QoE-Based SVC Layer Dropping in LTE Networks Using Content-Aware Layer Priorities
TOMCCAP(2015)
摘要
The increasing popularity of mobile video streaming applications has led to a high volume of video traffic in mobile networks. As the base station, for instance, the eNB in LTE networks, has limited physical resources, it can be overloaded by this traffic. This problem can be addressed by using Scalable Video Coding (SVC), which allows the eNB to drop layers of the video streams to dynamically adapt the bitrate. The impact of bitrate adaptation on the Quality of Experience (QoE) for the users depends on the content characteristics of videos. As the current mobile network architectures do not support the eNB in obtaining video content information, QoE optimization schemes with explicit signaling of content information have been proposed. These schemes, however, require the eNB or a specific optimization module to process the video content on the fly in order to extract the required information. This increases the computation and signaling overhead significantly, raising the OPEX for mobile operators. To address this issue, in this article, a content-aware (CA) priority marking and layer dropping scheme is proposed. The CA priority indicates a transmission order for the layers of all transmitted videos across all users, resulting from a comparison of their utility versus rate characteristics. The CA priority values can be determined at the P-GW on the fly, allowing mobile operators to control the priority marking process. Alternatively, they can be determined offline at the video servers, avoiding real-time computation in the core network. The eNB can perform content-aware SVC layer dropping using only the priority values. No additional content processing is required. The proposed scheme is lightweight both in terms of architecture and computation. The improvement in QoE is substantial and very close to the performance obtained with the computation and signaling-intensive QoE optimization schemes.
更多查看译文
关键词
lte
AI 理解论文
溯源树
样例
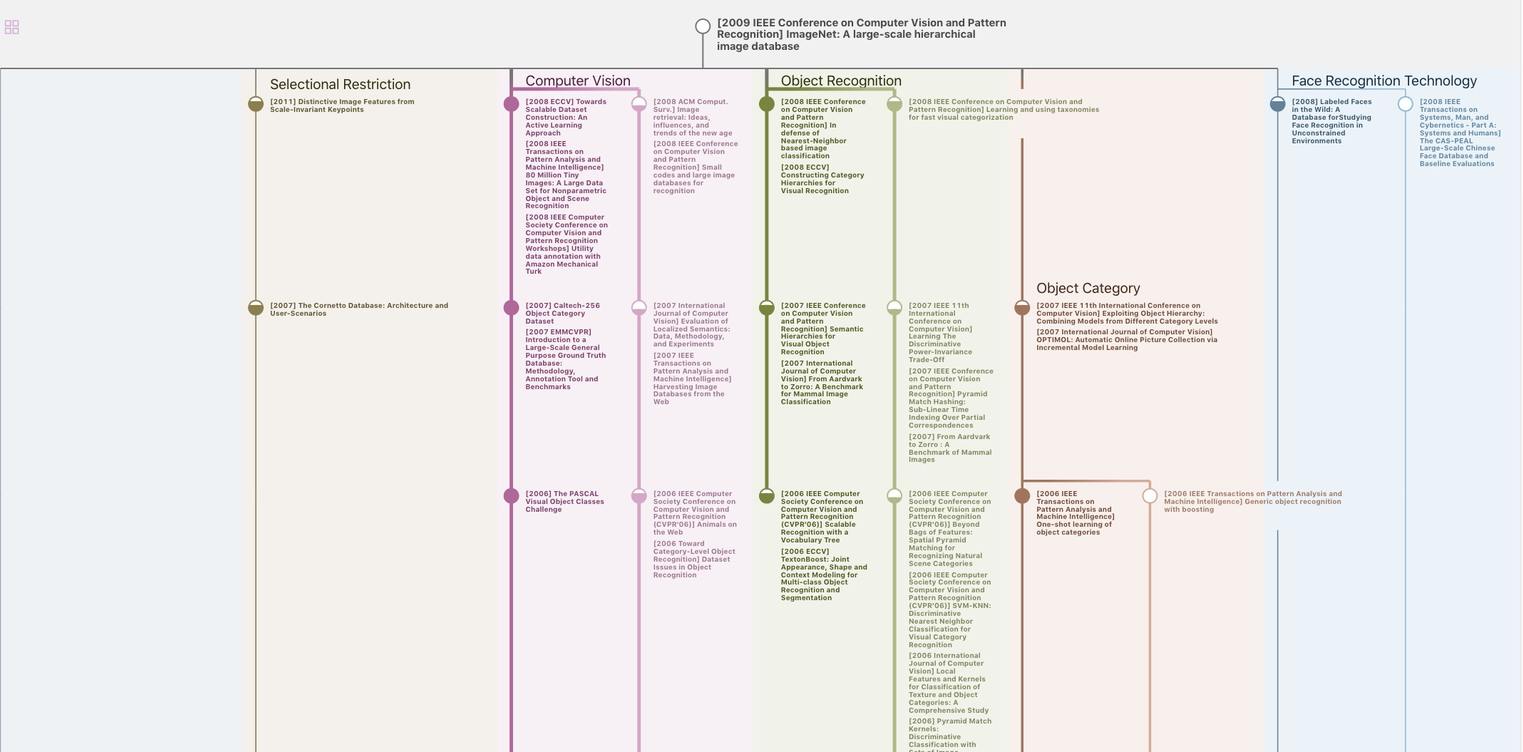
生成溯源树,研究论文发展脉络
Chat Paper
正在生成论文摘要