A Comparison of Optimization Methods for Large-scale L 1-regularized Linear Classification
mag(2010)
摘要
Large-scale linear classification is widely used in many areas. The L1-regularized form can be applied for feature selection, but its non-differentiability causes more difficulties in training. Various optimization methods have been proposed in recent years, but no serious comparison among them has been made. In this paper, we discuss several state of the art methods and propose two new implementations. We then conduct a comprehensive comparison. Results show that decomposition methods, in particular coordinate descent methods, are very suitable for training large document data.
更多查看译文
关键词
support vector machines,linear classification,l1 regularization,logistic regression
AI 理解论文
溯源树
样例
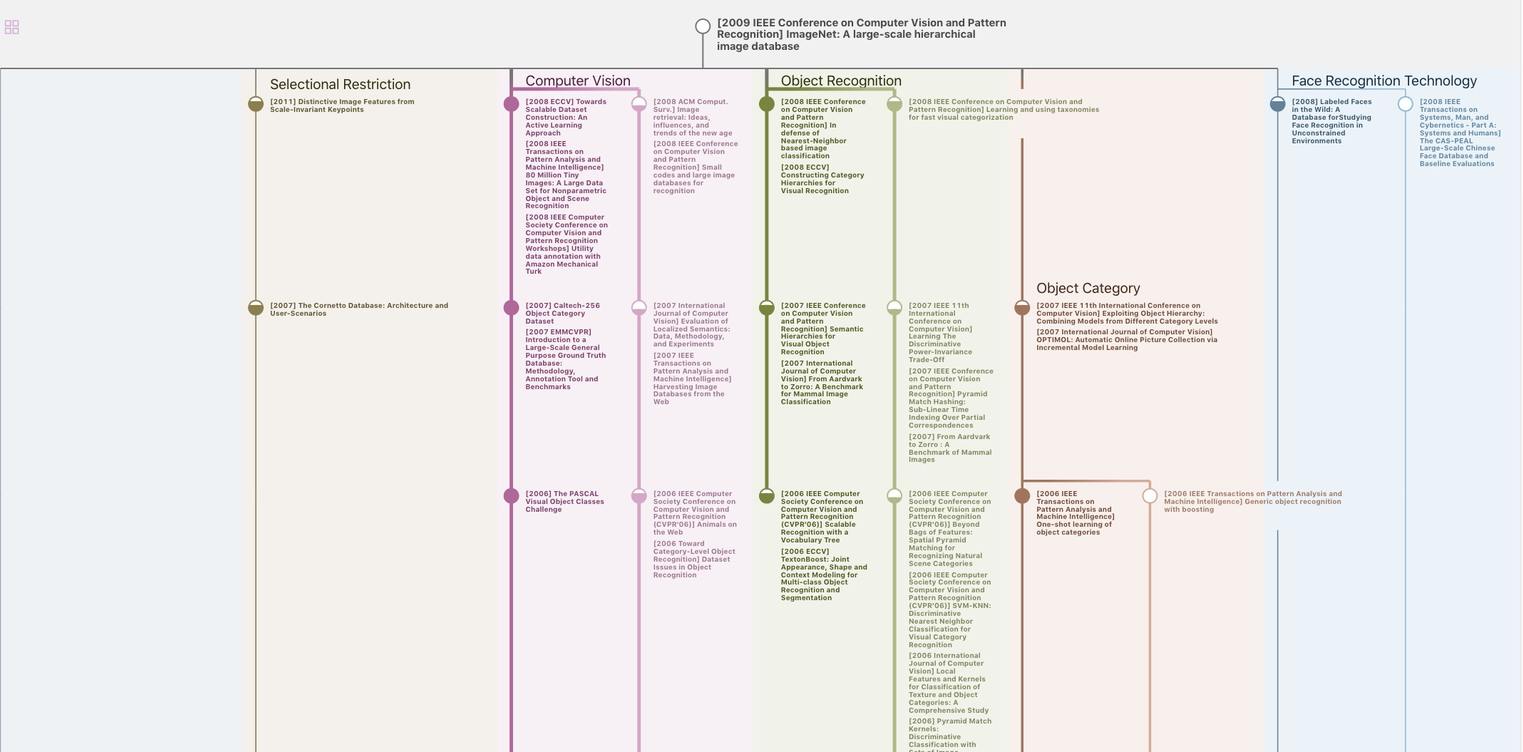
生成溯源树,研究论文发展脉络
Chat Paper
正在生成论文摘要