Spatio-temporal trend analysis of air temperature in Europe and Western Asia using data-coupled clustering
Global and Planetary Change(2015)
摘要
Over the last decades, different machine learning techniques have been used to detect climate change patterns, mostly using data from measuring stations located in different parts of the world. Some previous studies focus on temperature as primary variable of study, though there have been other works focused on precipitation or even wind speed as objective variable. In this paper, we use the self-organized Second Order Data Coupled Clustering (SODCC) algorithm to carry out a spatio-temporal analysis of temperature patterns in Europe. By applying the SODCC we identify three different regimes of spatio-temporal correlations based on their geographical extent: small, medium, and large-scale regimes. Based on these regimes, it is possible to detect a change in the spatio-temporal trend of air temperature, reflecting a shift in the extent of the correlations in stations in the Iberian Peninsula and Southern France. We also identify an oscillating spatio-temporal trend in the Western Asia region and a stable medium-scale regime affecting the British Isles. These results are found to be consistent with previous studies in climate change. The patterns obtained with the SODCC algorithm may represent a signal of climate change to be taken into account, and so the SODCC could be used as detection method.
更多查看译文
关键词
Air temperature,Clustering techniques,Spatio-temporal trend,Climate change
AI 理解论文
溯源树
样例
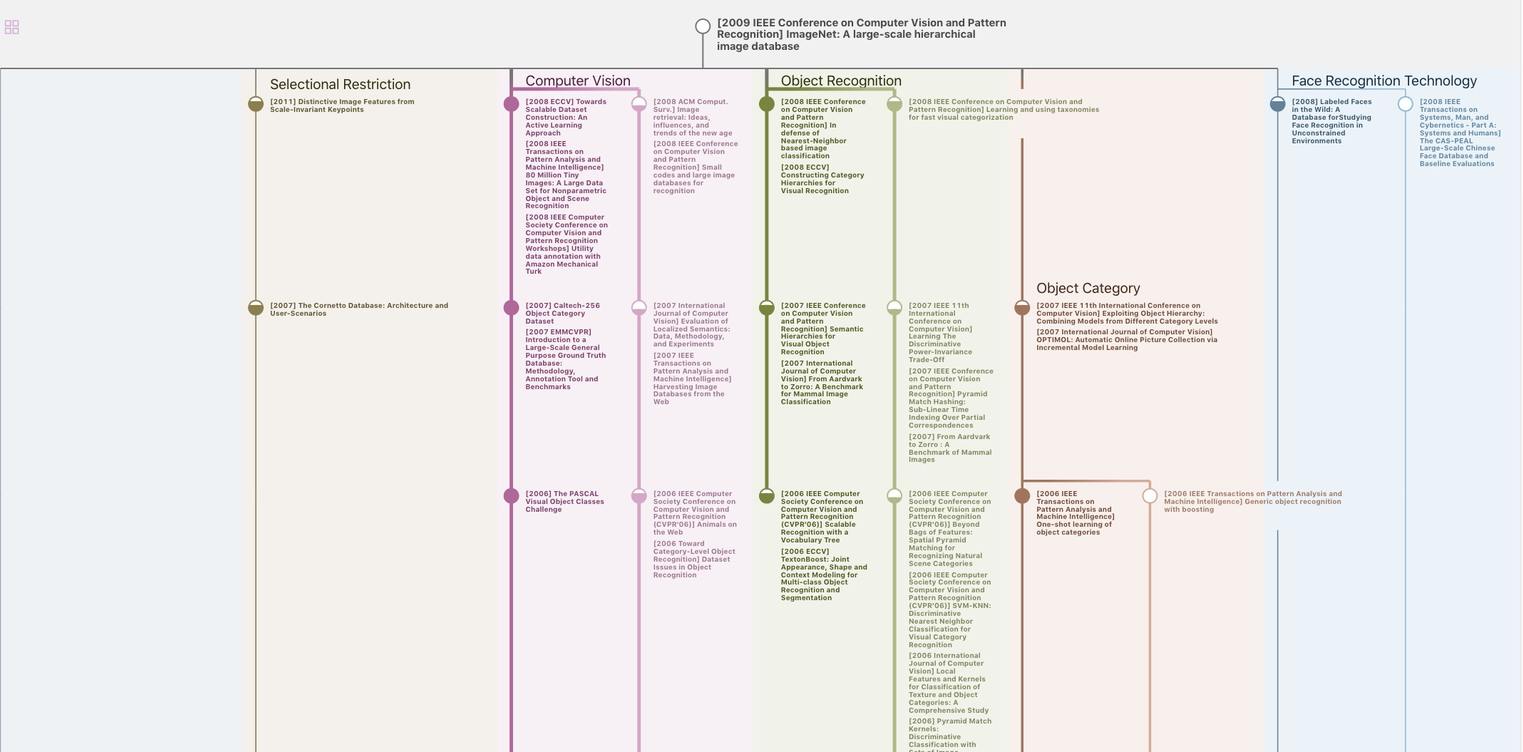
生成溯源树,研究论文发展脉络
Chat Paper
正在生成论文摘要