A Novel Ontology and Machine Learning Inspired Hybrid Cardiovascular Decision Support Framework
SSCI(2015)
摘要
Healthcare information management systems (HIMS) have a substantial amount of limitations such as rigidity and nonconformity to complex clinical processes like Electronic Healthcare records and effective utilisation of clinical practice guidelines to help provide effective clinical decision support. The conventional healthcare systems suffer from a general lack of intelligence, they are successful in offering basic patient management capabilities, but they do not offer consistent and holistic decision support capabilities for clinicians working under tight deadlines in a fast paced environment. The conventional healthcare information management systems are designed using branching logic based rigid architectures, which are hard to maintain and upgrade without considerable labour intensive effort. The proposed ontology and machine learning driven hybrid clinical decision support framework comprises of two key components (1) ontology driven clinical risk assessment and recommendation system and (2) machine learning driven prognostic system. The key aim of our research is to utilise information collected through the knowledge based ontology driven clinical risk assessment and recommendation system and non-knowledge based/evidence based machine learning driven prognostic system to deliver a holistic clinical decision support framework in the cardiovascular domain. The ontology driven clinical risk assessment and recommendation system could be used as a triage system for cardiovascular patients as a preventative solution, this could help clinicians prioritise patient referrals after reviewing a snapshot of patient's medical history (collected through an ontology driven intelligent context aware information collection using standardised clinical questionnaires) containing patient demographics information, cardiac risk scores, cardiac chest pain score, medication and recommended lab tests details. The machine learning driven prognostic system is developed using a chest pain clinical case study identified by the consultant cardiologist, Professor Stephen Leslie from Raigmore Hospital in Inverness. The key aim of this clinical case study UK is to provide a clinical decision support mechanism for Raigmore Hospital's Rapid Access Chest Pain Clinic (RACPC) patients by combining evidence, extrapolated through legacy patient data (based on machine learning driven techniques) to facilitate evidence based cardiovascular preventative care. The machine learning driven prognostic system provides cardiac chest pain prognosis through a cardiac chest pain specific prognostic model which is validated through consultant cardiologist from Raigmore Hospital. The cardiac chest pain prognostic model could help clinicians diagnose cardiac chest pain patients efficiently and could also help clinicians reduce load on overly prescribed angiography treatment in a cost effective manner. Additional two clinical case studies in the heart disease and breast cancer domains are considered for the development and clinical validation of the machine learning driven prognostic system. The proposed novel ontology and machine learning driven hybrid clinical decision support framework will also be validated in other application areas.
更多查看译文
关键词
risk management,ontologies
AI 理解论文
溯源树
样例
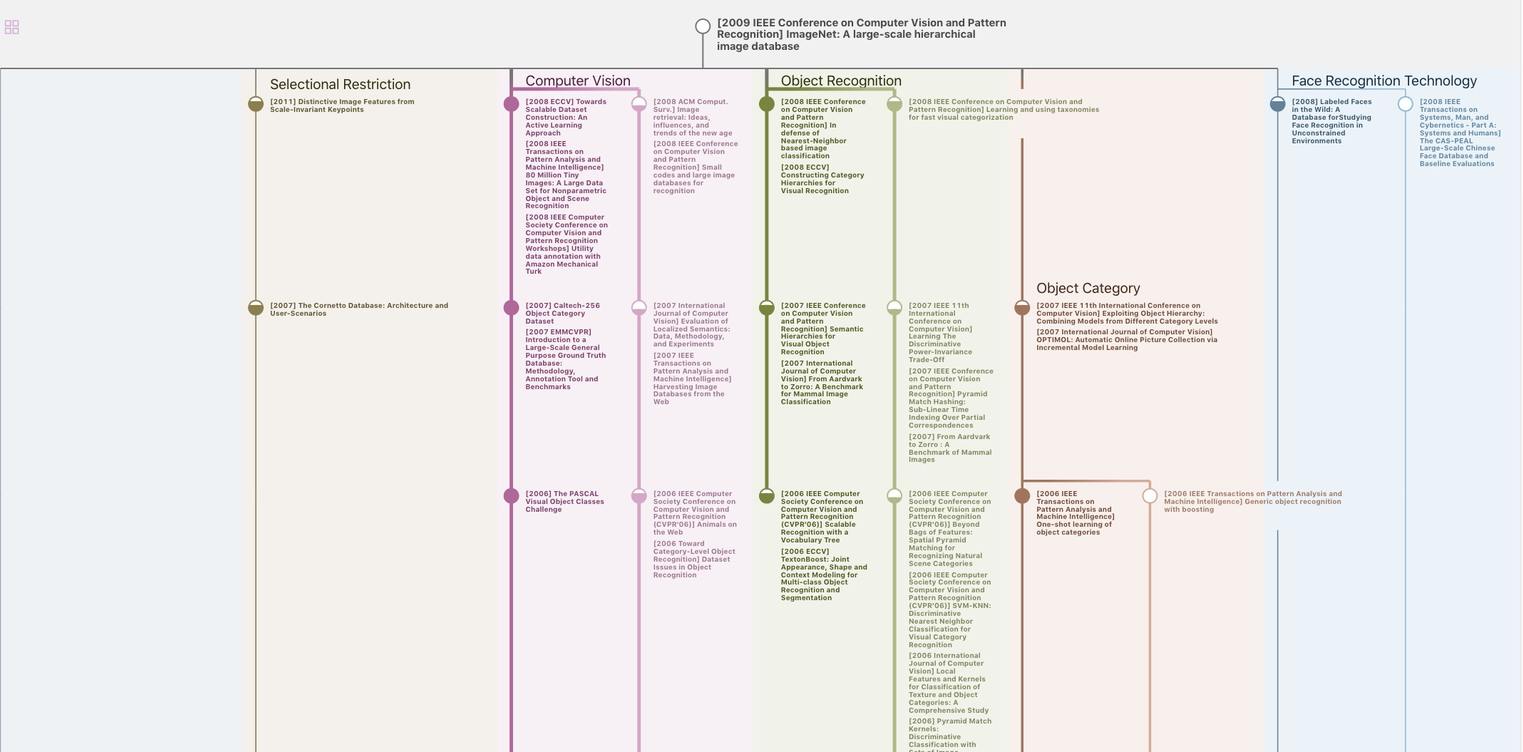
生成溯源树,研究论文发展脉络
Chat Paper
正在生成论文摘要