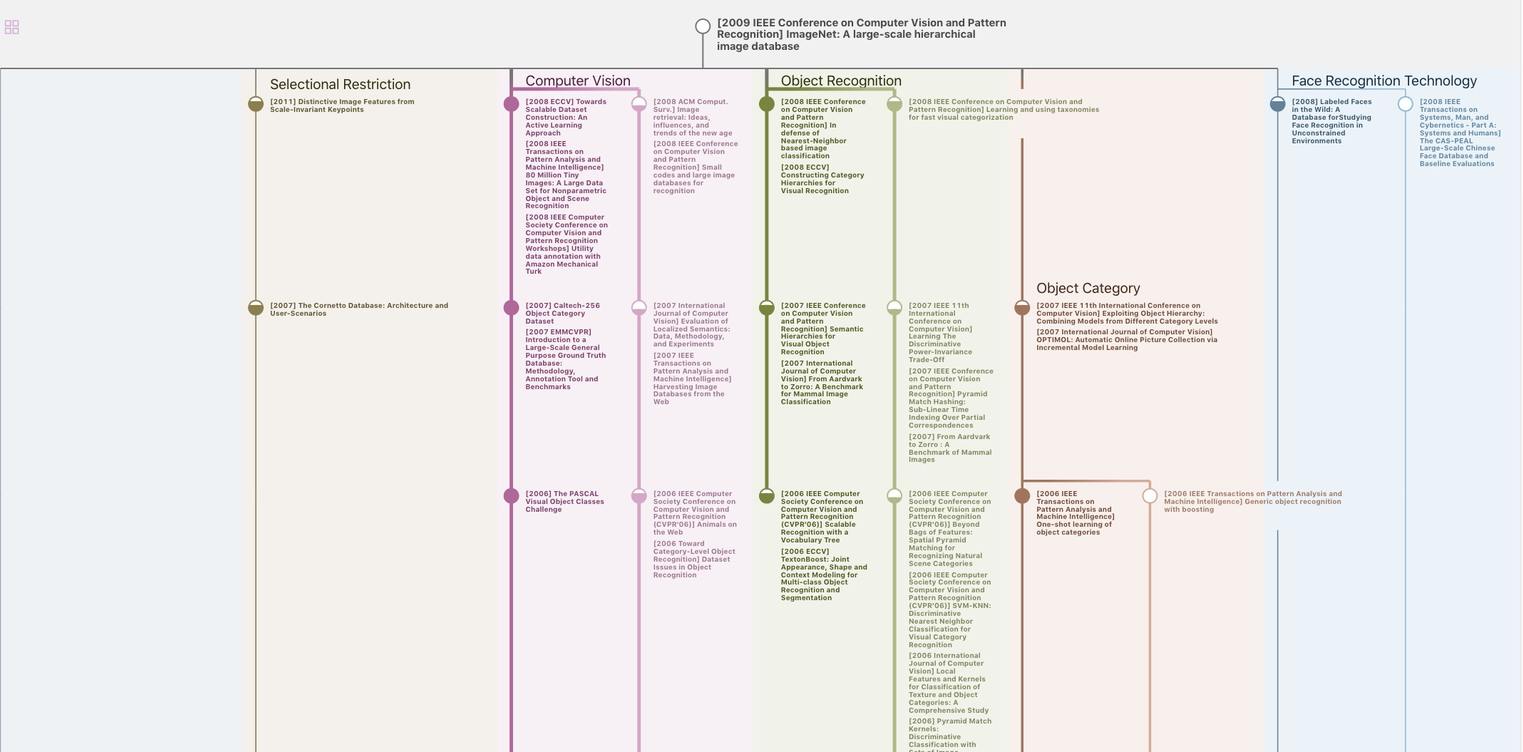
生成溯源树,研究论文发展脉络
Chat Paper
正在生成论文摘要