Applications of Hidden Markov Models in Microarray Gene Expression Data
Hidden Markov Models, Theory and Applications(2011)
摘要
Hidden Markov models (HMMs) are well developed statistical models to capture hidden information from observable sequential symbols. They were first used in speech recognition in 1970s and have been successfully applied to the analysis of biological sequences since late 1980s as in finding protein secondary structure, CpG islands and families of related DNA or protein sequences [1]. In a HMM, the system being modeled is assumed to be a Markov process with unknown parameters, and the challenge is to determine the hidden parameters from the observable parameters. In this chapter, we described two applications using HMMs to predict gene functions in yeast and DNA copy number alternations in human tumor cells, based on gene expression microarray data. The first application employed HMMs as a gene function prediction tool to infer budding yeast Saccharomyces cerevisiae gene function from time-series microarray gene expression data. The sequential observations in HMM were the discretized expression measurements at each time point for the genes from the time-series microarray experiments. Yeast is an excellent model organism which has reasonably simple genome structure, well characterized gene functions, and huge expression data sets. A wide variety of data mining methods have been applied for inferring yeast gene functions from gene expression data sets, such as Decision Tree, Artificial Neural Networks, Support Vector Machines (SVMs) and K-Nearest Neighbors (KNNs) [2-4]. However those methods achieved only about 40% prediction precision in function prediction of un-annotated genes [2-4]. Based on our observations, there are three main reasons for the low prediction performance. First, the computational models are too simple to address the systematic variations of biological systems. One assumption is that genes from the same function class will show a similar expression pattern. However, clustering results have shown that functions and clusters have many-to-many relationship and it is often difficult to assign a function to an expression pattern (Eisen et al., supplementary data) [5]. Second, the measurements of expression value are generally not very accurate and show experimental errors (or noise). The observed expression values may not reflect the real expression levels of genes. For example, a correlation as low as 60% was reported between measurements of the same sample hybridized to two slides [6]. Third, none of the above methods explicitly address the less obvious but significant correlation of gene expressions. Our results indicate that the expression value of a gene depends significantly on its previous expression value. Therefore, Markov property can be assumed to simplify the non-independence of gene
更多查看译文
AI 理解论文
溯源树
样例
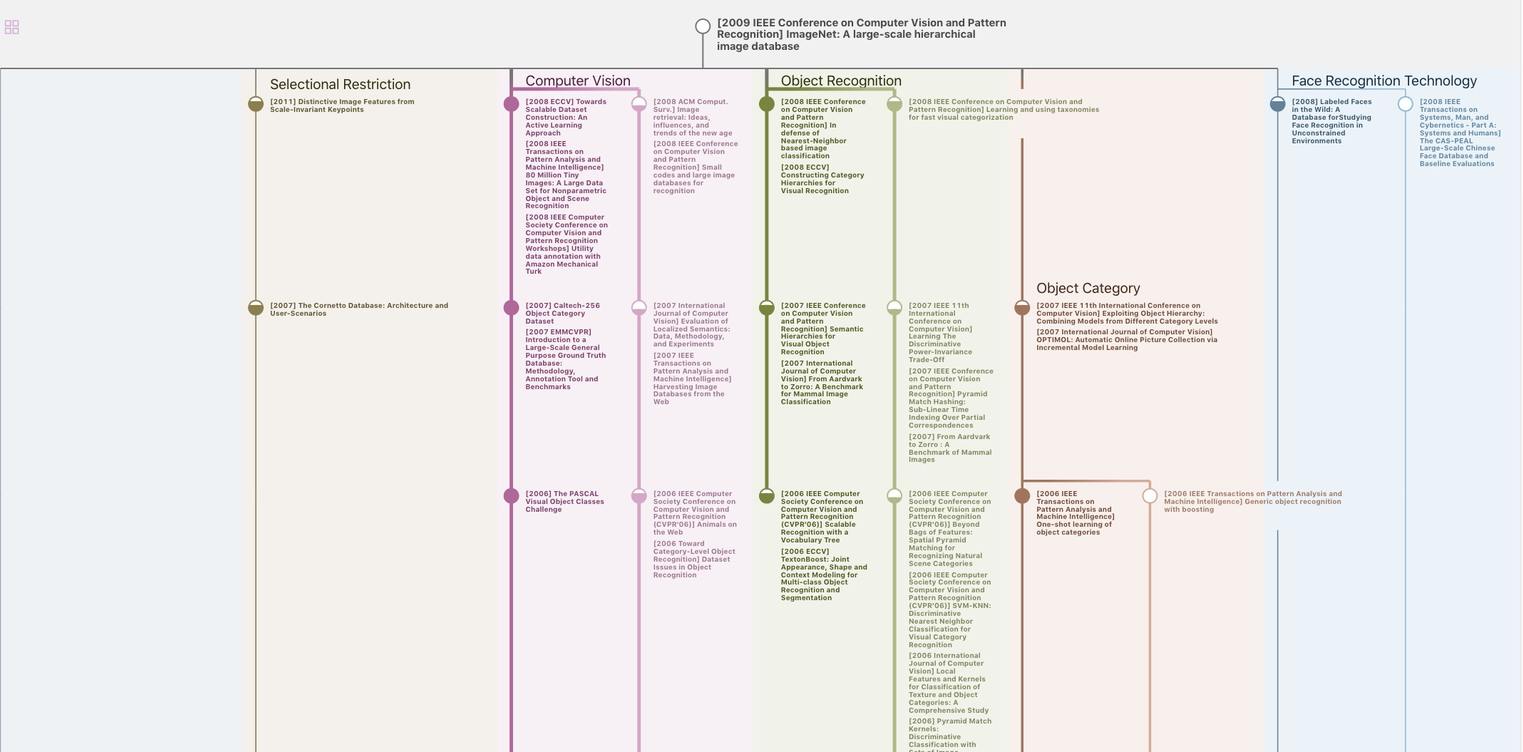
生成溯源树,研究论文发展脉络
Chat Paper
正在生成论文摘要