Multiple views as aid to linguistic annotation error analysis
LAW@COLING(2014)
摘要
This paper describes a methodology for supporting the task of annotating sentiment in natural language by detecting borderline cases and inconsistencies. Inspired by the co-training strategy, a number of machine learning models are trained on different views of the same data. The predictions obtained by these models are then automatically compared in order to bring to light highly uncertain annotations and systematic mistakes. We tested the methodology against an English corpus annotated according to a fine-grained sentiment analysis annotation schema (SentiML). We detected that 153 instances (35%) classified differently from the gold standard were acceptable and further 69 instances (16%) suggested that the gold standard should have been improved.
更多查看译文
关键词
multiple views
AI 理解论文
溯源树
样例
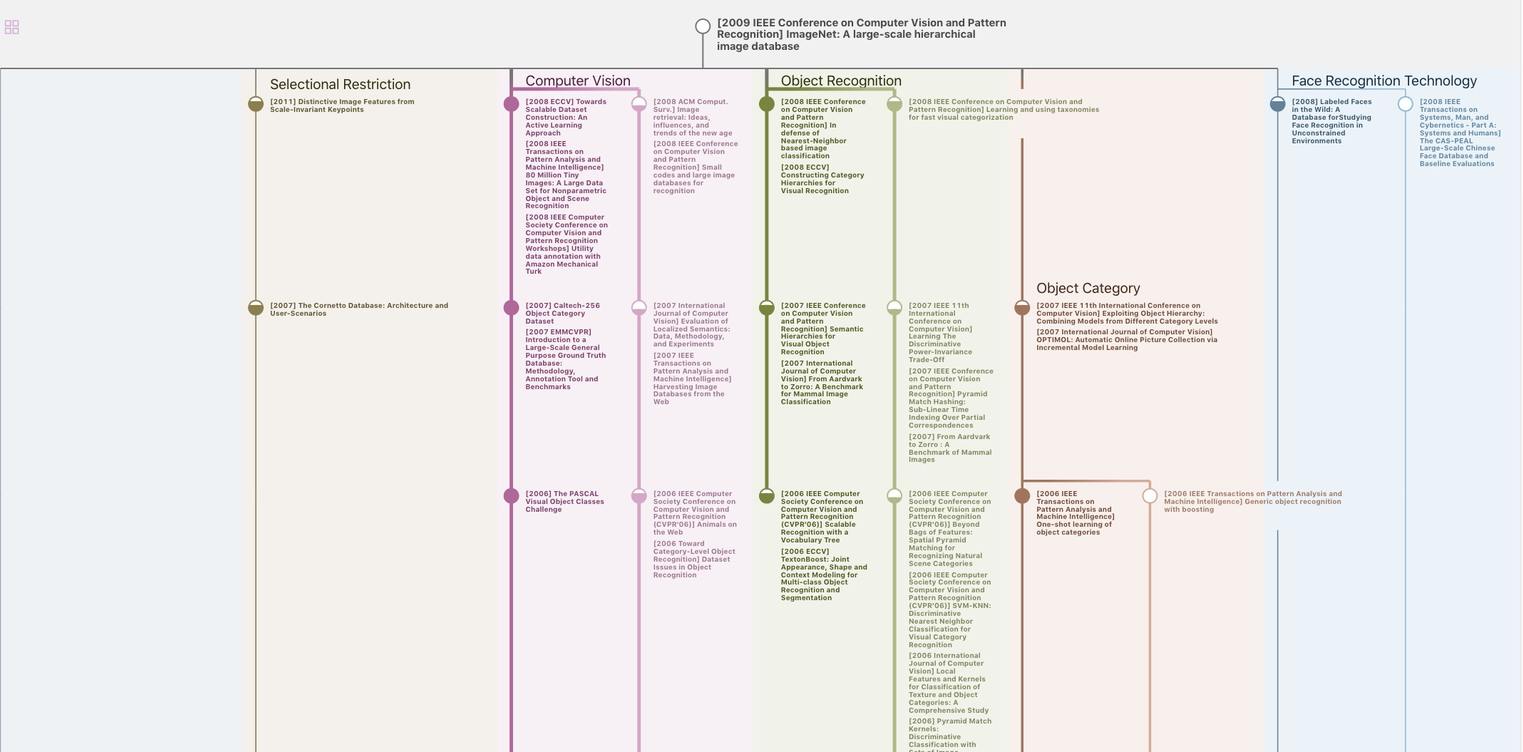
生成溯源树,研究论文发展脉络
Chat Paper
正在生成论文摘要