Decision tree induction for detection of clinical mastitis using data from six Dutch dairy herds milking with an automatic milking system.
MASTITIS CONTROL: FROM SCIENCE TO PRACTICE(2008)
摘要
This study explored the potential of decision tree induction for the automated detection of clinical mastitis. Sensor data (including electrical conductivity, colour and yield) of over 670,000 quarter milkings were collected from December 2006 till August 2007 at six dairy herds using automatic milking. Farmers recordings of quarter milkings that showed clear clinical signs of mastitis were considered as gold standard positive cases (n=100). Quarter milkings that were not visually checked, that were checked but scored as being normal, and that were outside a 2-week range before or after a gold standard positive case were used as gold standard negative cases. A random sample of 3,000 gold standard negative cases was selected for further analyses. Using 10-fold cross validation, a decision tree algorithm was implemented to estimate the probability of having clinical mastitis for each quarter milking. Parameter settings of the decision tree algorithm were varied to study the effect on sensitivity, specificity, the False Alert rate and the Success Rate. Receiver-operating characteristic curves were constructed to visualise all potential combinations of sensitivity and specificity of different detection models. Although data from the field was used, the rather simple algorithms and the limited time window used for the models to account an alert as true positive, results suggested that decision tree induction has potential for detecting clinical mastitis using sensor data. The default decision tree showed higher sensitivity values for a specificity range of 97 to 100% compared to two alternative trees. By changing the settings it was possible to reach higher levels of sensitivity for specificity values lower than 97%.
更多查看译文
关键词
automatic milking,clinical mastitis,data mining,diagnosis
AI 理解论文
溯源树
样例
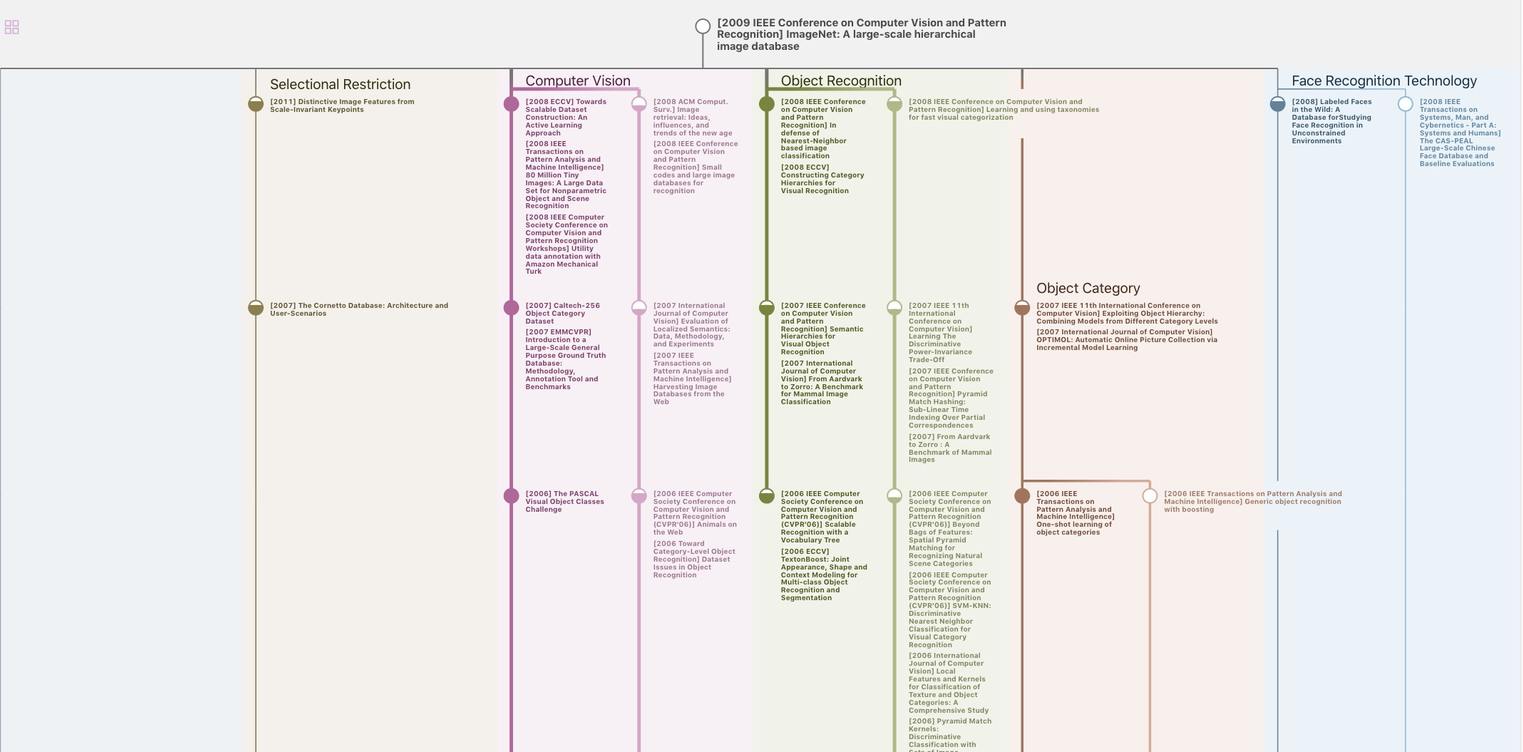
生成溯源树,研究论文发展脉络
Chat Paper
正在生成论文摘要