Uncertainty Characterization Using Copulas For Classification
MULTISENSOR, MULTISOURCE INFORMATION FUSION: ARCHITECTURES, ALGORITHMS, AND APPLICATIONS 2015(2015)
摘要
We address the problem of characterizing uncertainty for multisensor data fusion in a classification problem. To achieve this goal, we model the joint density of given multivariate data using copula functions while allowing the ability to incorporate any desired marginal distributions, i.e., any desired modalities. The proposed model is data driven in that the corresponding copula functions and their parameters are learned from the data. Our results show that the proposed framework can capture the uncertainties more accurately than current state of the practice, and lead to robust and improved classification performance compared to traditional classifiers.
更多查看译文
关键词
Multisource data fusion, copula theory, classification
AI 理解论文
溯源树
样例
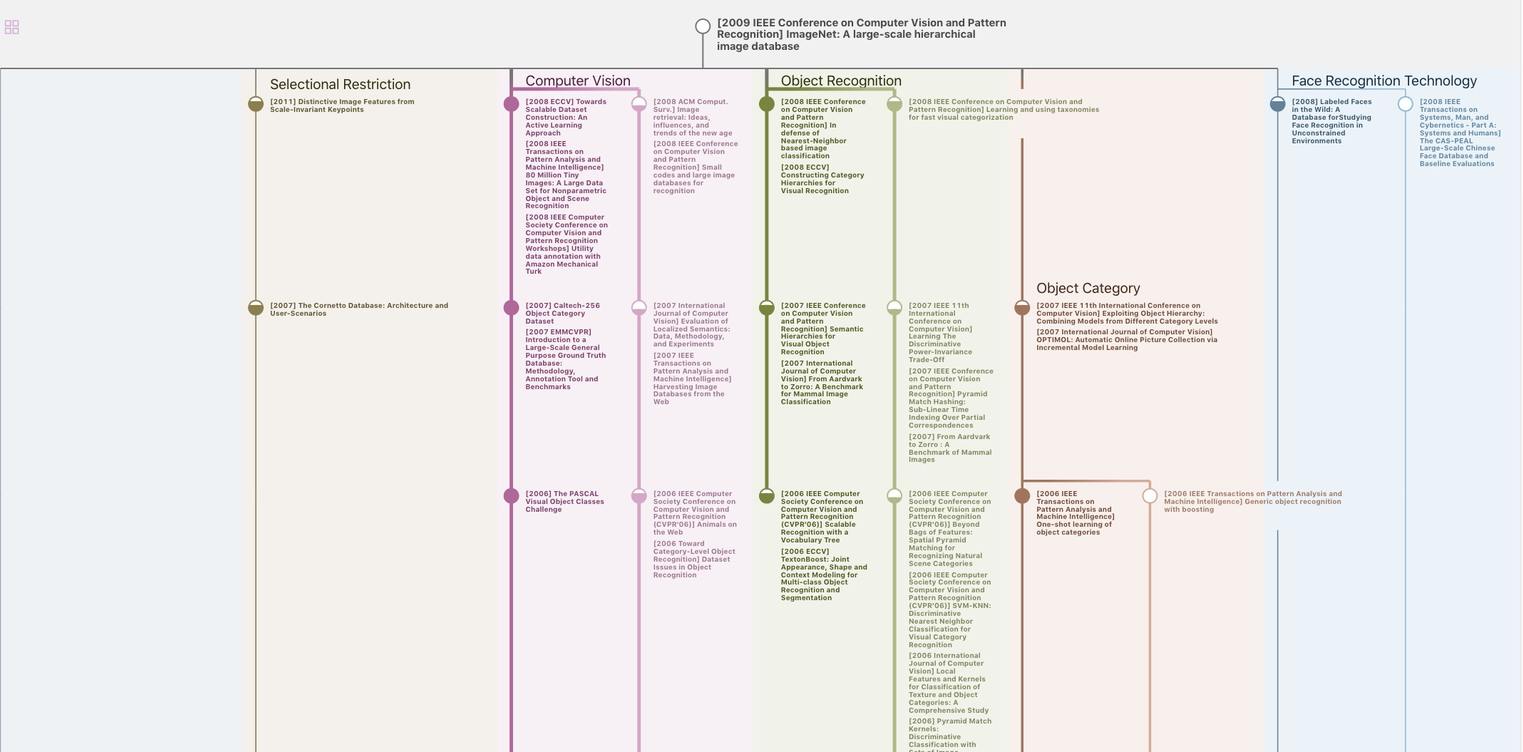
生成溯源树,研究论文发展脉络
Chat Paper
正在生成论文摘要