Improvements to multivariate data analysis and monitoring of batch processes by multilevel methods
Journal of Chemometrics(2012)
摘要
Batch process data contain between-run and within-run sources of variation, which complicates data analysis and process monitoring. Multilevel methods, such as multilevel simultaneous component analysis, greatly enhance the interpretation of large sets of batch process data by separating the between-run and within-run variation. Using a multilevel approach in batch process monitoring greatly reduces the occurrence of false alarms that are caused by trivial variations and process changes that were made on purpose. This also reduces the need for model updating, which often is a large effort and causes downtime of the monitoring system. Furthermore, relevant phenomena that are masked by larger but uninteresting sources of variation when standard batch process monitoring methods are applied can often be detected if the multilevel approach is used. Chemical batch process data are used to illustrate the methods. Copyright (c) 2012 John Wiley & Sons, Ltd.
更多查看译文
关键词
multilevel models,MLSCA,MSPC,batch process monitoring,false alarms
AI 理解论文
溯源树
样例
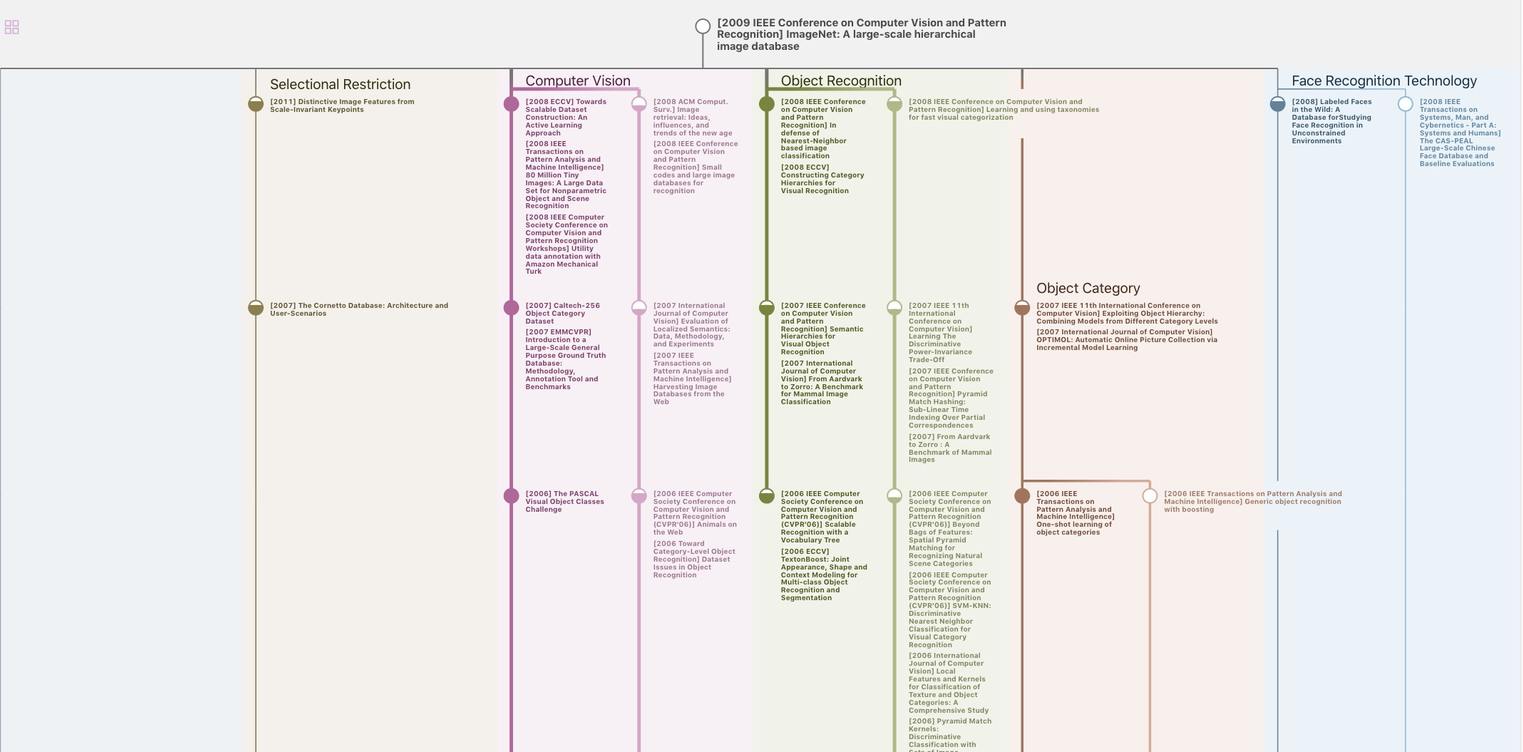
生成溯源树,研究论文发展脉络
Chat Paper
正在生成论文摘要