Localization with Improved Proposals
mag(2010)
摘要
Solving localization and navigation tasks reliably is an essential objective for autonomous mobile systems and robots. A popular technique for estimating the robot’s pose is localization with particle filters, also known as Monte–Carlo localization (MCL). In this paper we present a MCL–based localization system that employs informed proposal distributions to sample particles during the motion step of the filter. While the standard MCL model computes the sampling proposal distribution based only on the movement on the robot, our approach also takes the most recent sensor observation into account. Experiments using datasets gathered by real robots in an indoor environment show that our approach is able to estimate the robot’s pose with less uncertainty than the standard MCL implementation.
更多查看译文
AI 理解论文
溯源树
样例
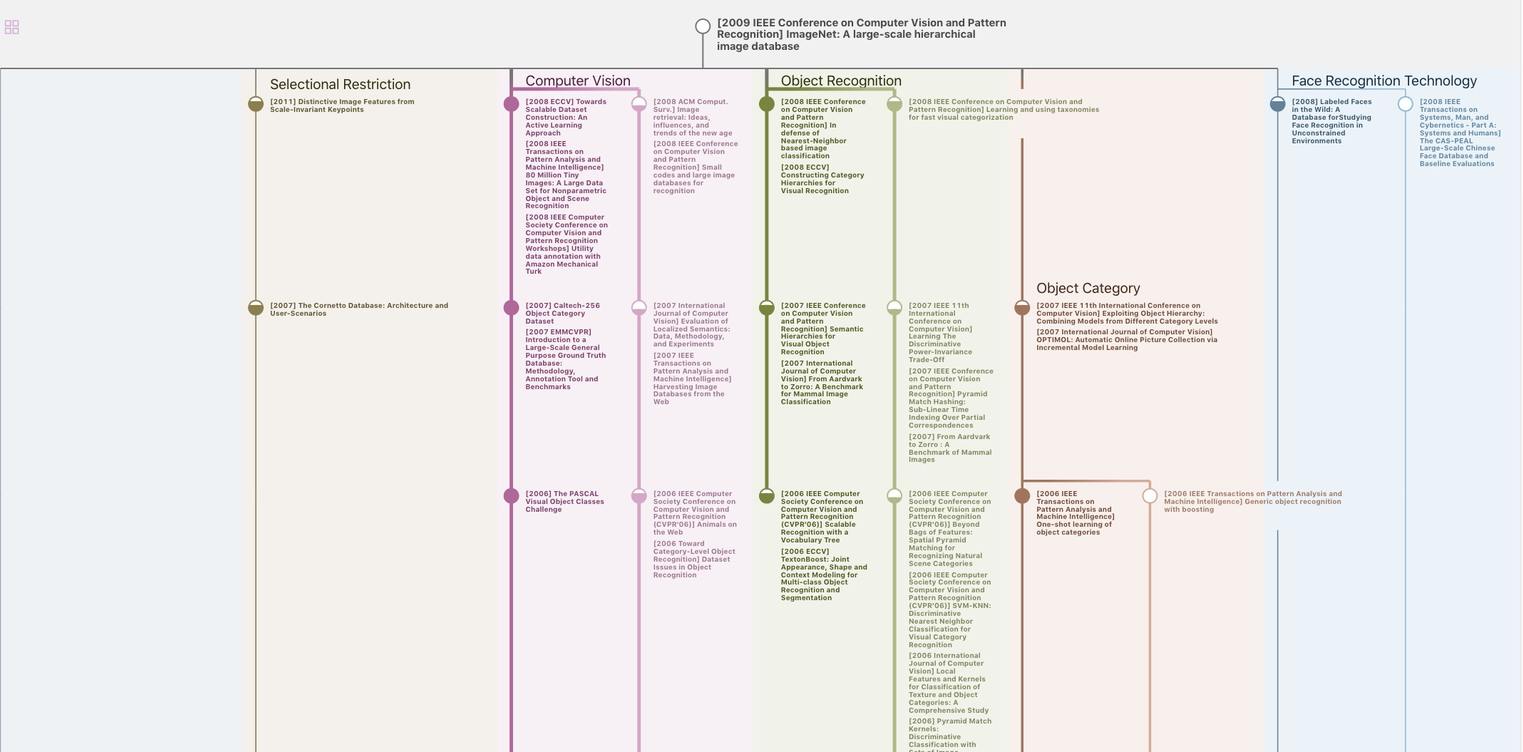
生成溯源树,研究论文发展脉络
Chat Paper
正在生成论文摘要