Bayesian inference for partially observed stochastic differential equations driven by fractional Brownian motion
BIOMETRIKA(2015)
摘要
We consider continuous-time diffusion models driven by fractional Brownian motion. Observations are assumed to possess a nontrivial likelihood given the latent path. Due to the non-Markovian and high-dimensional nature of the latent path, estimating posterior expectations is computationally challenging. We present a reparameterization framework based on the Davies and Harte method for sampling stationary Gaussian processes and use it to construct a Markov chain Monte Carlo algorithm that allows computationally efficient Bayesian inference. The algorithm is based on a version of hybrid Monte Carlo simulation that delivers increased efficiency when used on the high-dimensional latent variables arising in this context. We specify the methodology on a stochastic volatility model, allowing for memory in the volatility increments through a fractional specification. The method is demonstrated on simulated data and on the S&P 500/VIX time series. In the latter case, the posterior distribution favours values of the Hurst parameter smaller than 1/2, pointing towards medium-range dependence.
更多查看译文
关键词
Bayesian inference,Davies and Harte algorithm,Fractional Brownian motion,Hybrid Monte Carlo algorithm
AI 理解论文
溯源树
样例
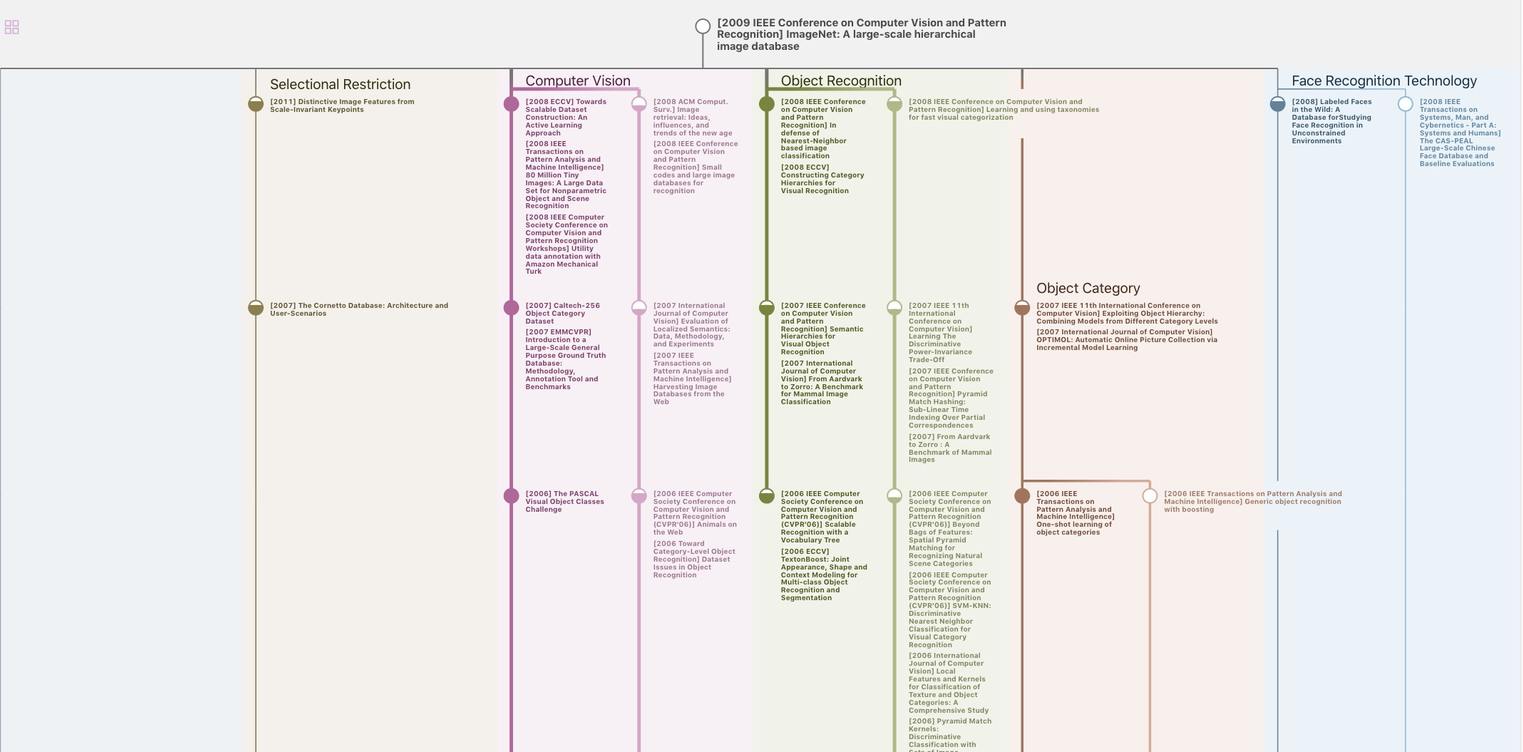
生成溯源树,研究论文发展脉络
Chat Paper
正在生成论文摘要