Learning Subspaces of Different Dimensions
JOURNAL OF COMPUTATIONAL AND GRAPHICAL STATISTICS(2022)
摘要
We introduce a Bayesian model for inferring mixtures of subspaces of different dimensions. The model allows flexible and efficient learning of a density supported in an ambient space which in fact can concentrate around some lower-dimensional space. The key challenge in such a mixture model is specification of prior distributions over subspaces of different dimensions. We address this challenge by embedding subspaces or Grassmann manifolds into a sphere of relatively low dimension and specifying priors on the sphere. We provide an efficient sampling algorithm for the posterior distribution of the model parameters. We illustrate that a simple extension of our mixture of subspaces model can be applied to topic modeling. The utility of our approach is demonstrated with applications to real and simulated data.
更多查看译文
关键词
Conway embedding, Mixtures of subspaces, Subspaces of different dimensions
AI 理解论文
溯源树
样例
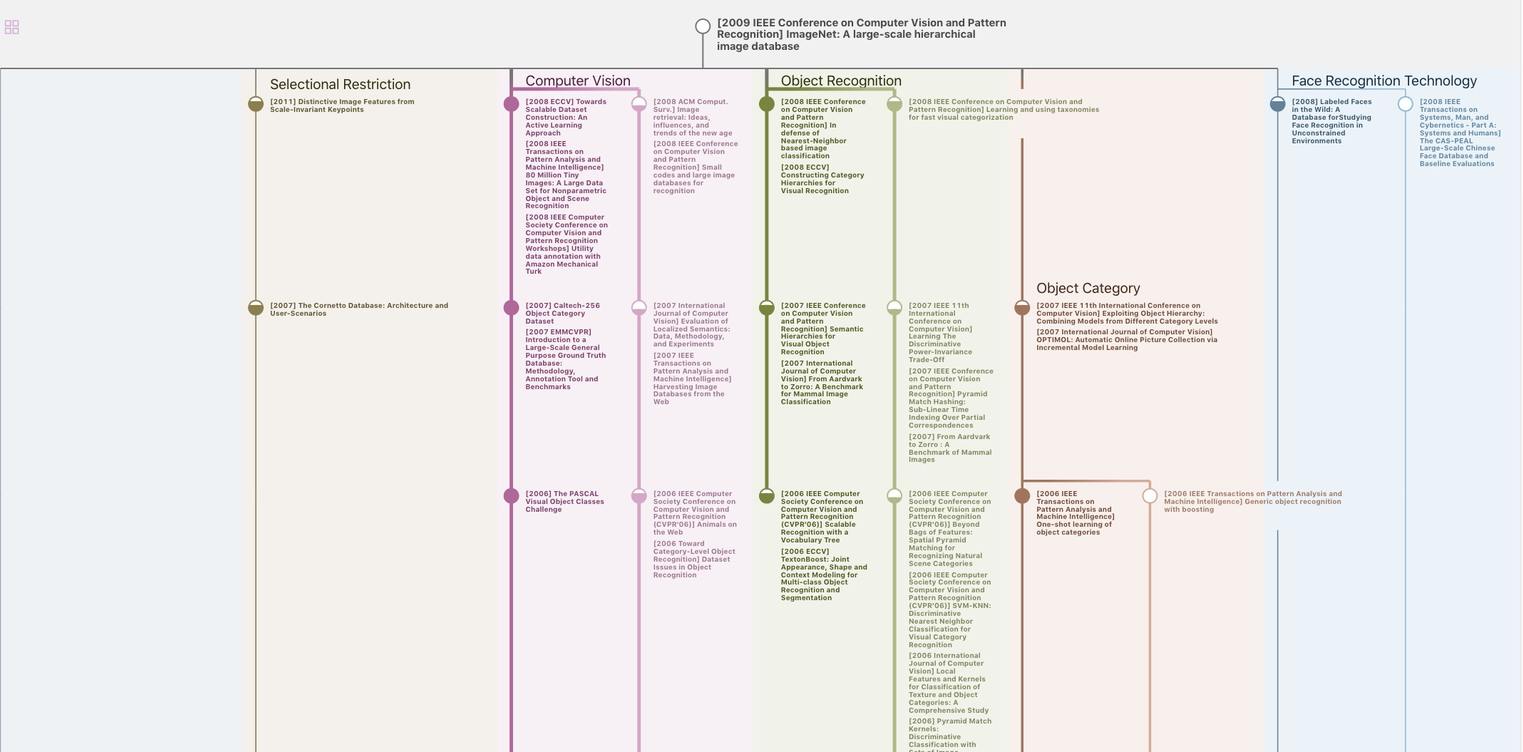
生成溯源树,研究论文发展脉络
Chat Paper
正在生成论文摘要