Prospective evaluation of a model-based dosing regimen for amikacin in preterm and term neonates in clinical practice
ANTIMICROBIAL AGENTS AND CHEMOTHERAPY(2015)
摘要
Based on a previously derived population pharmacokinetic model, a novel neonatal amikacin dosing regimen was developed. The aim of the current study was to prospectively evaluate this dosing regimen. First, early (before and after second dose) therapeutic drug monitoring (TDM) observations were evaluated for achieving target trough (<3 mg/liter) and peak (>24 mg/liter) levels. Second, all observed TDM concentrations were compared with model-predicted concentrations, whereby the results of a normalized prediction distribution error (NPDE) were considered. Subsequently, Monte Carlo simulations were performed. Finally, remaining causes limiting amikacin predictability (i.e., prescription errors and disease characteristics of outliers) were explored. In 579 neonates (median birth body weight, 2,285 [range, 420 to 4,850] g; postnatal age 2 days [range, 1 to 30 days]; gestational age, 34 weeks [range, 24 to 41 weeks]), 90.5% of the observed early peak levels reached 24 mg/liter, and 60.2% of the trough levels were <3 mg/liter (93.4% <= 5 mg/liter). Observations were accurately predicted by the model without bias, which was confirmed by the NPDE. Monte Carlo simulations showed that peak concentrations of >24 mg/liter were reached at steady state in almost all patients. Trough values of <3 mg/liter at steady state were documented in 78% to 100% and 45% to 96% of simulated cases with and without ibuprofen coadministration, respectively; suboptimal trough levels were found in patients with postnatal age <14 days and current weight of >2,000 g. Prospective evaluation of a model-based neonatal amikacin dosing regimen resulted in optimized peak and trough concentrations in almost all patients. Slightly adapted dosing for patient subgroups with suboptimal trough levels was proposed. This model-based approach improves neonatal dosing individualization.
更多查看译文
AI 理解论文
溯源树
样例
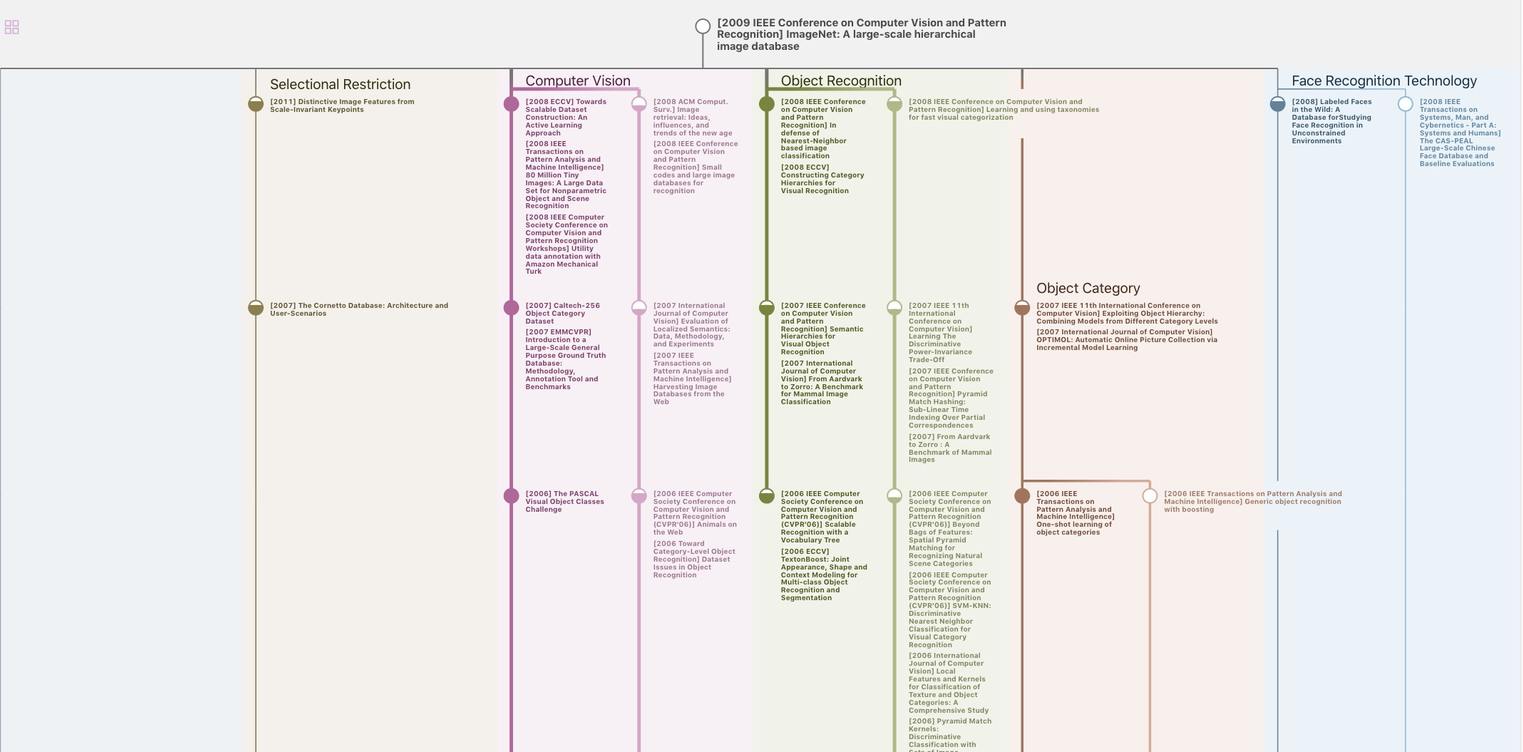
生成溯源树,研究论文发展脉络
Chat Paper
正在生成论文摘要