Application of Machine Learning Algorithms for the Query Performance Prediction
ADVANCES IN ELECTRICAL AND COMPUTER ENGINEERING(2015)
摘要
This paper analyzes the relationship between the system load/throughput and the query response time in a real Online transaction processing (OLTP) system environment. Although OLTP systems are characterized by short transactions, which normally entail high availability and consistent short response times, the need for operational reporting may jeopardize these objectives. We suggest a new approach to performance prediction for concurrent database workloads, based on the system state vector which consists of 36 attributes. There is no bias to the importance of certain attributes, but the machine learning methods are used to determine which attributes better describe the behavior of the particular database server and how to model that system. During the learning phase, the system's profile is created using multiple reference queries, which are selected to represent frequent business processes. The possibility of the accurate response time prediction may be a foundation for automated decision-making for database (DB) query scheduling. Possible applications of the proposed method include adaptive resource allocation, quality of service (QoS) management or real-time dynamic query scheduling (e.g. estimation of the optimal moment for a complex query execution).
更多查看译文
关键词
machine learning,prediction algorithms,query processing,transaction databases
AI 理解论文
溯源树
样例
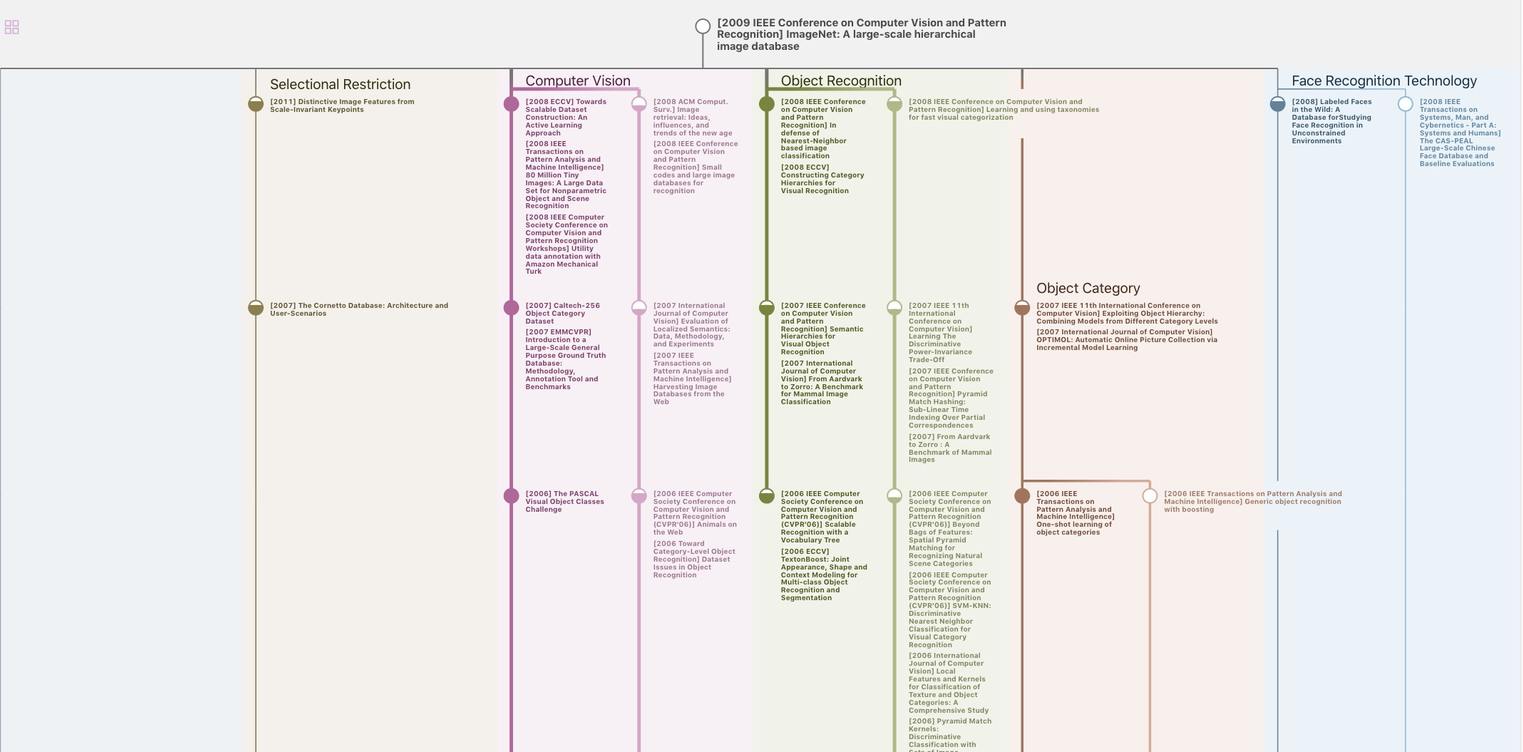
生成溯源树,研究论文发展脉络
Chat Paper
正在生成论文摘要