Particle Control in Phase Space by Global K-Means Clustering
mag(2015)
摘要
We devise and explore an iterative optimization procedure for controlling particle populations in particle-in-cell (PIC) codes via merging and splitting of computational macro-particles. Our approach, is to compute an optimal representation of the global particle phase space structure while decreasing or increasing the entire particle population, based on k-means clustering of the data. In essence the procedure amounts to merging or splitting particles by statistical means, throughout the entire simulation volume in question, while minimizing a 6-dimensional total distance measure to preserve the physics. Particle merging is by far the most demanding procedure when considering conservation laws of physics; it amounts to lossy compression of particle phase space data. We demonstrate that our k-means approach conserves energy and momentum to high accuracy, even for high compression ratios, $\mathcal{R} \approx 3$ --- \emph{i.e.}, $N_{f} \lesssim 0.33N_{i}$. Interestingly, we find that an accurate particle splitting step can be performed using k-means as well; this from an argument of symmetry. The split solution, using k-means, places splitted particles optimally, to obtain maximal spanning on the phase space manifold. Implementation and testing is done using an electromagnetic PIC code, the \ppcode. Nonetheless, the k-means framework is general; it is not limited to Vlasov-Maxwell type PIC codes. We discuss advantages and drawbacks of this optimal phase space reconstruction.
更多查看译文
AI 理解论文
溯源树
样例
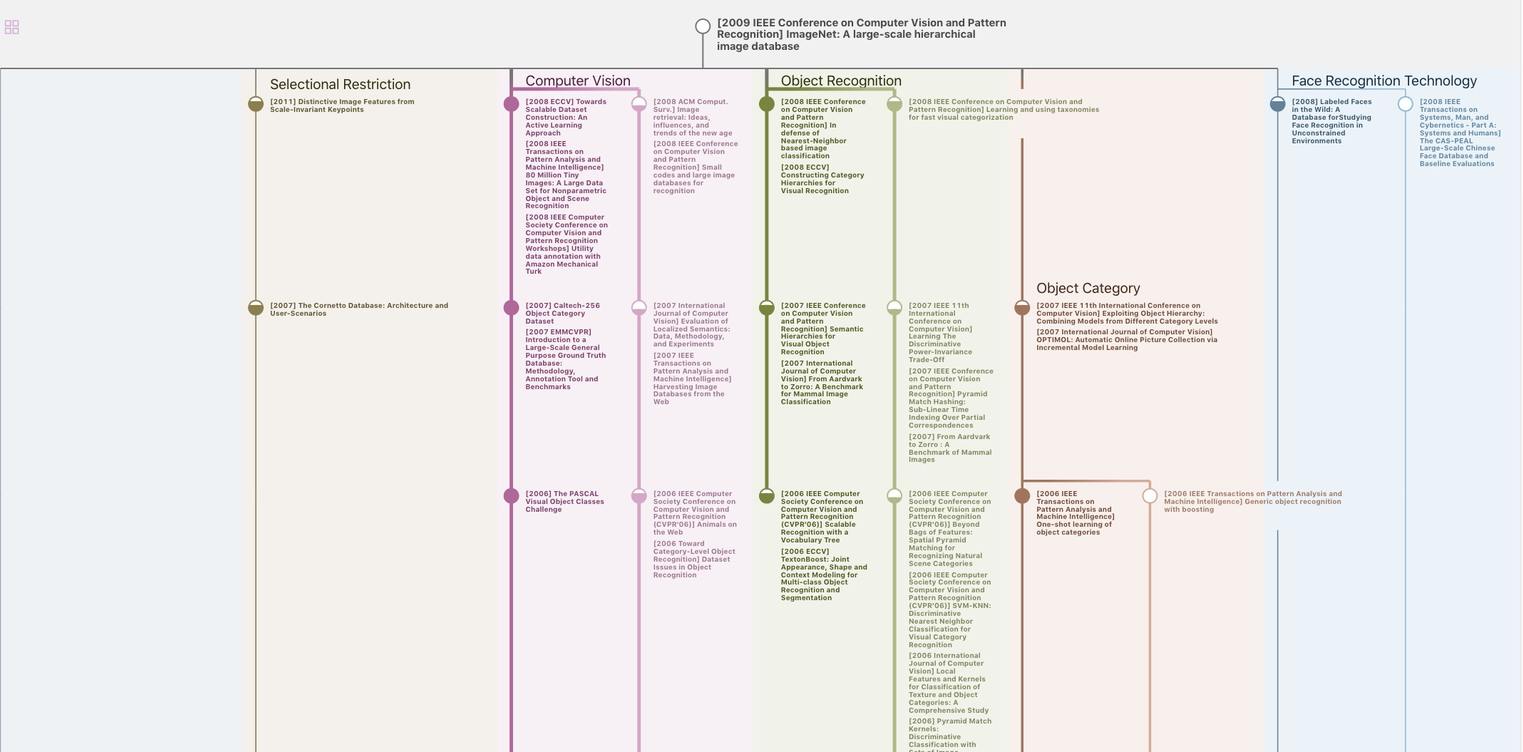
生成溯源树,研究论文发展脉络
Chat Paper
正在生成论文摘要