Using a false biofeedback methodology to explore relationships between learners’ affect, metacognition, and performance
Contemporary Educational Psychology(2013)
摘要
We used a false-biofeedback methodology to manipulate physiological arousal in order to induce affective states that would influence learners’ metacognitive judgments and learning performance. False-biofeedback is a method used to induce physiological arousal (and resultant affective states) by presenting learners with audio stimuli of false heart beats. Learners were presented with accelerated, baseline, or no heart beat (control) while they completed a challenging learning task. We tested four hypotheses about the effect of false-biofeedback. The alarm vs. alert hypothesis predicted that false biofeedback would be appraised as either a signal of distress and would impair learning (alarm), or as a signal of engagement and would facilitate learning (alert). The differential biofeedback hypothesis predicted that the alarm and alert effects would be dependent on the type of biofeedback (accelerated vs. baseline). The question depth hypothesis predicted that these effects would be more pronounced for challenging inference questions. Lastly, the self vs. recording hypothesis predicted that effects would only occur if participants believed that false biofeedback was indicative of their own physiological arousal. In general, learners experienced more positive/activating affective states, made more confident metacognitive judgments, and achieved higher learning when they received accelerated or baseline biofeedback while answering a challenging inference question, irrespective of the perceived source of the biofeedback. Thus, our findings supported the alert and question depth hypotheses, but not the differential biofeedback or self vs. recording hypotheses. Implications of the findings for the integration of affective processes into models of cognitive and metacognitive processes during learning are discussed.
更多查看译文
关键词
Affect,Metacognition,Learning outcomes,Self-regulated learning
AI 理解论文
溯源树
样例
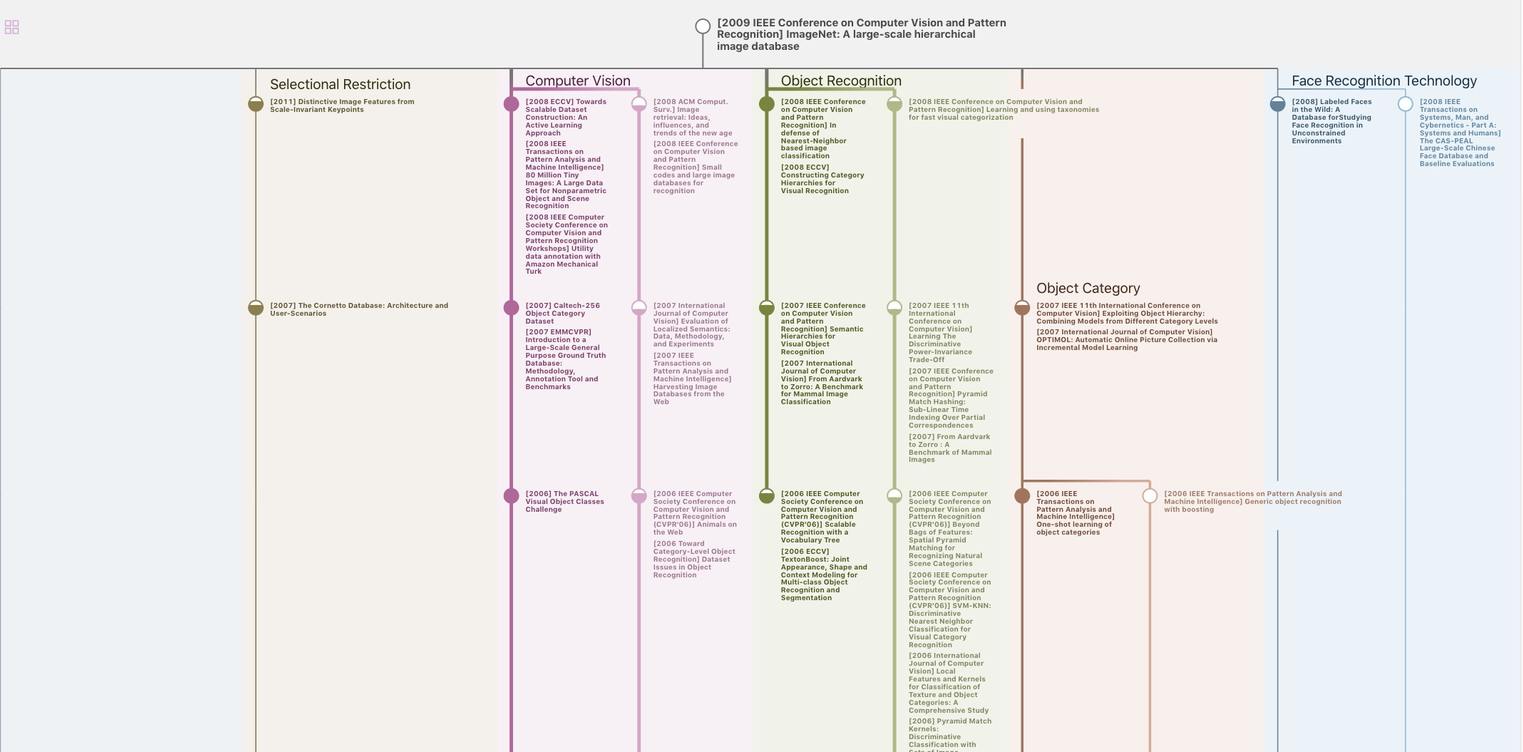
生成溯源树,研究论文发展脉络
Chat Paper
正在生成论文摘要