A Learning-Based Approach For Automatic Defect Detection In Textile Images
IFAC PAPERSONLINE(2015)
摘要
This paper addresses the textile defect detection problem using a machine-learning approach. We propose a novel algorithm that uses supervised learning to classify textile textures in defect and non-defect classes based on suitable feature extraction and classification. We use statistical modeling of multi-scale contourlet image decomposition to obtain compact and accurate signatures for texture description. Our defect detection algorithm is based on two phases. In the first phase, using a training set of images, we extract reference defect-free signatures for each textile category. Then, we use the Bayes classifier (BC) to learn signatures of defected and non-defected classes. In the second phase, defects are detected on new images using the trained BC and an appropriate decomposition of images into blocks. Our algorithm has the capability to achieve highly accurate defect detection and localisation in textile textures while ensuring an efficient computational time. Compared to recent state-of-the-art methods, our algorithm has yielded better results on the standard TILDA database. (C) 2015, lFAC (International Federation of Automatic Control) Hosting by Elsevier Ltd. All rights reserved.
更多查看译文
关键词
Textile defect detection, mixtures of generalized Gaussians (MoGG), contourlets, Bayes classifier
AI 理解论文
溯源树
样例
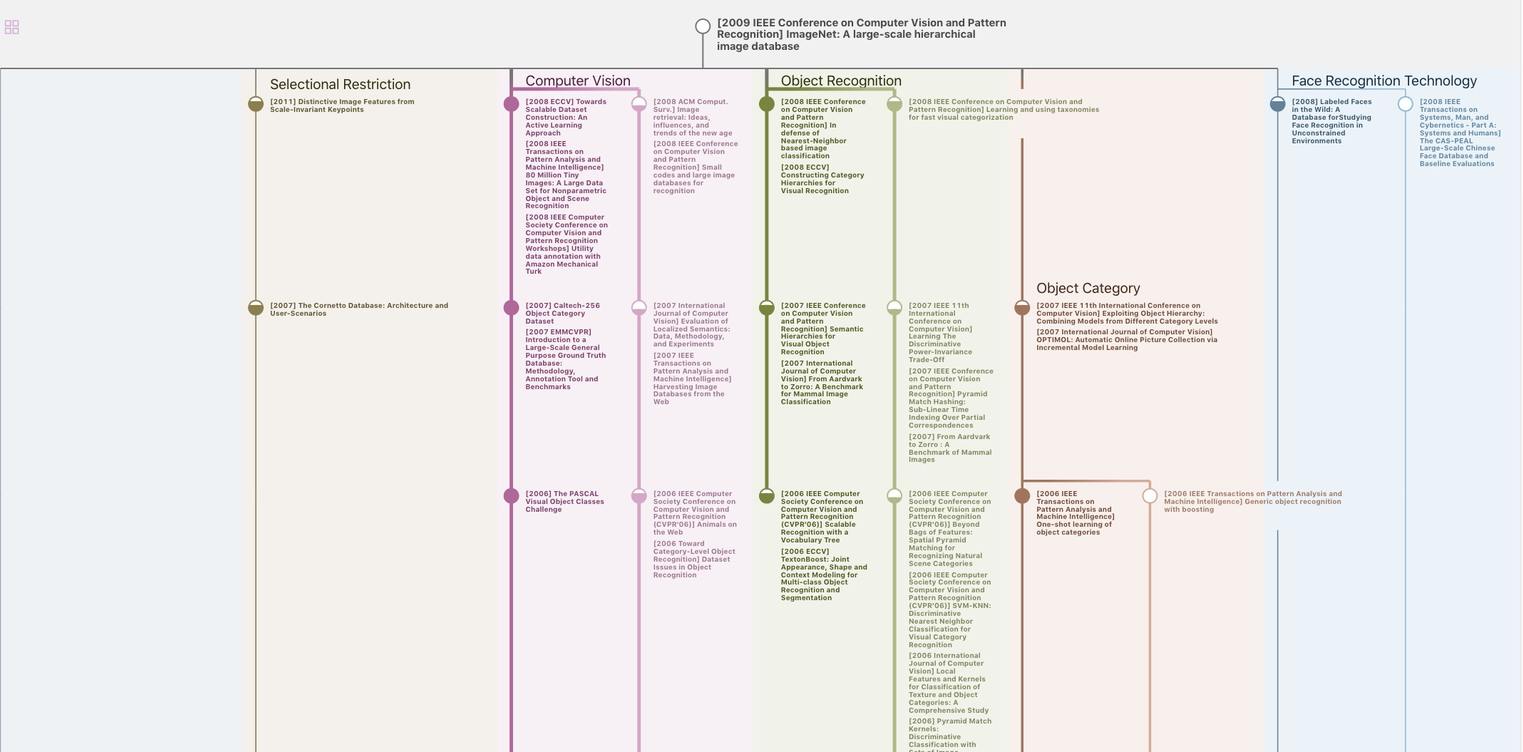
生成溯源树,研究论文发展脉络
Chat Paper
正在生成论文摘要