Gpu-Enabled Pet Motion Compensation Using Sparse And Low-Rank Decomposition
2012 IEEE NUCLEAR SCIENCE SYMPOSIUM AND MEDICAL IMAGING CONFERENCE RECORD (NSS/MIC)(2012)
摘要
The resolution and signal-to-noise ratio (SNR) of motion-compensated PET images depend highly on the motion estimation accuracy. However, in many practical settings, the estimated motion information contains noise. In this work, we propose a fast and accurate method to incorporate noisy motion estimation into PET image reconstruction in a systematic framework using multiscale sliding time window reconstruction followed by matrix decomposition.In the first step, we propose multiscale sliding window to trade off the resolution and the SNR of the images. A Gaussian window is placed at multiple time points in the list-mode data, giving higher weight to the events that have less motion artifact relative to the current window position. The window size for each position is varied, generating high SNR images with wide windows and high resolution images with narrow windows.In the second step, we use matrix decomposition to combine the set of images in the first step into an image that has both high resolution and high SNR. More specifically, the results generated by different windows are organized into a matrix with each column representing an image. In the ideal case, the images should be highly correlated with each other. Based on this observation, we decompose the matrix into the sum of a low-rank matrix and a sparse matrix. The low-rank matrix represents the high resolution and high SNR image, while the sparse matrix corresponds to noise.The proposed method is implemented on a graphics processing unit (GPU) using the compute unified device architecture (CUDA) framework for fast execution. Experiments on a simulated data set demonstrate that the proposed approach can effectively preserve the high resolution of the narrow time window while maintaining the low noise of the wide time window. Quantitative analysis shows 22.4% improvement in spatial resolution, 16.7% reduction in noise, and 20.9% improvement in contrast-to-noise ratio compared to the baseline method.
更多查看译文
关键词
motion compensation,motion estimation,matrix decomposition
AI 理解论文
溯源树
样例
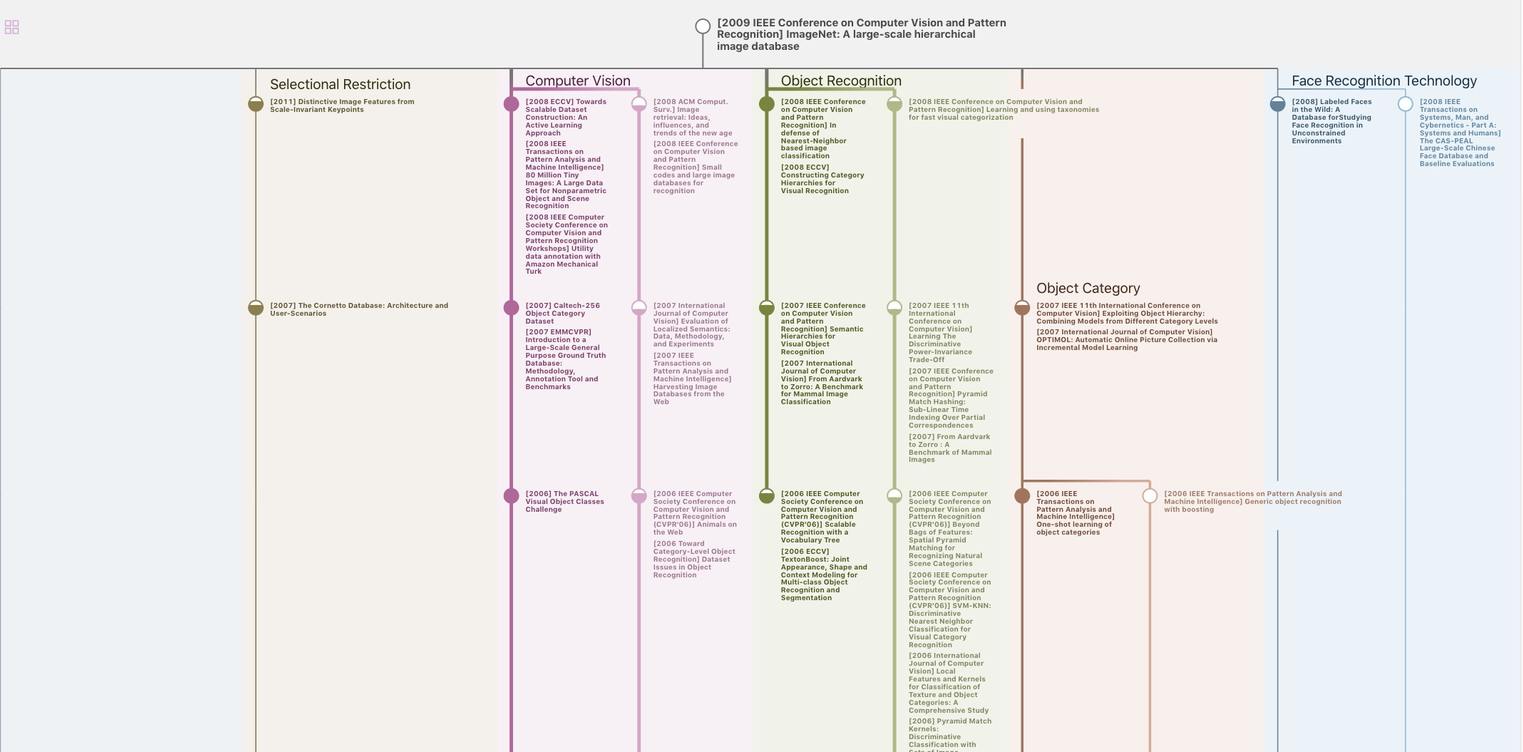
生成溯源树,研究论文发展脉络
Chat Paper
正在生成论文摘要