Neuro-Ophthalmic Literature Review
Neuro-Ophthalmology(2022)
摘要
Neuro-Ophthalmic Literature Review David A. Bellows, Noel C.Y. Chan, John J. Chen, Hui-Chen Cheng, Peter W MacIntosh, Jenny A. Nij Bijvank, and Michael S. Vaphiades Smoking, among other factors, carries an increased risk of conversion from ocular to generalised myasthenia gravis. Apinyawasisuk S, Chongpison Y, Thitisaksakul C, Jariyakosol S. Factors affecting generalization of ocular myasthenia gravis in patients with positive acetylcholine receptor antibody. Am J Ophthalmol 2020;209:10–17. doi:10.1016/j.ajo.2019.09.019 The purpose of this retrospective study was to identify those factors that were associated with an increased or decreased risk of conversion from ocular myasthenia gravis to generalised myasthenia gravis. The authors reviewed the records of 71 patients who presented with ocular myasthenia, between July 2009 and December 2016, and had a positive test for acetylcholine receptor antibody. At the end of the study period, 35 patients remained purely ocular myasthenic and 36 patients converted to generalised myasthenia gravis. Factors associated with conversion to generalised myasthenia gravis, and their adjusted odds ratios, include female sex (4.02), smoking (6.13) and the presence of a thymic abnormality (4.13). Factors associated with a lower risk of conversion to generalised myasthenia gravis included treatment with immunosuppressive agents (0.47) and pyridostigmine (0.23). The age of onset of ocular myasthenia gravis and the presence of other autoimmune disorders were not found to increase or decrease the risk of conversion to generalised myasthenia gravis. David A. Bellows Artificial intelligence for disc swelling: Does it work? Ahn, JM, Kim S, Ahn K-S, Cho S-H, Kim US. Accuracy of machine learning for differentiation between optic neuropathies and pseudopapilledema. BMC Opthalmol 2019;19:178. doi:10.1186/ s12886-019-1184-0 Artificial intelligence has been a hot topic in the evaluation of various ophthalmic disorders such as glaucoma and diabetic retinopathy. In neurology, it has also been evaluated for predicting dementia at an early stage. It is not surprising that this technology will eventually be employed in the field of neuroophthalmology. This study tried to evaluate the efficacy of machine learning for differentiating optic neuropathies, pseudo-papilloedema, and normal controls using fundus photographs. Non-mydriatic fundus cameras were used to capture a total of 1369 images from the three groups, which include 295 images of optic neuropathies, 295 of pseudo-papilloedema and 779 normal control images. The optic neuropathies group comprised 177 cases of ischaemic optic neuropathy, 48 of optic neuritis, 17 of diabetic optic neuropathy, 22 of papilloedema, and 31 of disc swelling secondary to retinal disorders (such as central retinal vein occlusion). All subjects in the pseudo-papilloedema group had normal visual function for more than 1 year with normal optical coherence tomography (OCT) findings. The entire set of images were split into an 876-image training dataset, a 274-image validation dataset and a 219image testing dataset. Aside from the authors’ CONTACT John J. Chen Chen.john@mayo.edu Department of Ophthalmology, Mayo Clinic, 200 First Street, SW, Rochester, MN 55905, USA NEURO-OPHTHALMOLOGY 2020, VOL. 44, NO. 2, 132–138 https://doi.org/10.1080/01658107.2020.1717901 © 2020 Taylor & Francis Group, LLC model, three other well-known convolutional neural networks were also tested. The authors showed that the accuracy of machine learning ranged from 95.89% to 98.63% with a high AUROC score of 0.999 in two of the classifier models. In real life, diagnosing pseudo-papilloedema often requires the aid of a number of investigative modalities aside from comprehensive history and examinations. Imaging analysis such as B-scan ultrasonography, fundus photography, autofluorescence, fluorescein angiogram, and OCT are often required to establish the diagnosis. This study results are promising, given the highpredictive capability of machine learning with fundus photo alone. Artificial intelligence appears to have an important role in the future algorithm although further large-scale validation is warranted.
更多查看译文
关键词
literature review,neuro-ophthalmic
AI 理解论文
溯源树
样例
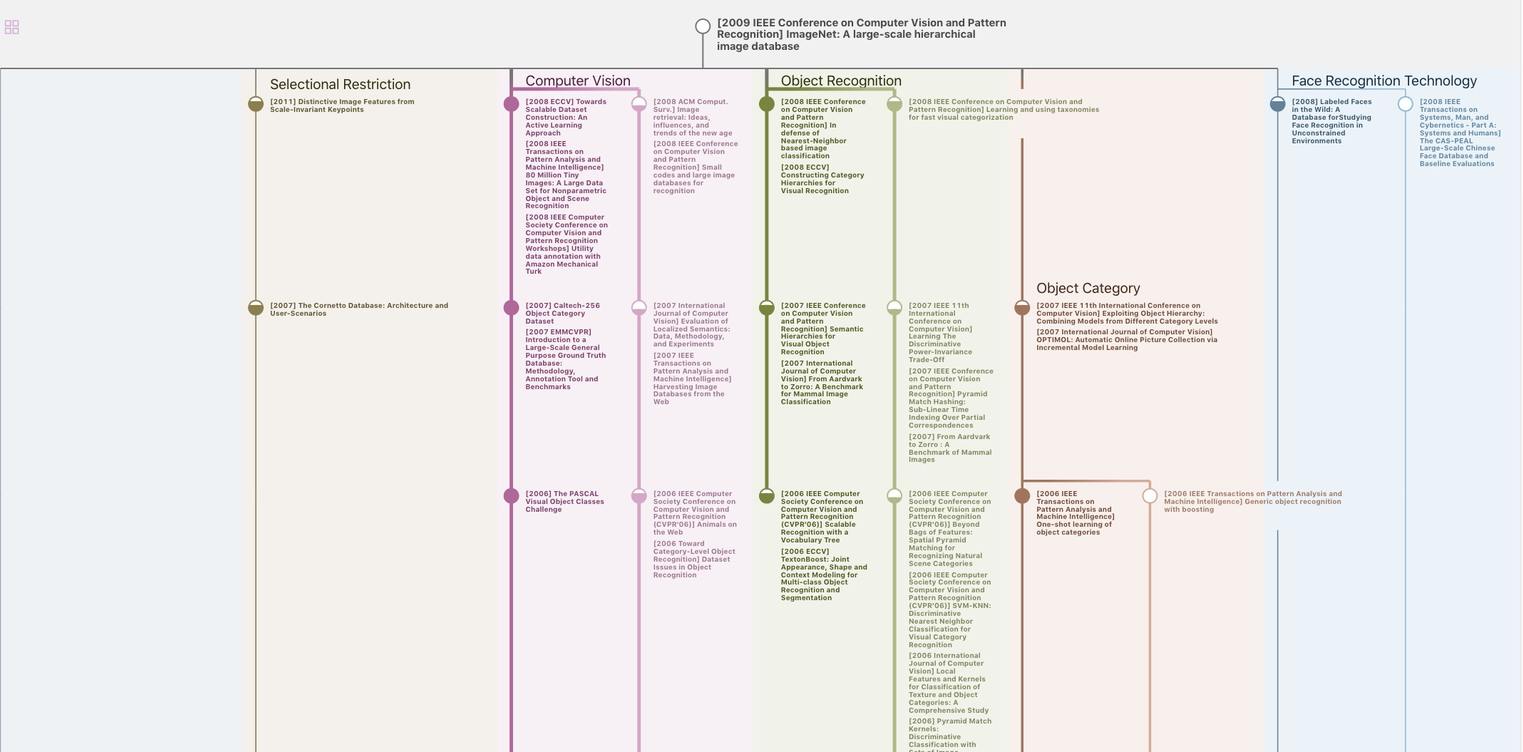
生成溯源树,研究论文发展脉络
Chat Paper
正在生成论文摘要