Supervised hyperspectral image segmentation: A convex formulation using hidden fields
2014 6th Workshop on Hyperspectral Image and Signal Processing: Evolution in Remote Sensing (WHISPERS)(2014)
摘要
Image segmentation is fundamentally a discrete problem. It consists of finding a partition of the image domain such that the pixels in each element of the partition exhibit some kind of similarity. The optimization is obtained via integer optimization which is NP-hard, apart from few exceptions. We sidestep from the discrete nature of image segmentation by formulating the problem in the Bayesian framework and introducing a hidden set of real-valued random fields determining the probability of a given partition. Armed with this model, the original discrete optimization is converted into a convex program. To infer the hidden fields, we introduce the Segmentation via the Constrained Split Augmented Lagrangian Shrinkage Algorithm (SegSALSA). The effectiveness of the proposed methodology is illustrated with hyperspectral image segmentation.
更多查看译文
关键词
Image segmentation,hidden Markov measure fields,hidden fields,alternating optimization,Constrained Split Augmented Lagrangian Shrinkage Algorithm (SALSA)
AI 理解论文
溯源树
样例
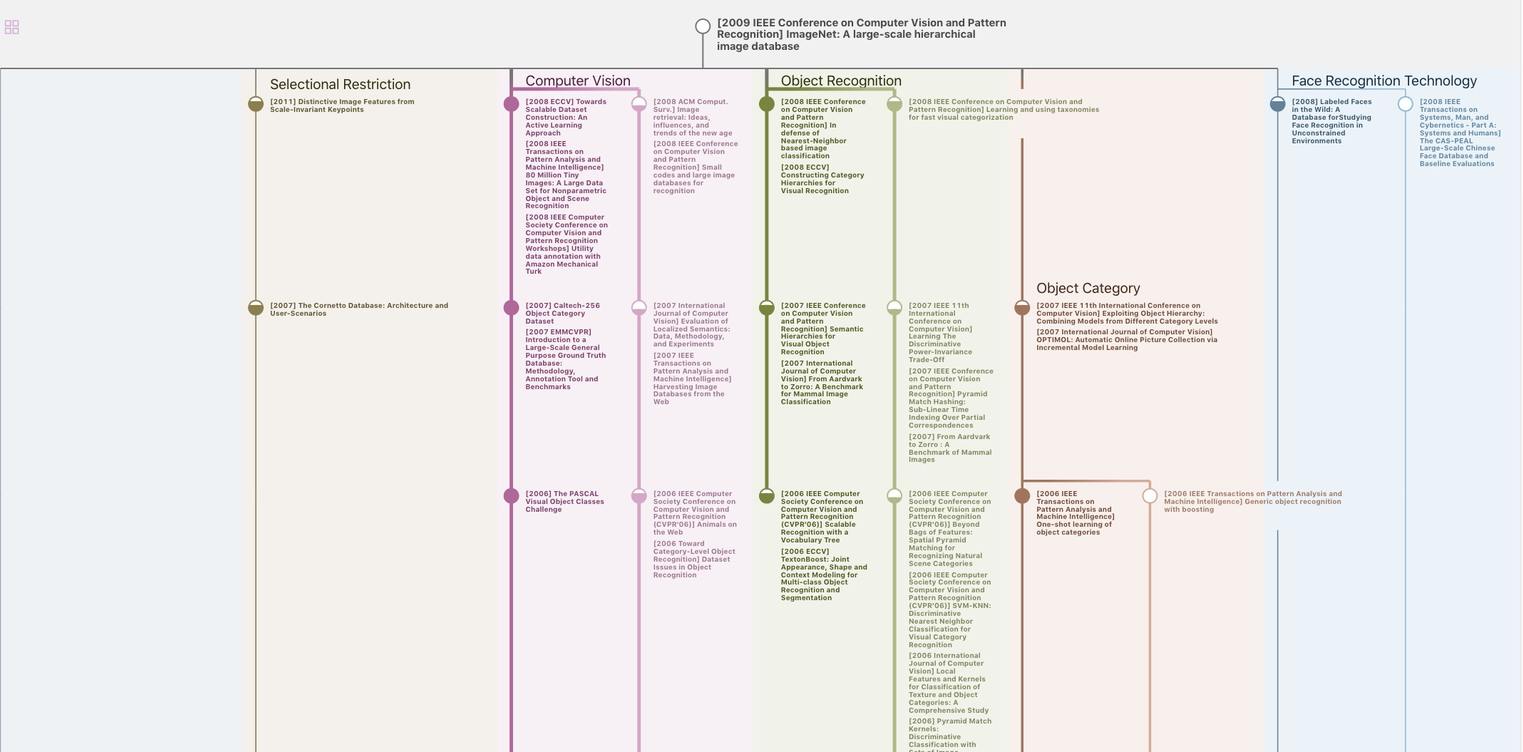
生成溯源树,研究论文发展脉络
Chat Paper
正在生成论文摘要